
🚀 Work With Us
Private Coaching
Language Editing
Qualitative Coding
✨ Free Resources
Templates & Tools
Short Courses
Articles & Videos

Research Topics & Ideas: Data Science
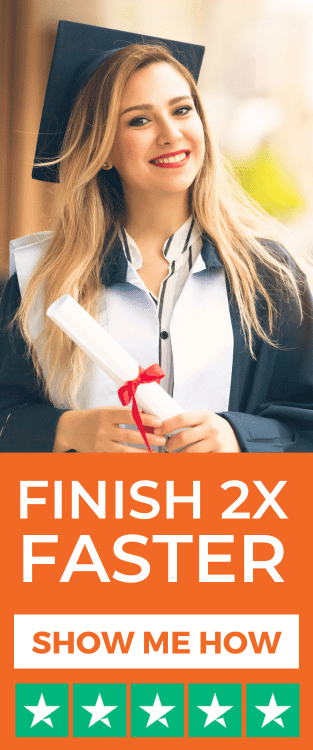
PS – This is just the start…
We know it’s exciting to run through a list of research topics, but please keep in mind that this list is just a starting point . These topic ideas provided here are intentionally broad and generic , so keep in mind that you will need to develop them further. Nevertheless, they should inspire some ideas for your project.
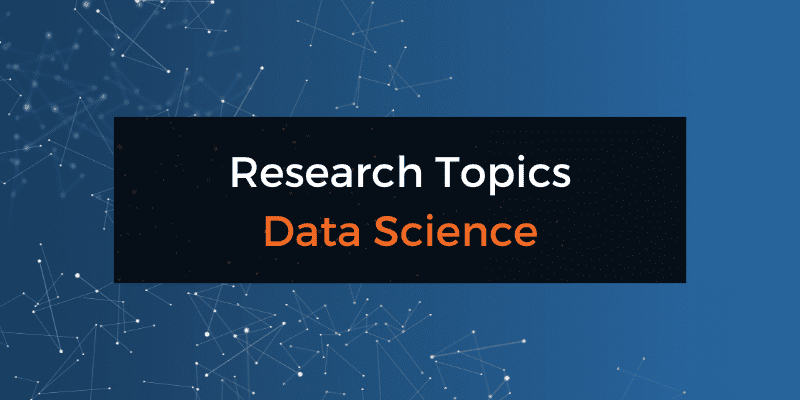
Data Science-Related Research Topics
- Developing machine learning models for real-time fraud detection in online transactions.
- The use of big data analytics in predicting and managing urban traffic flow.
- Investigating the effectiveness of data mining techniques in identifying early signs of mental health issues from social media usage.
- The application of predictive analytics in personalizing cancer treatment plans.
- Analyzing consumer behavior through big data to enhance retail marketing strategies.
- The role of data science in optimizing renewable energy generation from wind farms.
- Developing natural language processing algorithms for real-time news aggregation and summarization.
- The application of big data in monitoring and predicting epidemic outbreaks.
- Investigating the use of machine learning in automating credit scoring for microfinance.
- The role of data analytics in improving patient care in telemedicine.
- Developing AI-driven models for predictive maintenance in the manufacturing industry.
- The use of big data analytics in enhancing cybersecurity threat intelligence.
- Investigating the impact of sentiment analysis on brand reputation management.
- The application of data science in optimizing logistics and supply chain operations.
- Developing deep learning techniques for image recognition in medical diagnostics.
- The role of big data in analyzing climate change impacts on agricultural productivity.
- Investigating the use of data analytics in optimizing energy consumption in smart buildings.
- The application of machine learning in detecting plagiarism in academic works.
- Analyzing social media data for trends in political opinion and electoral predictions.
- The role of big data in enhancing sports performance analytics.
- Developing data-driven strategies for effective water resource management.
- The use of big data in improving customer experience in the banking sector.
- Investigating the application of data science in fraud detection in insurance claims.
- The role of predictive analytics in financial market risk assessment.
- Developing AI models for early detection of network vulnerabilities.
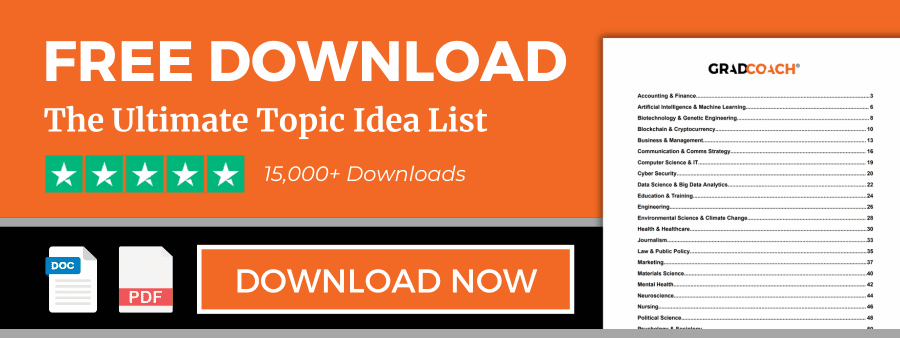
Data Science Research Ideas (Continued)
- The application of big data in public transportation systems for route optimization.
- Investigating the impact of big data analytics on e-commerce recommendation systems.
- The use of data mining techniques in understanding consumer preferences in the entertainment industry.
- Developing predictive models for real estate pricing and market trends.
- The role of big data in tracking and managing environmental pollution.
- Investigating the use of data analytics in improving airline operational efficiency.
- The application of machine learning in optimizing pharmaceutical drug discovery.
- Analyzing online customer reviews to inform product development in the tech industry.
- The role of data science in crime prediction and prevention strategies.
- Developing models for analyzing financial time series data for investment strategies.
- The use of big data in assessing the impact of educational policies on student performance.
- Investigating the effectiveness of data visualization techniques in business reporting.
- The application of data analytics in human resource management and talent acquisition.
- Developing algorithms for anomaly detection in network traffic data.
- The role of machine learning in enhancing personalized online learning experiences.
- Investigating the use of big data in urban planning and smart city development.
- The application of predictive analytics in weather forecasting and disaster management.
- Analyzing consumer data to drive innovations in the automotive industry.
- The role of data science in optimizing content delivery networks for streaming services.
- Developing machine learning models for automated text classification in legal documents.
- The use of big data in tracking global supply chain disruptions.
- Investigating the application of data analytics in personalized nutrition and fitness.
- The role of big data in enhancing the accuracy of geological surveying for natural resource exploration.
- Developing predictive models for customer churn in the telecommunications industry.
- The application of data science in optimizing advertisement placement and reach.
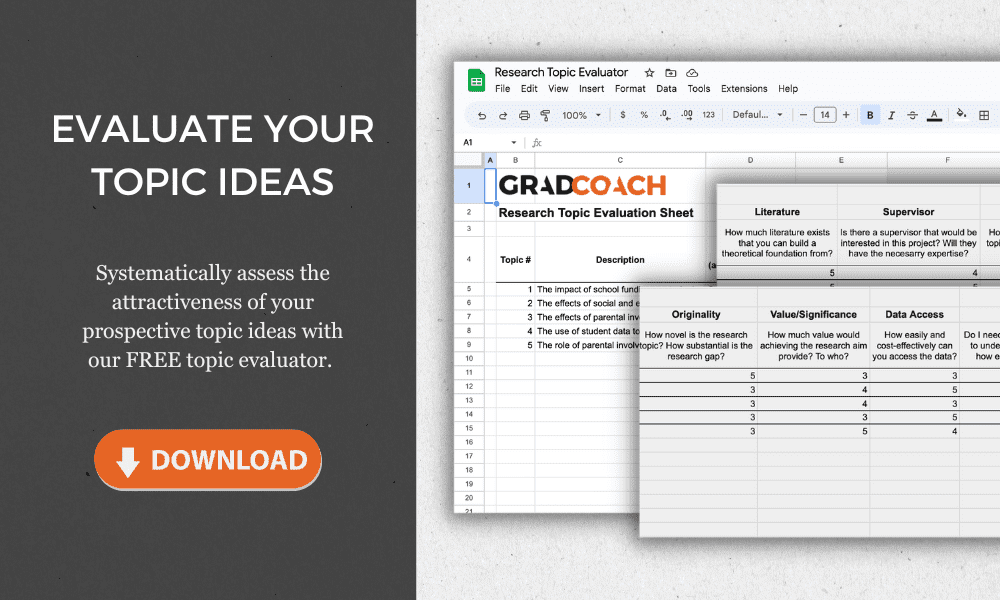
Recent Data Science-Related Studies
While the ideas we’ve presented above are a decent starting point for finding a research topic, they are fairly generic and non-specific. So, it helps to look at actual studies in the data science and analytics space to see how this all comes together in practice.
Below, we’ve included a selection of recent studies to help refine your thinking. These are actual studies, Â so they can provide some useful insight as to what a research topic looks like in practice.
- Data Science in Healthcare: COVID-19 and Beyond (Hulsen, 2022)
- Auto-ML Web-application for Automated Machine Learning Algorithm Training and evaluation (Mukherjee & Rao, 2022)
- Survey on Statistics and ML in Data Science and Effect in Businesses (Reddy et al., 2022)
- Visualization in Data Science VDS @ KDD 2022 (Plant et al., 2022)
- An Essay on How Data Science Can Strengthen Business (Santos, 2023)
- A Deep study of Data science related problems, application and machine learning algorithms utilized in Data science (Ranjani et al., 2022)
- You Teach WHAT in Your Data Science Course?!? (Posner & Kerby-Helm, 2022)
- Statistical Analysis for the Traffic Police Activity: Nashville, Tennessee, USA (Tufail & Gul, 2022)
- Data Management and Visual Information Processing in Financial Organization using Machine Learning (Balamurugan et al., 2022)
- A Proposal of an Interactive Web Application Tool QuickViz: To Automate Exploratory Data Analysis (Pitroda, 2022)
- Applications of Data Science in Respective Engineering Domains (Rasool & Chaudhary, 2022)
- Jupyter Notebooks for Introducing Data Science to Novice Users (Fruchart et al., 2022)
- Towards a Systematic Review of Data Science Programs: Themes, Courses, and Ethics (Nellore & Zimmer, 2022)
- Application of data science and bioinformatics in healthcare technologies (Veeranki & Varshney, 2022)
- TAPS Responsibility Matrix: A tool for responsible data science by design (Urovi et al., 2023)
- Data Detectives: A Data Science Program for Middle Grade Learners (Thompson & Irgens, 2022)
- MACHINE LEARNING FOR NON-MAJORS: A WHITE BOX APPROACH (Mike & Hazzan, 2022)
- COMPONENTS OF DATA SCIENCE AND ITS APPLICATIONS (Paul et al., 2022)
- Analysis on the Application of Data Science in Business Analytics (Wang, 2022)
As you can see, these research topics are a lot more focused than the generic topic ideas we presented earlier. So, for you to develop a high-quality research topic, you’ll need to get specific and laser-focused on a specific context with specific variables of interest. In the video below, we explore some other important things you’ll need to consider when crafting your research topic.
Get 1-On-1 Help
If you’re still unsure about how to find a quality research topic, check out our Private Coaching service, the perfect starting point for developing a unique, well-justified research topic.
Find The Perfect Research Topic
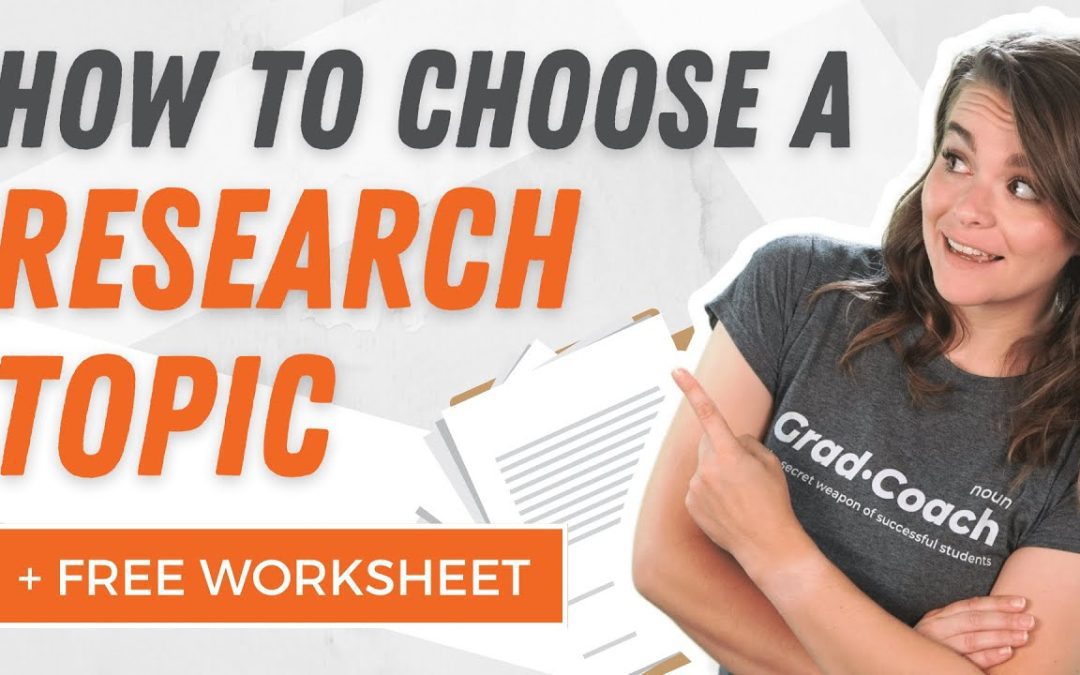
How To Choose A Research Topic: 5 Key Criteria
Learn how to systematically evaluate potential research topics and choose the best option for your dissertation, thesis or research paper.
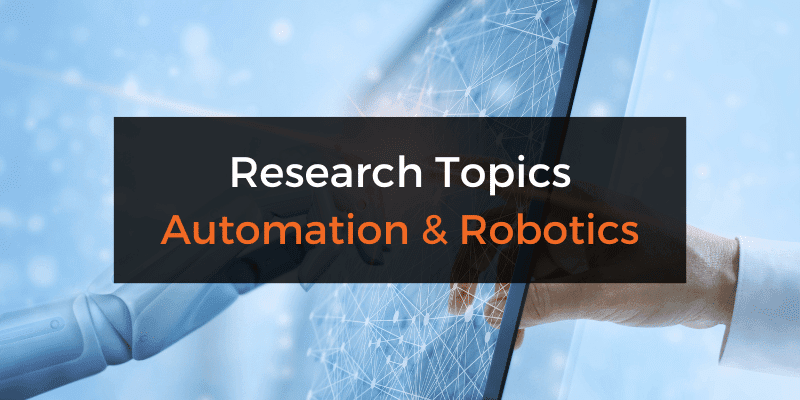
Research Topics & Ideas: Automation & Robotics
A comprehensive list of automation and robotics-related research topics. Includes free access to a webinar and research topic evaluator.
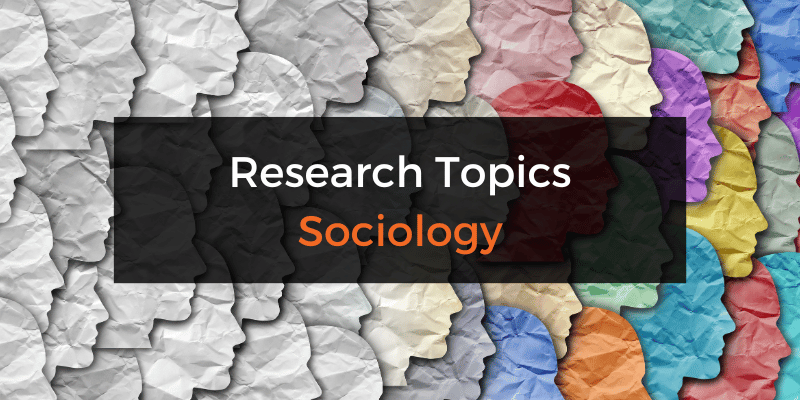
Research Topics & Ideas: Sociology
A comprehensive list of sociology-related research topics. Includes free access to a webinar and research topic evaluator.
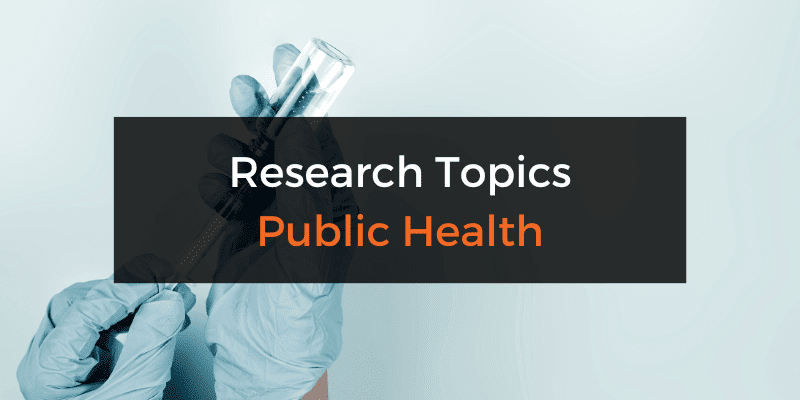
Research Topics & Ideas: Public Health & Epidemiology
A comprehensive list of public health-related research topics. Includes free access to a webinar and research topic evaluator.
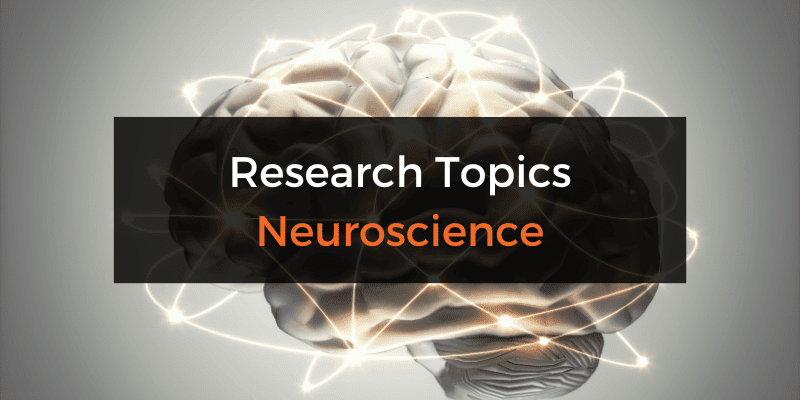
Research Topics & Ideas: Neuroscience
A comprehensive list of neuroscience-related research topics. Includes free access to a webinar and research topic evaluator.
đź“„ FREE TEMPLATES
Research Topic Ideation
Proposal Writing
Literature Review
Methodology & Analysis
Academic Writing
Referencing & Citing
Apps, Tools & Tricks
The Grad Coach Podcast
I have to submit dissertation. can I get any help
Submit a Comment Cancel reply
Your email address will not be published. Required fields are marked *
Save my name, email, and website in this browser for the next time I comment.
Submit Comment
- Print Friendly
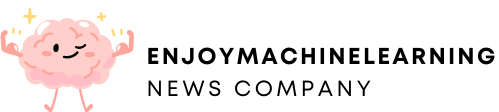
37 Research Topics In Data Science To Stay On Top Of
- February 22, 2024
As a data scientist, staying on top of the latest research in your field is essential.
The data science landscape changes rapidly, and new techniques and tools are constantly being developed.
To keep up with the competition, you need to be aware of the latest trends and topics in data science research.
In this article, we will provide an overview of 37 hot research topics in data science.
We will discuss each topic in detail, including its significance and potential applications.
These topics could be an idea for a thesis or simply topics you can research independently.
Stay tuned – this is one blog post you don’t want to miss!
37 Research Topics in Data Science
1.) predictive modeling.
Predictive modeling is a significant portion of data science and a topic you must be aware of.
Simply put, it is the process of using historical data to build models that can predict future outcomes.
Predictive modeling has many applications, from marketing and sales to financial forecasting and risk management.
As businesses increasingly rely on data to make decisions, predictive modeling is becoming more and more important.
While it can be complex, predictive modeling is a powerful tool that gives businesses a competitive advantage.
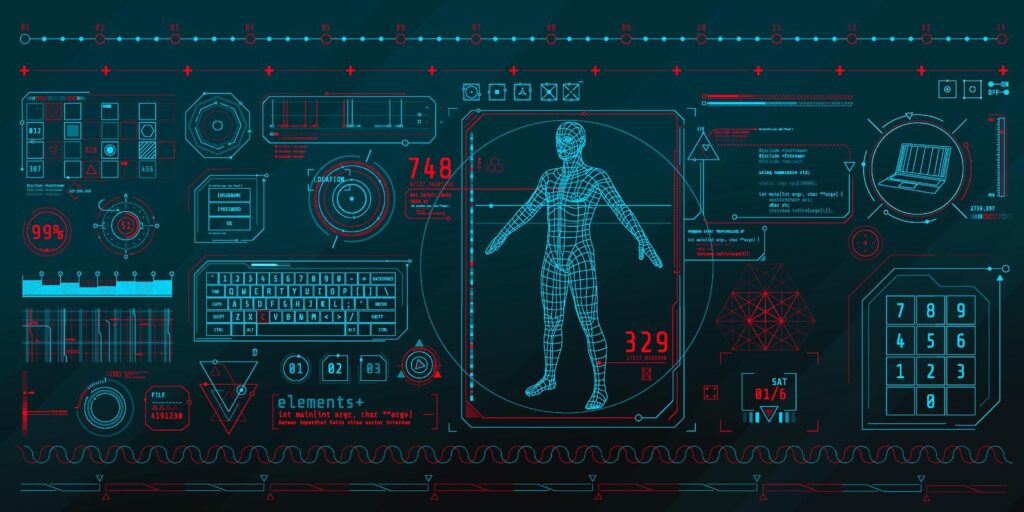
2.) Big Data Analytics
These days, it seems like everyone is talking about big data.
And with good reason – organizations of all sizes are sitting on mountains of data, and they’re increasingly turning to data scientists to help them make sense of it all.
But what exactly is big data? And what does it mean for data science?
Simply put, big data is a term used to describe datasets that are too large and complex for traditional data processing techniques.
Big data typically refers to datasets of a few terabytes or more.
But size isn’t the only defining characteristic – big data is also characterized by its high Velocity (the speed at which data is generated), Variety (the different types of data), and Volume (the amount of the information).
Given the enormity of big data, it’s not surprising that organizations are struggling to make sense of it all.
That’s where data science comes in.
Data scientists use various methods to wrangle big data, including distributed computing and other decentralized technologies.
With the help of data science, organizations are beginning to unlock the hidden value in their big data.
By harnessing the power of big data analytics, they can improve their decision-making, better understand their customers, and develop new products and services.
3.) Auto Machine Learning
Auto machine learning is a research topic in data science concerned with developing algorithms that can automatically learn from data without intervention.
This area of research is vital because it allows data scientists to automate the process of writing code for every dataset.
This allows us to focus on other tasks, such as model selection and validation.
Auto machine learning algorithms can learn from data in a hands-off way for the data scientist – while still providing incredible insights.
This makes them a valuable tool for data scientists who either don’t have the skills to do their own analysis or are struggling.
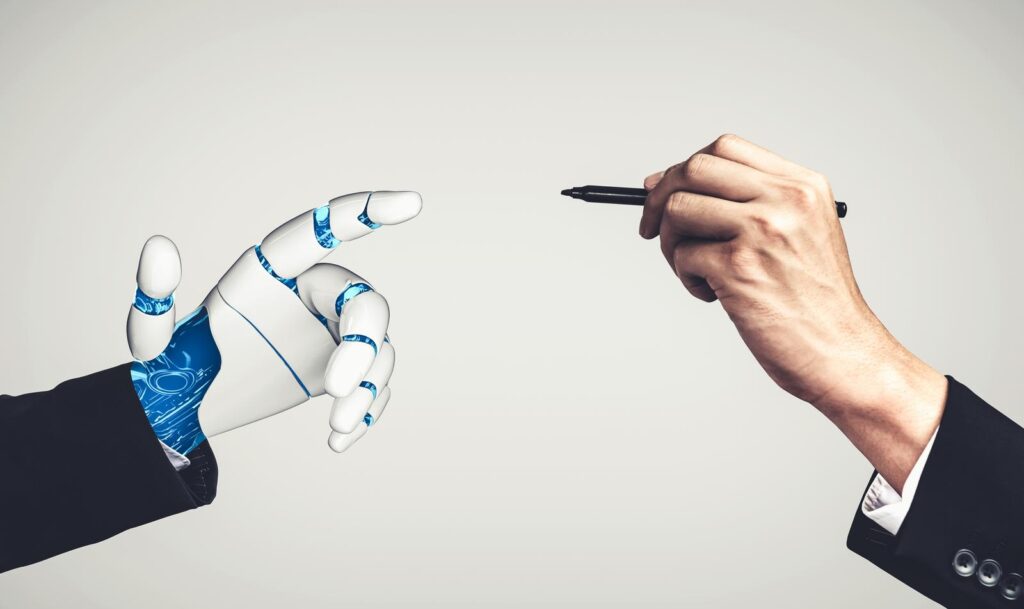
4.) Text Mining
Text mining is a research topic in data science that deals with text data extraction.
This area of research is important because it allows us to get as much information as possible from the vast amount of text data available today.
Text mining techniques can extract information from text data, such as keywords, sentiments, and relationships.
This information can be used for various purposes, such as model building and predictive analytics.
5.) Natural Language Processing
Natural language processing is a data science research topic that analyzes human language data.
This area of research is important because it allows us to understand and make sense of the vast amount of text data available today.
Natural language processing techniques can build predictive and interactive models from any language data.
Natural Language processing is pretty broad, and recent advances like GPT-3 have pushed this topic to the forefront.
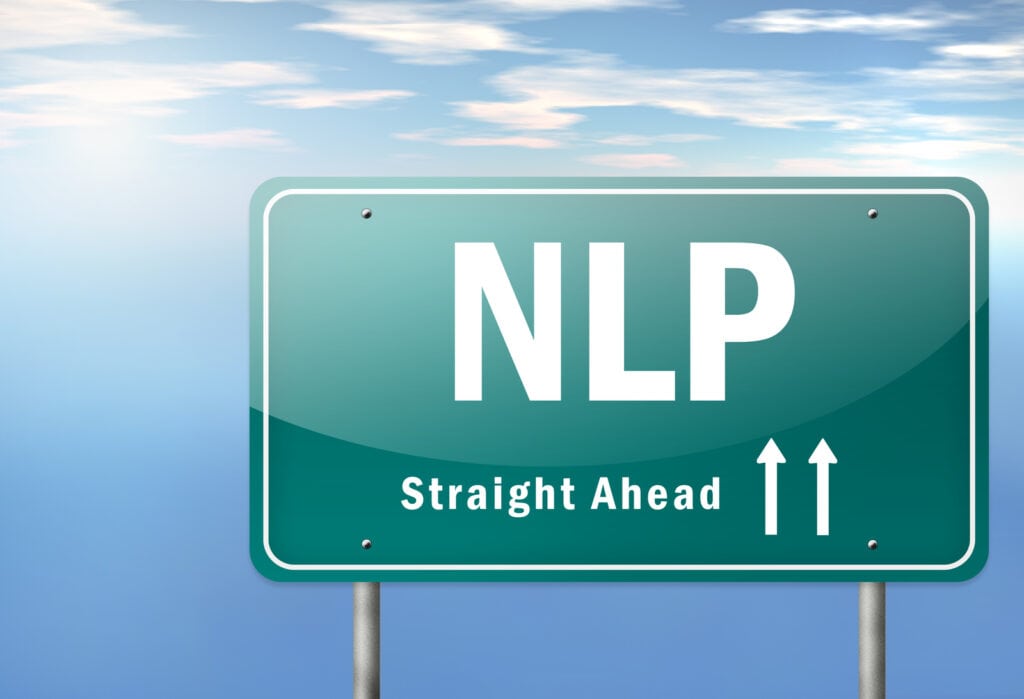
6.) Recommender Systems
Recommender systems are an exciting topic in data science because they allow us to make better products, services, and content recommendations.
Businesses can better understand their customers and their needs by using recommender systems.
This, in turn, allows them to develop better products and services that meet the needs of their customers.
Recommender systems are also used to recommend content to users.
This can be done on an individual level or at a group level.
Think about Netflix, for example, always knowing what you want to watch!
Recommender systems are a valuable tool for businesses and users alike.
7.) Deep Learning
Deep learning is a research topic in data science that deals with artificial neural networks.
These networks are composed of multiple layers, and each layer is formed from various nodes.
Deep learning networks can learn from data similarly to how humans learn, irrespective of the data distribution.
This makes them a valuable tool for data scientists looking to build models that can learn from data independently.
The deep learning network has become very popular in recent years because of its ability to achieve state-of-the-art results on various tasks.
There seems to be a new SOTA deep learning algorithm research paper on https://arxiv.org/  every single day!
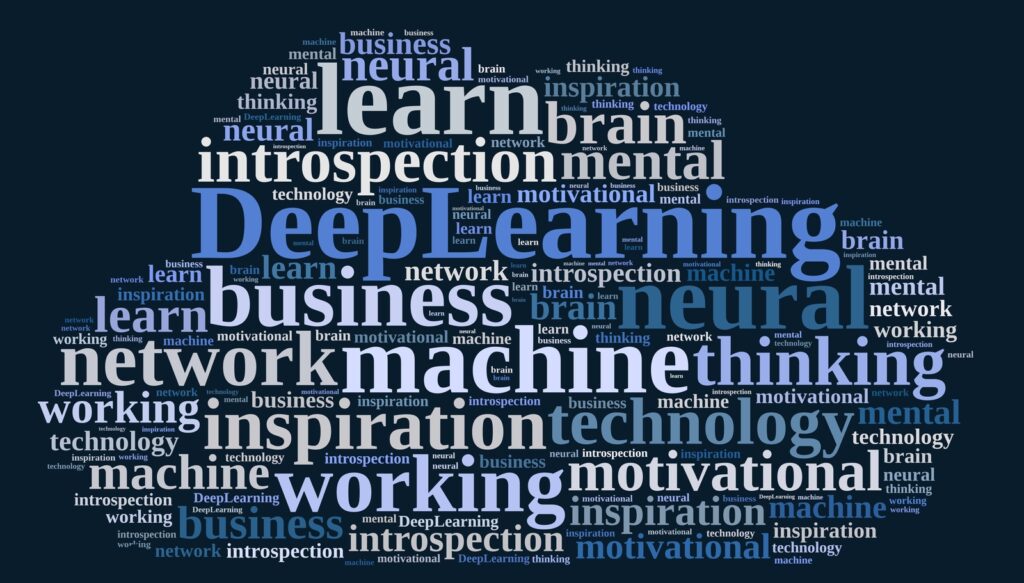
8.) Reinforcement Learning
Reinforcement learning is a research topic in data science that deals with algorithms that can learn on multiple levels from interactions with their environment.
This area of research is essential because it allows us to develop algorithms that can learn non-greedy approaches to decision-making, allowing businesses and companies to win in the long term compared to the short.
9.) Data Visualization
Data visualization is an excellent research topic in data science because it allows us to see our data in a way that is easy to understand.
Data visualization techniques can be used to create charts, graphs, and other visual representations of data.
This allows us to see the patterns and trends hidden in our data.
Data visualization is also used to communicate results to others.
This allows us to share our findings with others in a way that is easy to understand.
There are many ways to contribute to and learn about data visualization.
Some ways include attending conferences, reading papers, and contributing to open-source projects.
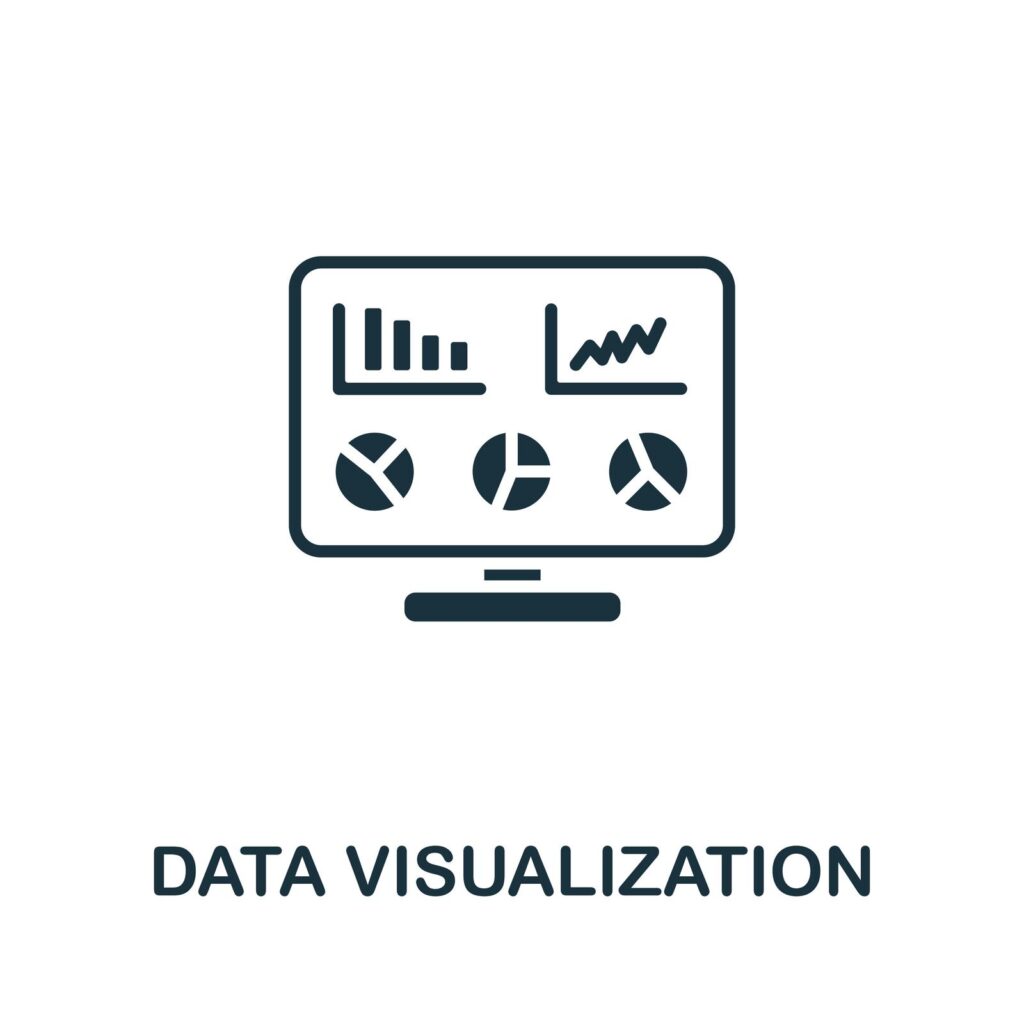
10.) Predictive Maintenance
Predictive maintenance is a hot topic in data science because it allows us to prevent failures before they happen.
This is done using data analytics to predict when a failure will occur.
This allows us to take corrective action before the failure actually happens.
While this sounds simple, avoiding false positives while keeping recall is challenging and an area wide open for advancement.
11.) Financial Analysis
Financial analysis is an older topic that has been around for a while but is still a great field where contributions can be felt.
Current researchers are focused on analyzing macroeconomic data to make better financial decisions.
This is done by analyzing the data to identify trends and patterns.
Financial analysts can use this information to make informed decisions about where to invest their money.
Financial analysis is also used to predict future economic trends.
This allows businesses and individuals to prepare for potential financial hardships and enable companies to be cash-heavy during good economic conditions.
Overall, financial analysis is a valuable tool for anyone looking to make better financial decisions.
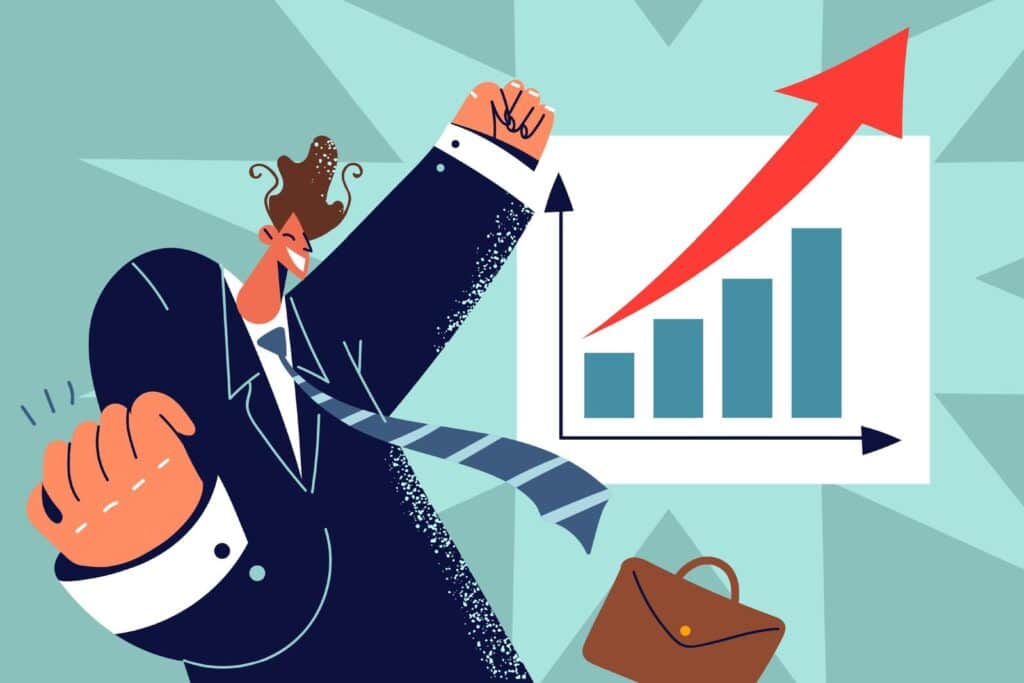
12.) Image Recognition
Image recognition is one of the hottest topics in data science because it allows us to identify objects in images.
This is done using artificial intelligence algorithms that can learn from data and understand what objects you’re looking for.
This allows us to build models that can accurately recognize objects in images and video.
This is a valuable tool for businesses and individuals who want to be able to identify objects in images.
Think about security, identification, routing, traffic, etc.
Image Recognition has gained a ton of momentum recently – for a good reason.
13.) Fraud Detection
Fraud detection is a great topic in data science because it allows us to identify fraudulent activity before it happens.
This is done by analyzing data to look for patterns and trends that may be associated with the fraud.
Once our machine learning model recognizes some of these patterns in real time, it immediately detects fraud.
This allows us to take corrective action before the fraud actually happens.
Fraud detection is a valuable tool for anyone who wants to protect themselves from potential fraudulent activity.
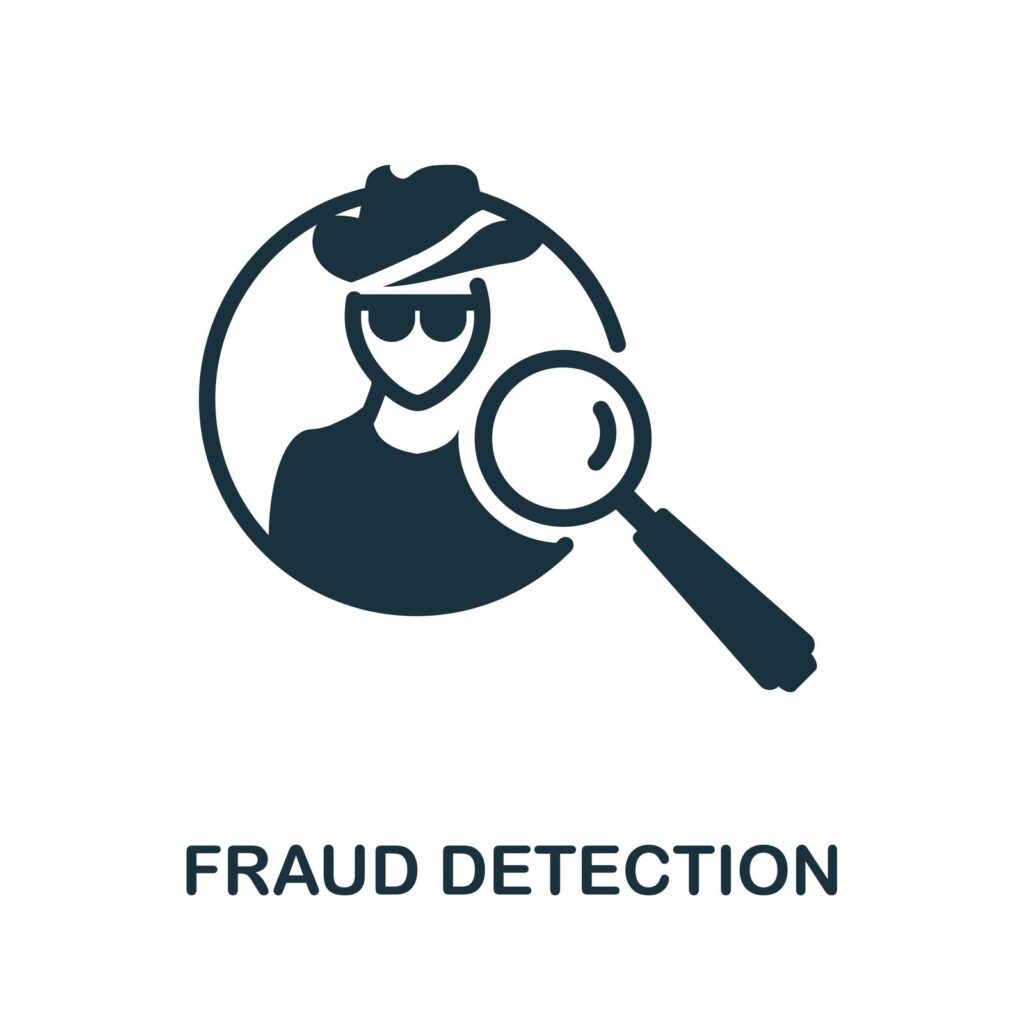
14.) Web Scraping
Web scraping is a controversial topic in data science because it allows us to collect data from the web, which is usually data you do not own.
This is done by extracting data from websites using scraping tools that are usually custom-programmed.
This allows us to collect data that would otherwise be inaccessible.
For obvious reasons, web scraping is a unique tool – giving you data your competitors would have no chance of getting.
I think there is an excellent opportunity to create new and innovative ways to make scraping accessible for everyone, not just those who understand Selenium and Beautiful Soup.
15.) Social Media Analysis
Social media analysis is not new; many people have already created exciting and innovative algorithms to study this.
However, it is still a great data science research topic because it allows us to understand how people interact on social media.
This is done by analyzing data from social media platforms to look for insights, bots, and recent societal trends.
Once we understand these practices, we can use this information to improve our marketing efforts.
For example, if we know that a particular demographic prefers a specific type of content, we can create more content that appeals to them.
Social media analysis is also used to understand how people interact with brands on social media.
This allows businesses to understand better what their customers want and need.
Overall, social media analysis is valuable for anyone who wants to improve their marketing efforts or understand how customers interact with brands.
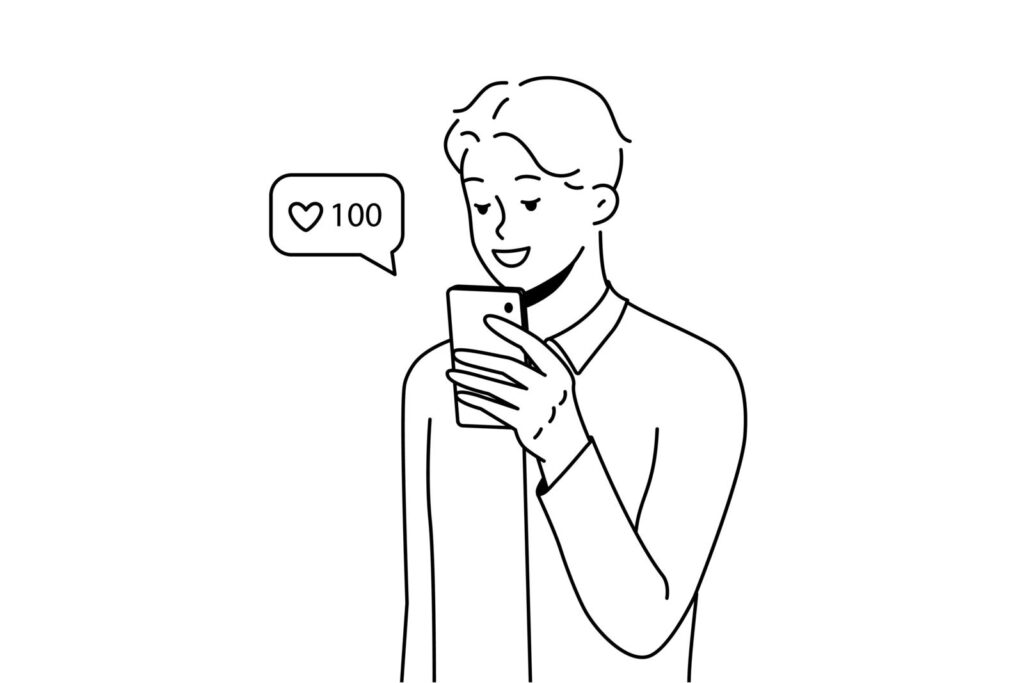
16.) GPU Computing
GPU computing is a fun new research topic in data science because it allows us to process data much faster than traditional CPUs .
Due to how GPUs are made, they’re incredibly proficient at intense matrix operations, outperforming traditional CPUs by very high margins.
While the computation is fast, the coding is still tricky.
There is an excellent research opportunity to bring these innovations to non-traditional modules, allowing data science to take advantage of GPU computing outside of deep learning.
17.) Quantum Computing
Quantum computing is a new research topic in data science and physics because it allows us to process data much faster than traditional computers.
It also opens the door to new types of data.
There are just some problems that can’t be solved utilizing outside of the classical computer.
For example, if you wanted to understand how a single atom moved around, a classical computer couldn’t handle this problem.
You’ll need to utilize a quantum computer to handle quantum mechanics problems.
This may be the “hottest” research topic on the planet right now, with some of the top researchers in computer science and physics worldwide working on it.
You could be too.
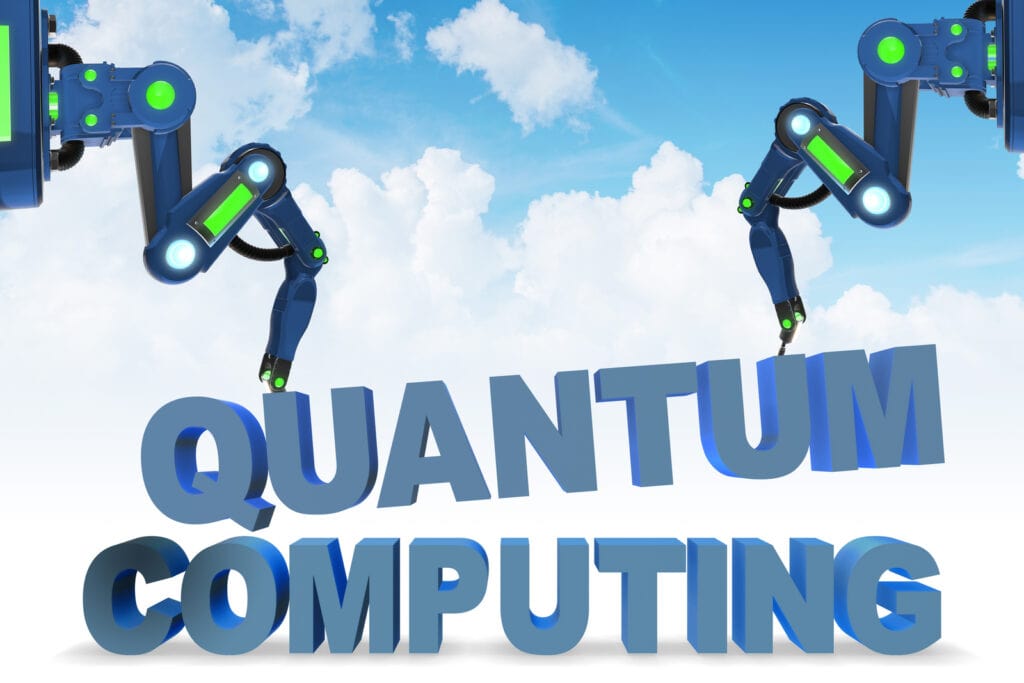
18.) Genomics
Genomics may be the only research topic that can compete with quantum computing regarding the “number of top researchers working on it.”
Genomics is a fantastic intersection of data science because it allows us to understand how genes work.
This is done by sequencing the DNA of different organisms to look for insights into our and other species.
Once we understand these patterns, we can use this information to improve our understanding of diseases and create new and innovative treatments for them.
Genomics is also used to study the evolution of different species.
Genomics is the future and a field begging for new and exciting research professionals to take it to the next step.
19.) Location-based services
Location-based services are an old and time-tested research topic in data science.
Since GPS and 4g cell phone reception became a thing, we’ve been trying to stay informed about how humans interact with their environment.
This is done by analyzing data from GPS tracking devices, cell phone towers, and Wi-Fi routers to look for insights into how humans interact.
Once we understand these practices, we can use this information to improve our geotargeting efforts, improve maps, find faster routes, and improve cohesion throughout a community.
Location-based services are used to understand the user, something every business could always use a little bit more of.
While a seemingly “stale” field, location-based services have seen a revival period with self-driving cars.
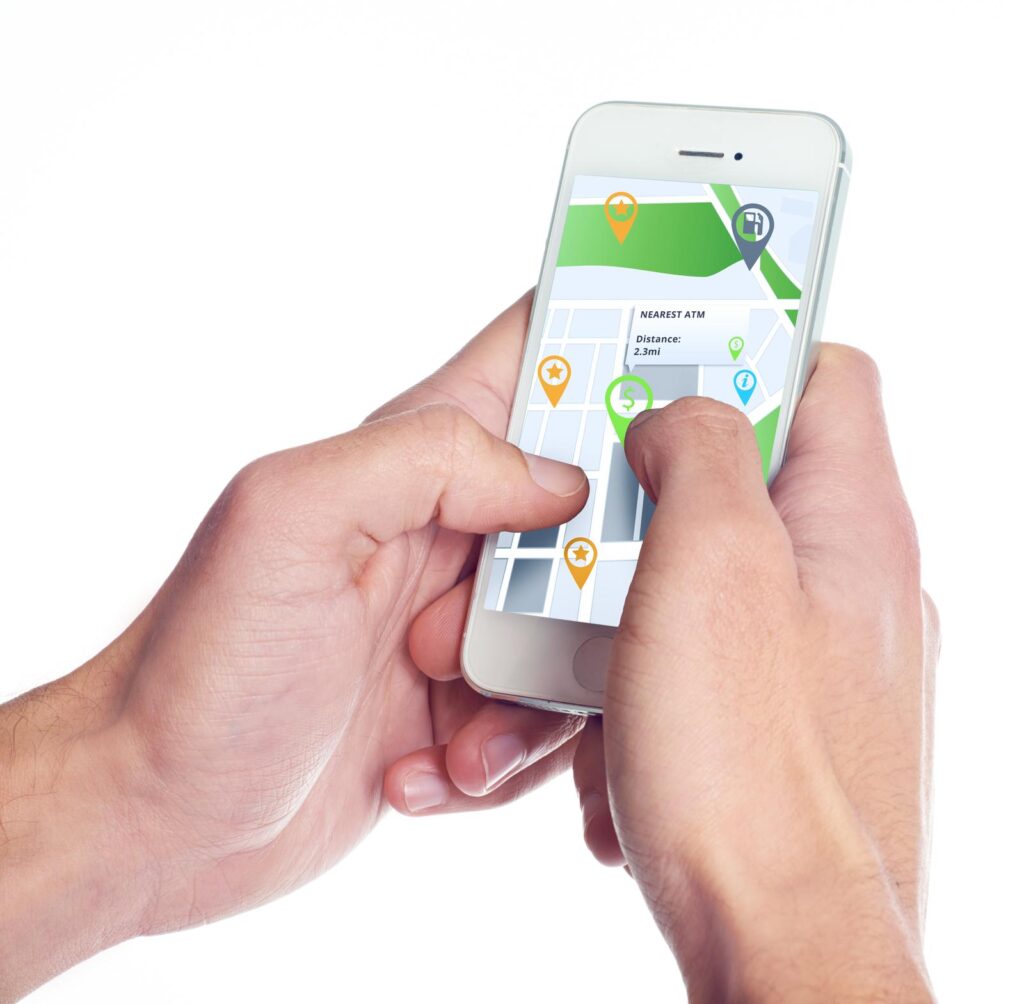
20.) Smart City Applications
Smart city applications are all the rage in data science research right now.
By harnessing the power of data, cities can become more efficient and sustainable.
But what exactly are smart city applications?
In short, they are systems that use data to improve city infrastructure and services.
This can include anything from traffic management and energy use to waste management and public safety.
Data is collected from various sources, including sensors, cameras, and social media.
It is then analyzed to identify tendencies and habits.
This information can make predictions about future needs and optimize city resources.
As more and more cities strive to become “smart,” the demand for data scientists with expertise in smart city applications is only growing.
21.) Internet Of Things (IoT)
The Internet of Things, or IoT, is exciting and new data science and sustainability research topic.
IoT is a network of physical objects embedded with sensors and connected to the internet.
These objects can include everything from alarm clocks to refrigerators; they’re all connected to the internet.
That means that they can share data with computers.
And that’s where data science comes in.
Data scientists are using IoT data to learn everything from how people use energy to how traffic flows through a city.
They’re also using IoT data to predict when an appliance will break down or when a road will be congested.
Really, the possibilities are endless.
With such a wide-open field, it’s easy to see why IoT is being researched by some of the top professionals in the world.
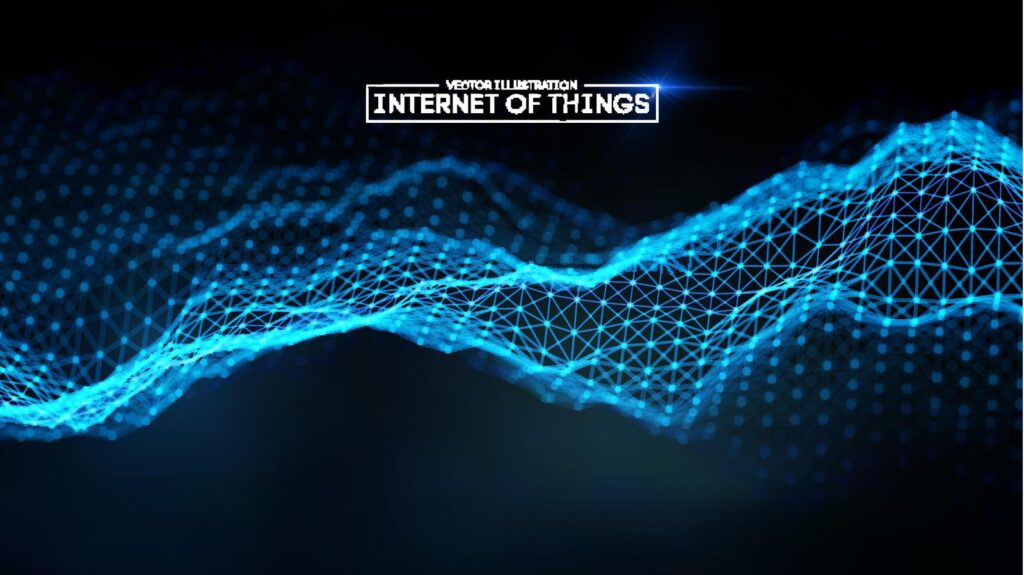
22.) Cybersecurity
Cybersecurity is a relatively new research topic in data science and in general, but it’s already garnering a lot of attention from businesses and organizations.
After all, with the increasing number of cyber attacks in recent years, it’s clear that we need to find better ways to protect our data.
While most of cybersecurity focuses on infrastructure, data scientists can leverage historical events to find potential exploits to protect their companies.
Sometimes, looking at a problem from a different angle helps, and that’s what data science brings to cybersecurity.
Also, data science can help to develop new security technologies and protocols.
As a result, cybersecurity is a crucial data science research area and one that will only become more important in the years to come.
23.) Blockchain
Blockchain is an incredible new research topic in data science for several reasons.
First, it is a distributed database technology that enables secure, transparent, and tamper-proof transactions.
Did someone say transmitting data?
This makes it an ideal platform for tracking data and transactions in various industries.
Second, blockchain is powered by cryptography, which not only makes it highly secure – but is a familiar foe for data scientists.
Finally, blockchain is still in its early stages of development, so there is much room for research and innovation.
As a result, blockchain is a great new research topic in data science that vows to revolutionize how we store, transmit and manage data.
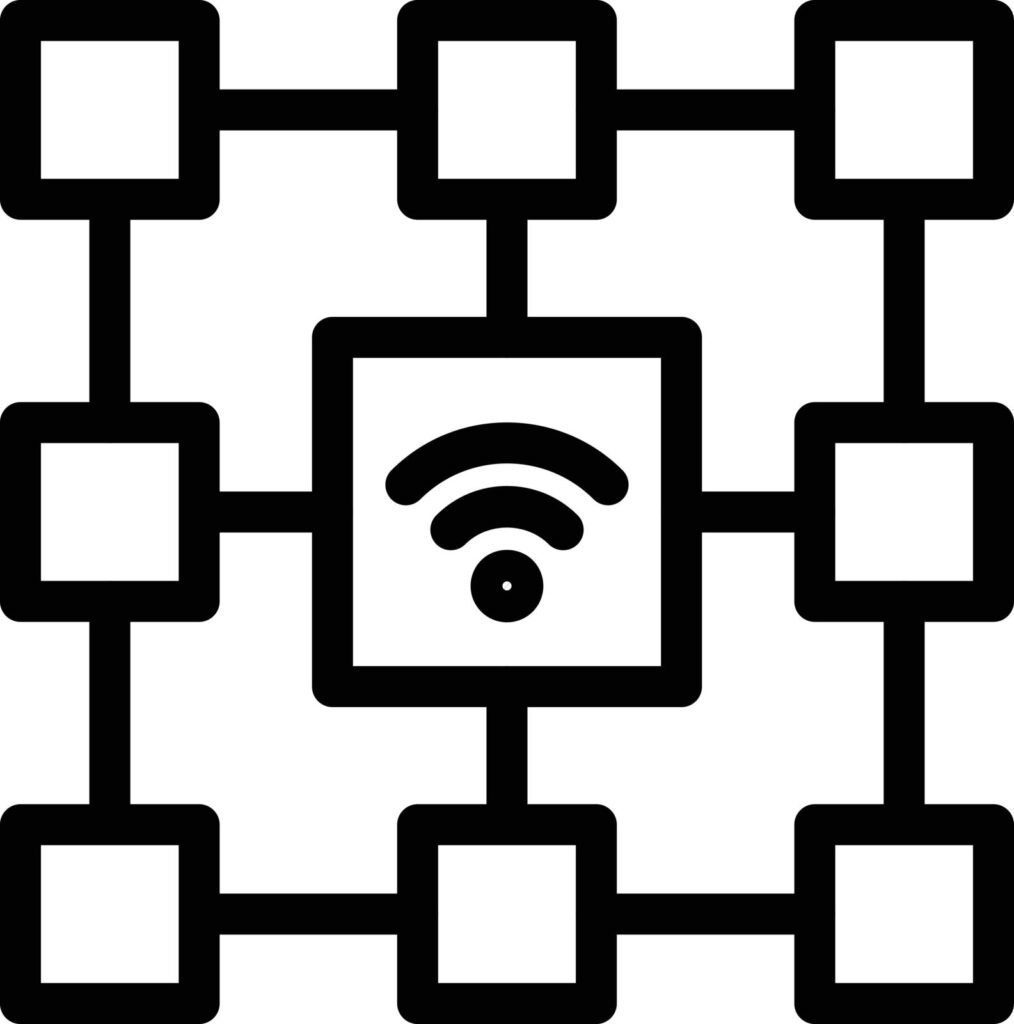
24.) Sustainability
Sustainability is a relatively new research topic in data science, but it is gaining traction quickly.
To keep up with this demand, The Wharton School of the University of Pennsylvania has started to offer an MBA in Sustainability .
This demand isn’t shocking, and some of the reasons include the following:
Sustainability is an important issue that is relevant to everyone.
Datasets on sustainability are constantly growing and changing, making it an exciting challenge for data scientists.
There hasn’t been a “set way” to approach sustainability from a data perspective, making it an excellent opportunity for interdisciplinary research.
As data science grows, sustainability will likely become an increasingly important research topic.
25.) Educational Data
Education has always been a great topic for research, and with the advent of big data, educational data has become an even richer source of information.
By studying educational data, researchers can gain insights into how students learn, what motivates them, and what barriers these students may face.
Besides, data science can be used to develop educational interventions tailored to individual students’ needs.
Imagine being the researcher that helps that high schooler pass mathematics; what an incredible feeling.
With the increasing availability of educational data, data science has enormous potential to improve the quality of education.
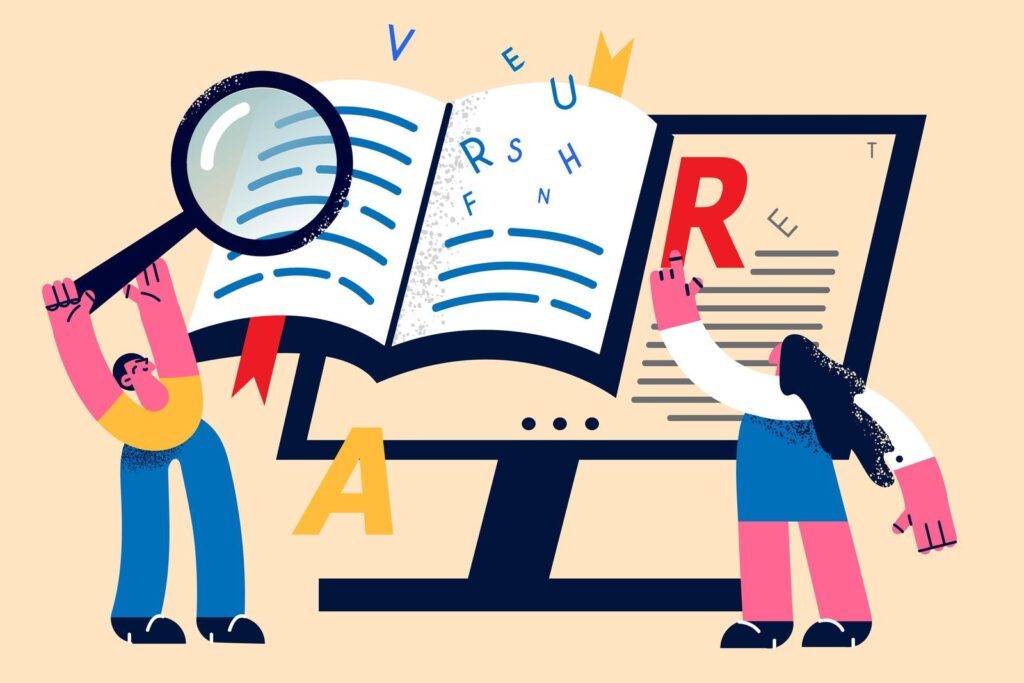
26.) Politics
As data science continues to evolve, so does the scope of its applications.
Originally used primarily for business intelligence and marketing, data science is now applied to various fields, including politics.
By analyzing large data sets, political scientists (data scientists with a cooler name) can gain valuable insights into voting patterns, campaign strategies, and more.
Further, data science can be used to forecast election results and understand the effects of political events on public opinion.
With the wealth of data available, there is no shortage of research opportunities in this field.
As data science evolves, so does our understanding of politics and its role in our world.
27.) Cloud Technologies
Cloud technologies are a great research topic.
It allows for the outsourcing and sharing of computer resources and applications all over the internet.
This lets organizations save money on hardware and maintenance costs while providing employees access to the latest and greatest software and applications.
I believe there is an argument that AWS could be the greatest and most technologically advanced business ever built (Yes, I know it’s only part of the company).
Besides, cloud technologies can help improve team members’ collaboration by allowing them to share files and work on projects together in real-time.
As more businesses adopt cloud technologies, data scientists must stay up-to-date on the latest trends in this area.
By researching cloud technologies, data scientists can help organizations to make the most of this new and exciting technology.
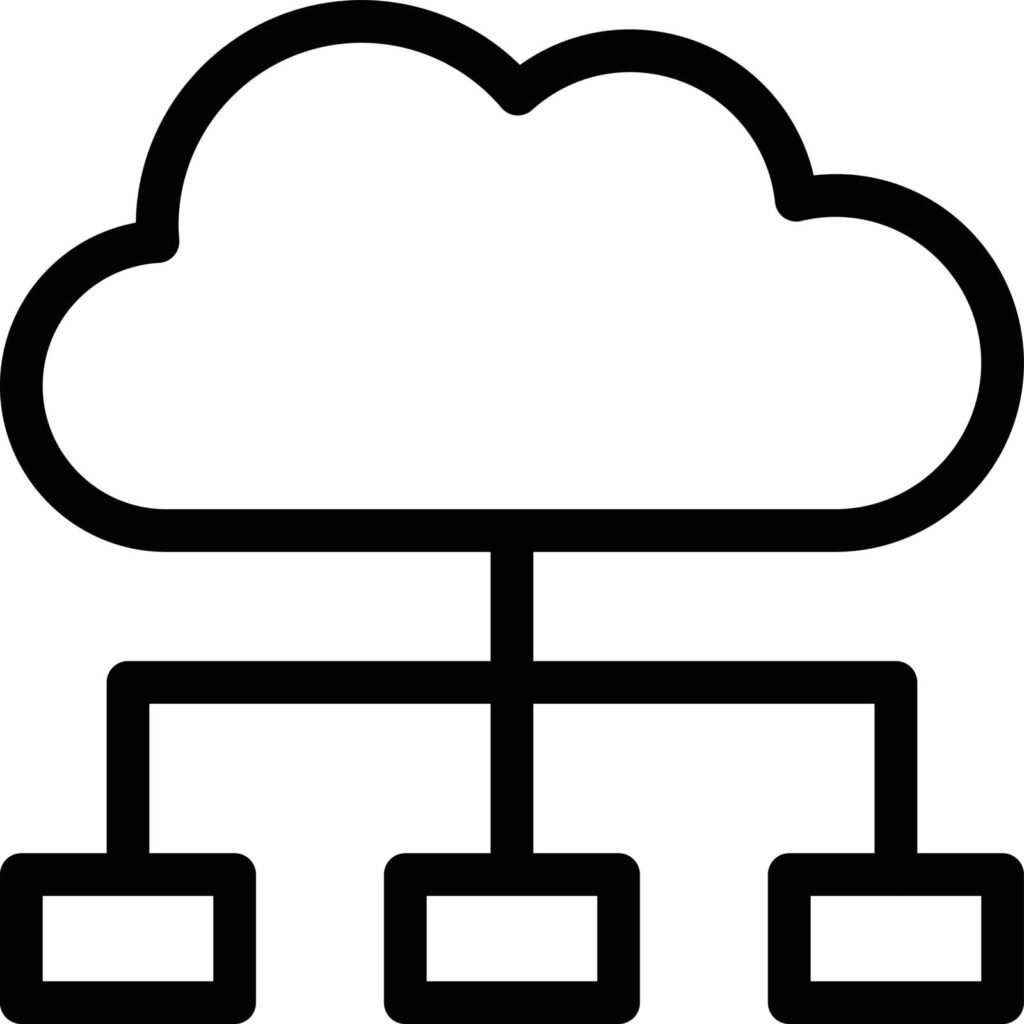
28.) Robotics
Robotics has recently become a household name, and it’s for a good reason.
First, robotics deals with controlling and planning physical systems, an inherently complex problem.
Second, robotics requires various sensors and actuators to interact with the world, making it an ideal application for machine learning techniques.
Finally, robotics is an interdisciplinary field that draws on various disciplines, such as computer science, mechanical engineering, and electrical engineering.
As a result, robotics is a rich source of research problems for data scientists.
29.) HealthCare
Healthcare is an industry that is ripe for data-driven innovation.
Hospitals, clinics, and health insurance companies generate a tremendous amount of data daily.
This data can be used to improve the quality of care and outcomes for patients.
This is perfect timing, as the healthcare industry is undergoing a significant shift towards value-based care, which means there is a greater need than ever for data-driven decision-making.
As a result, healthcare is an exciting new research topic for data scientists.
There are many different ways in which data can be used to improve healthcare, and there is a ton of room for newcomers to make discoveries.
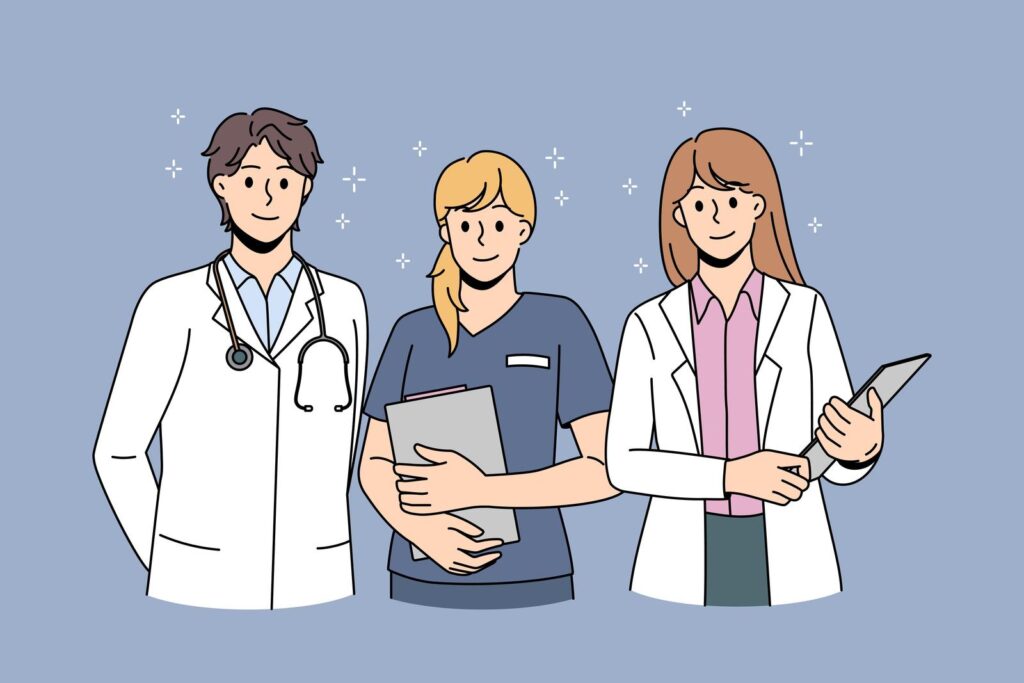
30.) Remote Work
There’s no doubt that remote work is on the rise.
In today’s global economy, more and more businesses are allowing their employees to work from home or anywhere else they can get a stable internet connection.
But what does this mean for data science? Well, for one thing, it opens up a whole new field of research.
For example, how does remote work impact employee productivity?
What are the best ways to manage and collaborate on data science projects when team members are spread across the globe?
And what are the cybersecurity risks associated with working remotely?
These are just a few of the questions that data scientists will be able to answer with further research.
So if you’re looking for a new topic to sink your teeth into, remote work in data science is a great option.
31.) Data-Driven Journalism
Data-driven journalism is an exciting new field of research that combines the best of both worlds: the rigor of data science with the creativity of journalism.
By applying data analytics to large datasets, journalists can uncover stories that would otherwise be hidden.
And telling these stories compellingly can help people better understand the world around them.
Data-driven journalism is still in its infancy, but it has already had a major impact on how news is reported.
In the future, it will only become more important as data becomes increasingly fluid among journalists.
It is an exciting new topic and research field for data scientists to explore.
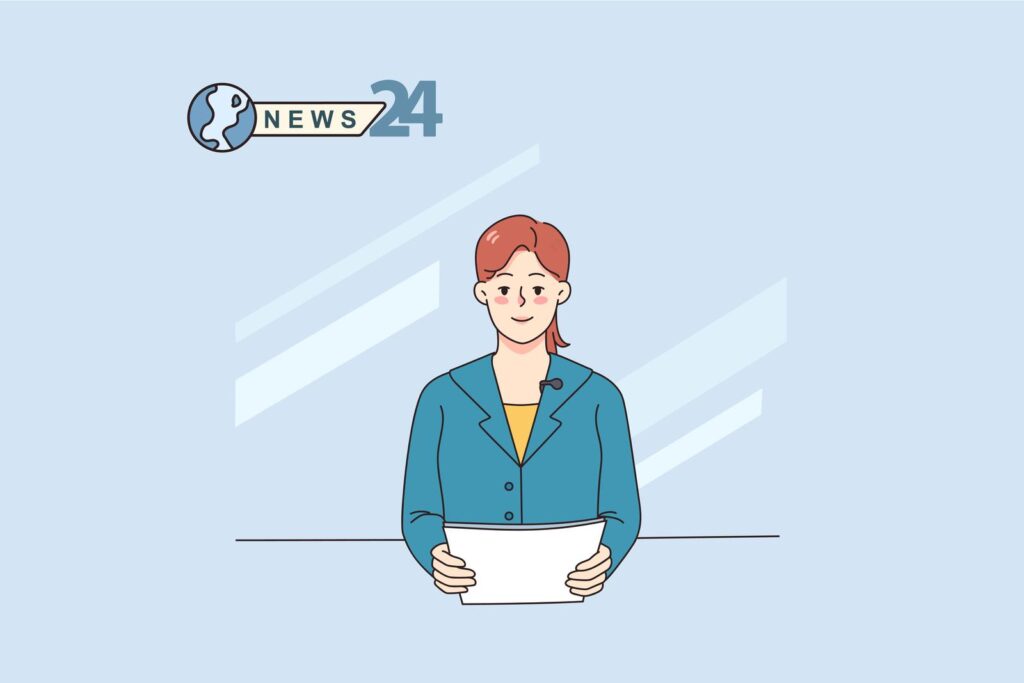
32.) Data Engineering
Data engineering is a staple in data science, focusing on efficiently managing data.
Data engineers are responsible for developing and maintaining the systems that collect, process, and store data.
In recent years, there has been an increasing demand for data engineers as the volume of data generated by businesses and organizations has grown exponentially.
Data engineers must be able to design and implement efficient data-processing pipelines and have the skills to optimize and troubleshoot existing systems.
If you are looking for a challenging research topic that would immediately impact you worldwide, then improving or innovating a new approach in data engineering would be a good start.
33.) Data Curation
Data curation has been a hot topic in the data science community for some time now.
Curating data involves organizing, managing, and preserving data so researchers can use it.
Data curation can help to ensure that data is accurate, reliable, and accessible.
It can also help to prevent research duplication and to facilitate the sharing of data between researchers.
Data curation is a vital part of data science. In recent years, there has been an increasing focus on data curation, as it has become clear that it is essential for ensuring data quality.
As a result, data curation is now a major research topic in data science.
There are numerous books and articles on the subject, and many universities offer courses on data curation.
Data curation is an integral part of data science and will only become more important in the future.
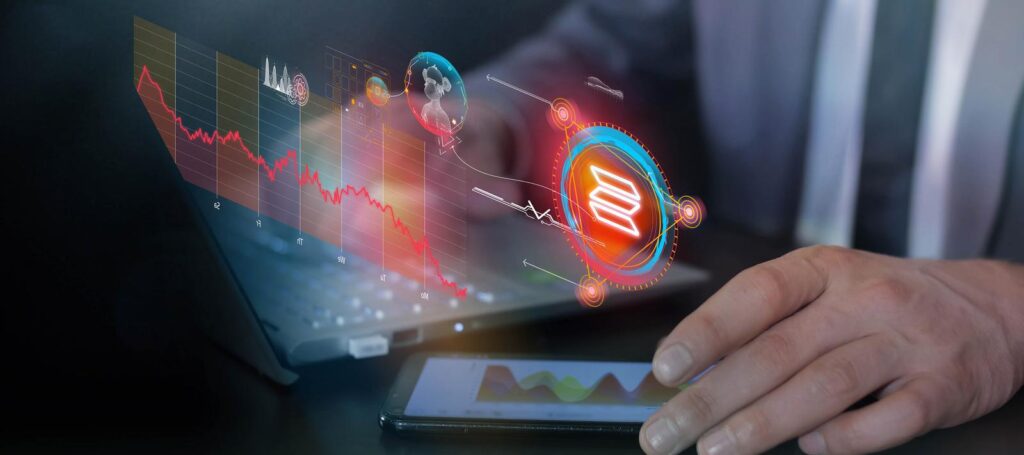
34.) Meta-Learning
Meta-learning is gaining a ton of steam in data science. It’s learning how to learn.
So, if you can learn how to learn, you can learn anything much faster.
Meta-learning is mainly used in deep learning, as applications outside of this are generally pretty hard.
In deep learning, many parameters need to be tuned for a good model, and there’s usually a lot of data.
You can save time and effort if you can automatically and quickly do this tuning.
In machine learning, meta-learning can improve models’ performance by sharing knowledge between different models.
For example, if you have a bunch of different models that all solve the same problem, then you can use meta-learning to share the knowledge between them to improve the cluster (groups) overall performance.
I don’t know how anyone looking for a research topic could stay away from this field; it’s what the Terminator  warned us about!
35.) Data Warehousing
A data warehouse is a system used for data analysis and reporting.
It is a central data repository created by combining data from multiple sources.
Data warehouses are often used to store historical data, such as sales data, financial data, and customer data.
This data type can be used to create reports and perform statistical analysis.
Data warehouses also store data that the organization is not currently using.
This type of data can be used for future research projects.
Data warehousing is an incredible research topic in data science because it offers a variety of benefits.
Data warehouses help organizations to save time and money by reducing the need for manual data entry.
They also help to improve the accuracy of reports and provide a complete picture of the organization’s performance.
Data warehousing feels like one of the weakest parts of the Data Science Technology Stack; if you want a research topic that could have a monumental impact – data warehousing is an excellent place to look.
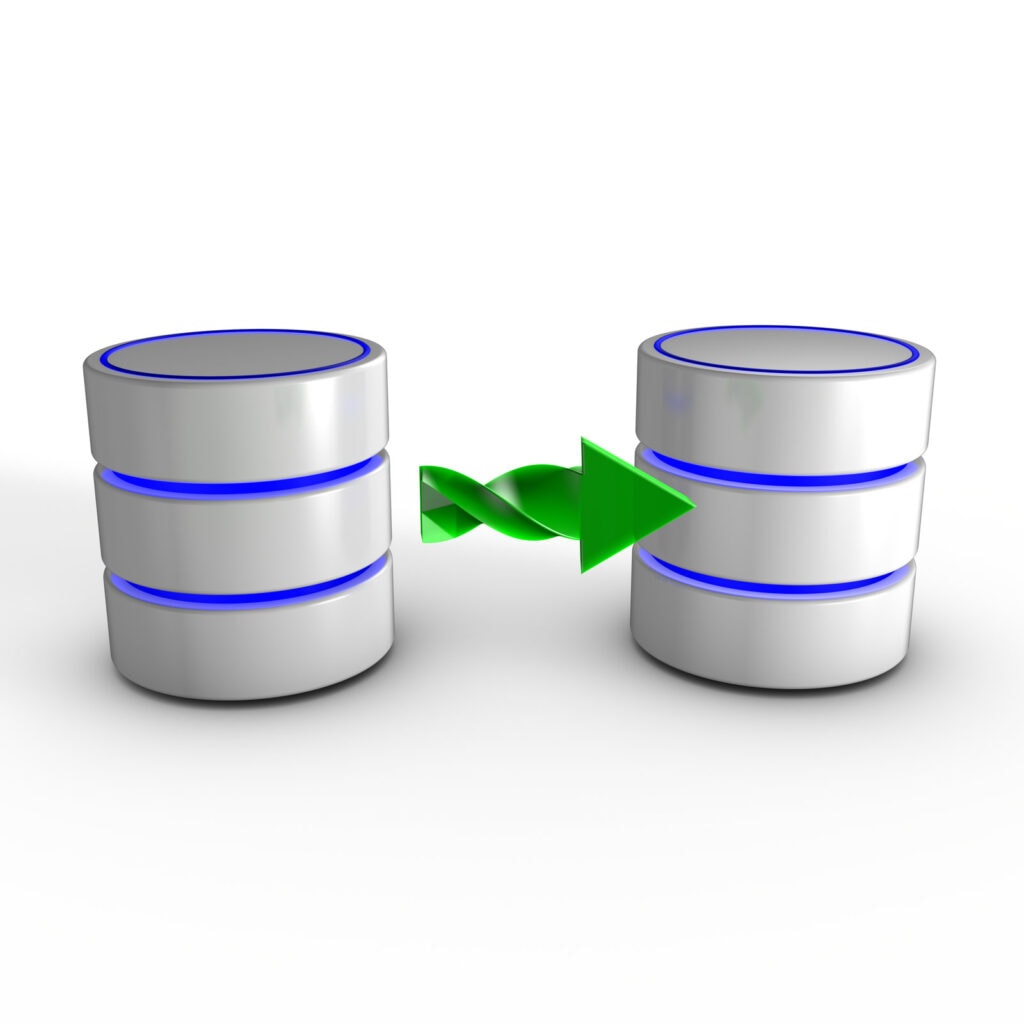
36.) Business Intelligence
Business intelligence aims to collect, process, and analyze data to help businesses make better decisions.
Business intelligence can improve marketing, sales, customer service, and operations.
It can also be used to identify new business opportunities and track competition.
BI is business and another tool in your company’s toolbox to continue dominating your area.
Data science is the perfect tool for business intelligence because it combines statistics, computer science, and machine learning.
Data scientists can use business intelligence to answer questions like, “What are our customers buying?” or “What are our competitors doing?” or “How can we increase sales?”
Business intelligence is a great way to improve your business’s bottom line and an excellent opportunity to dive deep into a well-respected research topic.
37.) Crowdsourcing
One of the newest areas of research in data science is crowdsourcing.
Crowdsourcing is a process of sourcing tasks or projects to a large group of people, typically via the internet.
This can be done for various purposes, such as gathering data, developing new algorithms, or even just for fun (think: online quizzes and surveys).
But what makes crowdsourcing so powerful is that it allows businesses and organizations to tap into a vast pool of talent and resources they wouldn’t otherwise have access to.
And with the rise of social media, it’s easier than ever to connect with potential crowdsource workers worldwide.
Imagine if you could effect that, finding innovative ways to improve how people work together.
That would have a huge effect.
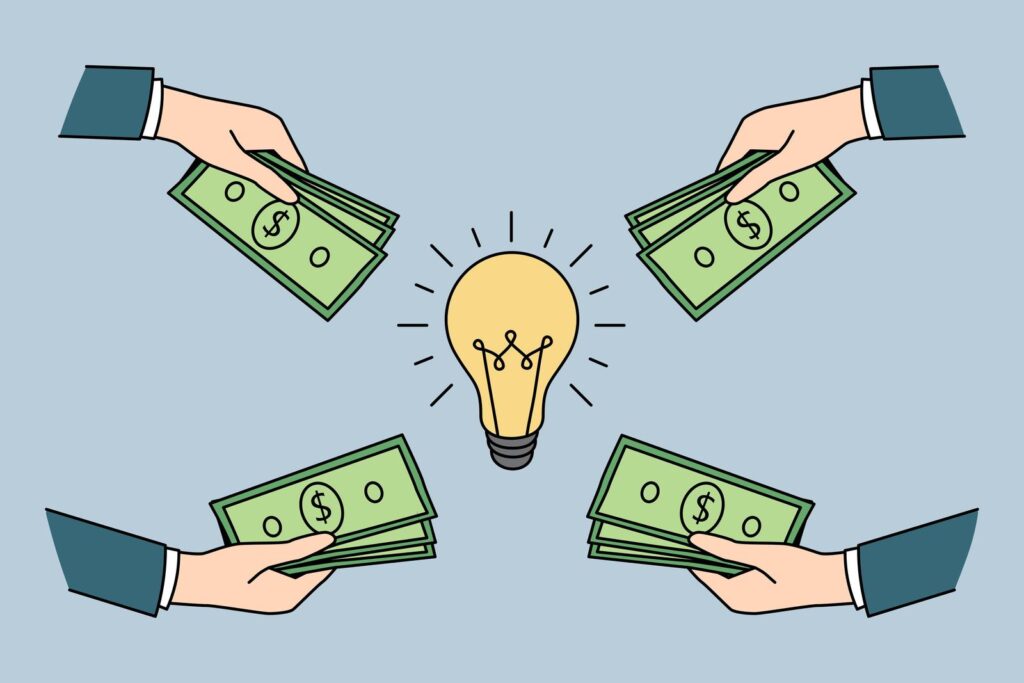
Final Thoughts, Are These Research Topics In Data Science For You?
Thirty-seven different research topics in data science are a lot to take in, but we hope you found a research topic that interests you.
If not, don’t worry – there are plenty of other great topics to explore.
The important thing is to get started with your research and find ways to apply what you learn to real-world problems.
We wish you the best of luck as you begin your data science journey!
Other Data Science Articles
We love talking about data science; here are a couple of our favorite articles:
- Why Are You Interested In Data Science?
- Recent Posts
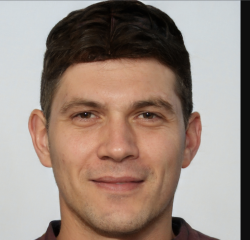
- How to use ProKitchen software [Unlock Your Full Potential] - December 8, 2024
- Do I Need to Install Software to Use Microsoft Teams? [Discover the Easy Answer] - December 7, 2024
- Does Netflix Hire Entry-Level Software Engineers? Find Out How to Land Your Dream Job [Secret Insider Tips] - December 7, 2024
- For Individuals
- For Businesses
- For Universities
- For Governments
- Online Degrees
- Join for Free
Getting a PhD in Data Science: What You Need to Know
A PhD in data science prepares you for some of the most cutting-edge research in the field and can advance your career. But, whether you should pursue one depends on your own personal goals and resources. Learn more inside.
![data science phd topics [Featured Image]: A candidate for a PhD degree in Data Science, is sitting at her desk, working on her laptop computer.](https://d3njjcbhbojbot.cloudfront.net/api/utilities/v1/imageproxy/https://images.ctfassets.net/wp1lcwdav1p1/5V6y6oXGWPmYkZs8M96ogf/b46e5d8ac45e46cd7e94fb3661aeb875/Stocksy_txp233b02ffaSu200_Medium_3441517.jpg?w=1500&h=680&q=60&fit=fill&f=faces&fm=jpg&fl=progressive&auto=format%2Ccompress&dpr=1&w=1000)
A Doctor of Philosophy (PhD) is the highest degree that a professional can obtain in the field of data science. Focused primarily on equipping degree holders with the skills and knowledge required to conduct original research, a PhD prepares degree holders for advanced professional positions in both industry and academia.Â
But, the path to obtaining a PhD is filled with many years of potentially costly study that can be discouraging to those looking for rapid career progression. Before jumping into a doctoral program, then, it’s important to define what your goals are and how a PhD may (or may not) fit into them.Â
In this article, you’ll learn more about PhDs in data science, the different factors you should consider before joining one, and types of programs to consider. At the end, you’ll also find some suggested online courses to help you get started today.Â
PhD in Data Science: OverviewÂ
A Doctor of Philosophy (PhD) is the terminal degree in the field of data science, meaning it is the highest possible degree that can be obtained in the subject. Holding a PhD in data science, consequently, signals your mastery and knowledge of the field to both potential employers and fellow professionals.Â
At a glance, here’s what you should know about a Data Science PhD:Â
PhD vs. Master’s Degree in Data Science
There are two graduate degrees in the field of data science: a master’s in Data science and a PhD in Data Science. While both of these degrees can have a beneficial impact on your job prospects, they also have key differences that might impact which one is better for you.Â
A Master’s in Data Science is a graduate degree between a bachelor’s and PhD, which usually takes between one and two years to complete. A master’s degree expands on what was learned in undergraduate school through more advanced courses in topics such as machine learning, data analytics, and statistics. Often, a master’s student in data science also pursues original research and completes a capstone project, which highlights what they learned in their program.
A PhD in Data Science is a research degree that typically takes four to five years to complete but can take longer depending on a range of personal factors. In addition to taking more advanced courses, PhD candidates devote a significant amount of time to teaching and conducting dissertation research with the intent of advancing the field. At the conclusion of their doctoral program, a PhD holder in Data Science will complete a dissertation representing a significant contribution to the field.Â
Typically, bachelor’s degree holders entering a PhD program are able to earn their master’s degree as a part of their doctoral program. Those entering a master’s program, however, will usually have to apply for a PhD program even if it’s in the same department.Â
Skills and curriculumÂ
Every PhD program is unique with its own requirements and focus. Nonetheless, they do have similar features, such as course, credit, and teaching requirements. To help you get a better understanding of how a doctoral graduate program in data science might be, here’s an example curriculum from NYU [ 1 ]:Â
Complete 72 credit hours while maintaining a cumulative grade point average of 3.0 (out of 4.0) each semester.
Core courses in topics like probability, statistics, machine learning, big data, inference, and research.Â
39 credit hours for elective courses in such topics as deep learning, natural language processing, and computational cognitive modeling.Â
Complete teaching requirements.
Pass a comprehensive exam.Â
Pass the Depth Qualifying Exam (DQE) by May 15 of their fourth semester.Â
Complete all steps for approval of their PhD dissertation.Â
Is a PhD in Data Science worth it?Â
A PhD can open doors to new career opportunities and boost your employment prospects. But, it can also take a lot of time and money to complete. Everyone’s personal and professional goals are different, so consider these things when deciding if you should pursue a PhD in Data Science: Â
Cost and time
The amount of time and money it takes to complete a PhD are perhaps the most concrete considerations one makes when deciding whether or not they should pursue a doctoral degree. According to research conducted by Education Data Initiative, the average cost of a doctorate degree is $114,300 and takes roughly four to eight years to complete [ 2 ].Â
The exact amount of time and money you might spend obtaining your doctoral degree will depend on your own circumstances and program. Before applying for a doctoral degree, make sure to review each program’s graduation requirements and costs, so you have a clear understanding of what you’re getting into.Â
Data Science PhD salaryÂ
While there are no official statistics on the salary gains data scientist earn by getting a PhD, the median salary for all data scientists is much higher than the national average in the United States. According to the U.S. Bureau of Labor Statistics (BLS), for example, the median salary for data scientists was $100,910 as of May 2021 [ 3 ].Â
Typically, the entry-level degree to get a data science position is a bachelor’s degree, meaning that even just an undergraduate degree could help you land a job that earns a higher than average salary. Nonetheless, a PhD will likely prepare you for more advanced positions that could offer higher pay than less specialized roles.Â
Data Science PhD programsÂ
There are several types of doctoral programs that you might consider if you would like to obtain a PhD in data science. These include:Â

PhD in data science online
An online PhD program may appeal to individuals who are interested in a more flexible program that allows them to complete their coursework at their own pace. Often, online programs can also be cheaper than their in-person counterparts, though they often offer less opportunities for networking and mentorship. If you’re an independent, self-starter looking for a program that can fit into their already busy life, then you might consider an online PhD program.Â
PhD in data science in-person
An in-person PhD program is a more traditional, educational method in which you attend classes on campus with your peers and instructors. In addition to providing doctoral-level instruction, you will also have more opportunities to network and gain more personalized instruction than you will likely encounter through online programs. In-person programs tend to be more expensive and inflexible than in-person ones.
If you prefer real-world instruction, networking opportunities, and a more rigid structure, then you might consider an in-person doctoral program.Â
AlternativesÂ
As an alternative to a PhD program, you might also consider obtaining a master’s degree. While covering some of the same material as a doctoral program, a master’s usually takes much less time and money to complete.
If you’re motivated primarily by the desire to boost your chances of landing a job and gaining financial stability, then a master’s degree program might better help you achieve your goals.
Learn more about data scienceÂ
Whatever your educational goals, data science requires extensive knowledge and training to enter the profession. To prepare for your next career move, then, you might consider taking a flexible online course through Coursera.Â
The University of Colorado Boulder’s Data Science Foundations: Data Structures and Algorithms Specialization teaches course takers how to design algorithms, create applications, and organize, store, and process data efficiently. Their online Master of Science in Data Science , meanwhile, teaches broadly applicable foundational skills alongside specialized competencies tailored to specific career paths in just two years of instruction.Â
Article sources
NYU Center for Data Science. “ PhD in Data Science, Curriculum , https://cds.nyu.edu/phd-curriculum-info/.” Accessed September 27, 2022.Â
Education Data Initiative. “ Average Cost of a Doctorate Degree , https://educationdata.org/average-cost-of-a-doctorate-degree.” Accessed September 27, 2022.Â
US BLS. “ Occupational Outlook Handbook: Data Scientists , https://www.bls.gov/ooh/math/data-scientists.htm#tab-1.” Accessed September 27, 2022.Â
Keep reading
Coursera staff.
Editorial Team
Coursera’s editorial team is comprised of highly experienced professional editors, writers, and fact...
This content has been made available for informational purposes only. Learners are advised to conduct additional research to ensure that courses and other credentials pursued meet their personal, professional, and financial goals.
DiscoverDataScience.org
PhD in Data Science – Your Guide to Choosing a Doctorate Degree Program
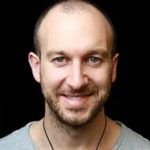
Created by aasif.faizal
Professional opportunities in data science are growing incredibly fast. That’s great news for students looking to pursue a career as a data scientist. But it also means that there are a lot more options out there to investigate and understand before developing the best educational path for you.
A PhD is the most advanced data science degree you can get, reflecting a depth of knowledge and technical expertise that will put you at the top of your field.
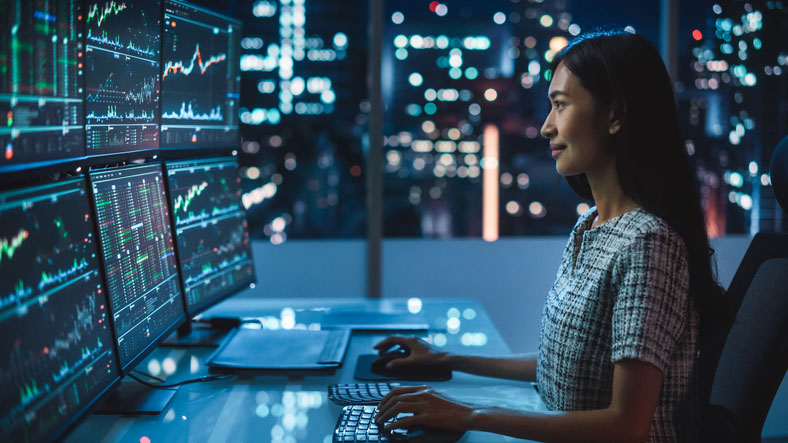
This means that PhD programs are the most time-intensive degree option out there, typically requiring that students complete dissertations involving rigorous research. This means that PhDs are not for everyone. Indeed, many who work in the world of big data hold master’s degrees rather than PhDs, which tend to involve the same coursework as PhD programs without a dissertation component. However, for the right candidate, a PhD program is the perfect choice to become a true expert on your area of focus.
If you’ve concluded that a data science PhD is the right path for you, this guide is intended to help you choose the best program to suit your needs. It will walk through some of the key considerations while picking graduate data science programs and some of the nuts and bolts (like course load and tuition costs) that are part of the data science PhD decision-making process.
Data Science PhD vs. Masters: Choosing the right option for you
If you’re considering pursuing a data science PhD, it’s worth knowing that such an advanced degree isn’t strictly necessary in order to get good work opportunities. Many who work in the field of big data only hold master’s degrees, which is the level of education expected to be a competitive candidate for data science positions.
So why pursue a data science PhD?
Simply put, a PhD in data science will leave you qualified to enter the big data industry at a high level from the outset.
You’ll be eligible for advanced positions within companies, holding greater responsibilities, keeping more direct communication with leadership, and having more influence on important data-driven decisions. You’re also likely to receive greater compensation to match your rank.
However, PhDs are not for everyone. Dissertations require a great deal of time and an interest in intensive research. If you are eager to jumpstart a career quickly, a master’s program will give you the preparation you need to hit the ground running. PhDs are appropriate for those who want to commit their time and effort to schooling as a long-term investment in their professional trajectory.
For more information on the difference between data science PhD’s and master’s programs, take a look at our guide here.
Topics include:
- Can I get an Online Ph.D in Data Science?
- Overview of Ph.d Coursework
Preparing for a Doctorate Program
Building a solid track record of professional experience, things to consider when choosing a school.
- What Does it Cost to Get a Ph.D in Data Science?
- School Listings
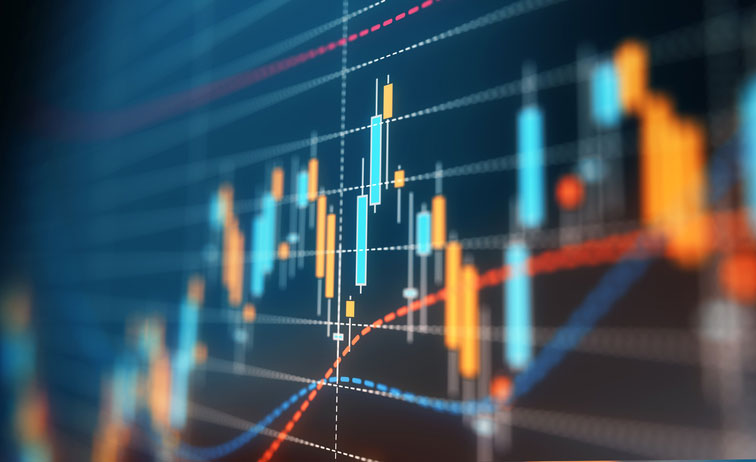
Data Science PhD Programs, Historically
Historically, data science PhD programs were one of the main avenues to get a good data-related position in academia or industry. But, PhD programs are heavily research oriented and require a somewhat long term investment of time, money, and energy to obtain. The issue that some data science PhD holders are reporting, especially in industry settings, is that that the state of the art is moving so quickly, and that the data science industry is evolving so rapidly, that an abundance of research oriented expertise is not always what’s heavily sought after.
Instead, many companies are looking for candidates who are up to date with the latest data science techniques and technologies, and are willing to pivot to match emerging trends and practices.
One recent development that is making the data science graduate school decisions more complex is the introduction of specialty master’s degrees, that focus on rigorous but compact, professional training. Both students and companies are realizing the value of an intensive, more industry-focused degree that can provide sufficient enough training to manage complex projects and that are more client oriented, opposed to research oriented.
However, not all prospective data science PhD students are looking for jobs in industry. There are some pretty amazing research opportunities opening up across a variety of academic fields that are making use of new data collection and analysis tools. Experts that understand how to leverage data systems including statistics and computer science to analyze trends and build models will be in high demand.
Can You Get a PhD in Data Science Online?
While it is not common to get a data science Ph.D. online, there are currently two options for those looking to take advantage of the flexibility of an online program.
Indiana University Bloomington and Northcentral University both offer online Ph.D. programs with either a minor or specialization in data science.
Given the trend for schools to continue increasing online offerings, expect to see additional schools adding this option in the near future.
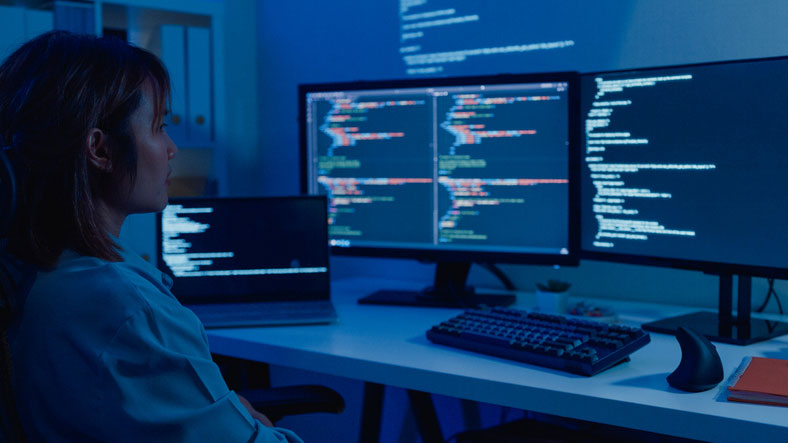
Overview of PhD Coursework
A PhD requires a lot of academic work, which generally requires between four and five years (sometimes longer) to complete.
Here are some of the high level factors to consider and evaluate when comparing data science graduate programs.
How many credits are required for a PhD in data science?
On average, it takes 71 credits to graduate with a PhD in data science — far longer (almost double) than traditional master’s degree programs. In addition to coursework, most PhD students also have research and teaching responsibilities that can be simultaneously demanding and really great career preparation.
What’s the core curriculum like?
In a data science doctoral program, you’ll be expected to learn many skills and also how to apply them across domains and disciplines. Core curriculums will vary from program to program, but almost all will have a core foundation of statistics.
All PhD candidates will have to take a qualifying exam. This can vary from university to university, but to give you some insight, it is broken up into three phases at Yale. They have a practical exam, a theory exam and an oral exam. The goal is to make sure doctoral students are developing the appropriate level of expertise.
Dissertation
One of the final steps of a PhD program involves presenting original research findings in a formal document called a dissertation. These will provide background and context, as well as findings and analysis, and can contribute to the understanding and evolution of data science. A dissertation idea most often provides the framework for how a PhD candidate’s graduate school experience will unfold, so it’s important to be thoughtful and deliberate while considering research opportunities.
Since data science is such a rapidly evolving field and because choosing the right PhD program is such an important factor in developing a successful career path, there are some steps that prospective doctoral students can take in advance to find the best-fitting opportunity.
Join professional associations
Even before being fully credentials, joining professional associations and organizations such as the Data Science Association and the American Association of Big Data Professionals is a good way to get exposure to the field. Many professional societies are welcoming to new members and even encourage student participation with things like discounted membership fees and awards and contest categories for student researchers. One of the biggest advantages to joining is that these professional associations bring together other data scientists for conference events, research-sharing opportunities, networking and continuing education opportunities.
Leverage your social network
Be on the lookout to make professional connections with professors, peers, and members of industry. There are a number of LinkedIn groups dedicated to data science. A well-maintained professional network is always useful to have when looking for advice or letters of recommendation while applying to graduate school and then later while applying for jobs and other career-related opportunities.
Kaggle competitions
Kaggle competitions provide the opportunity to solve real-world data science problems and win prizes. A list of data science problems can be found at Kaggle.com . Winning one of these competitions is a good way to demonstrate professional interest and experience.
Internships
Internships are a great way to get real-world experience in data science while also getting to work for top names in the world of business. For example, IBM offers a data science internship which would also help to stand out when applying for PhD programs, as well as in seeking employment in the future.
Demonstrating professional experience is not only important when looking for jobs, but it can also help while applying for graduate school. There are a number of ways for prospective students to gain exposure to the field and explore different facets of data science careers.
Get certified
There are a number of data-related certificate programs that are open to people with a variety of academic and professional experience. DeZyre has an excellent guide to different certifications, some of which might help provide good background for graduate school applications.
Conferences
Conferences are a great place to meet people presenting new and exciting research in the data science field and bounce ideas off of newfound connections. Like professional societies and organizations, discounted student rates are available to encourage student participation. In addition, some conferences will waive fees if you are presenting a poster or research at the conference, which is an extra incentive to present.
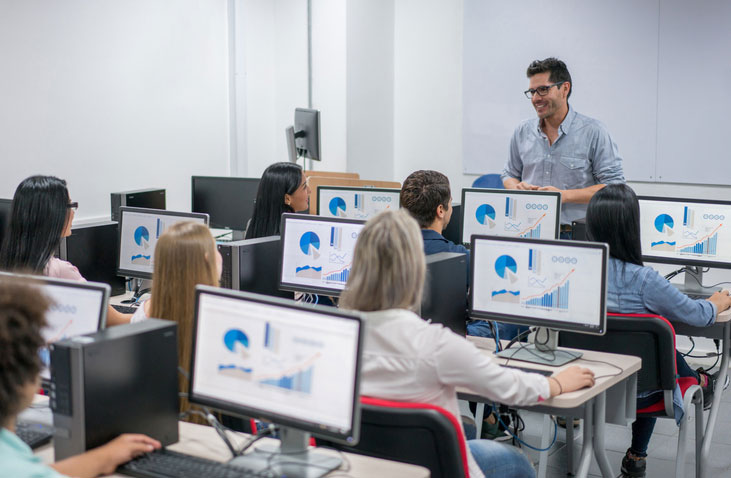
It can be hard to quantify what makes a good-fit when it comes to data science graduate school programs. There are easy to evaluate factors, such as cost and location, and then there are harder to evaluate criteria such as networking opportunities, accessibility to professors, and the up-to-dateness of the program’s curriculum.
Nevertheless, there are some key relevant considerations when applying to almost any data science graduate program.
What most schools will require when applying:
- All undergraduate and graduate transcripts
- A statement of intent for the program (reason for applying and future plans)
- Letters of reference
- Application fee
- Online application
- A curriculum vitae (outlining all of your academic and professional accomplishments)
What Does it Cost to Get a PhD in Data Science?
The great news is that many PhD data science programs are supported by fellowships and stipends. Some are completely funded, meaning the school will pay tuition and basic living expenses. Here are several examples of fully funded programs:
- University of Southern California
- University of Nevada, Reno
- Kennesaw State University
- Worcester Polytechnic Institute
- University of Maryland
For all other programs, the average range of tuition, depending on the school can range anywhere from $1,300 per credit hour to $2,000 amount per credit hour. Remember, typical PhD programs in data science are between 60 and 75 credit hours, meaning you could spend up to $150,000 over several years.
That’s why the financial aspects are so important to evaluate when assessing PhD programs, because some schools offer full stipends so that you are able to attend without having to find supplemental scholarships or tuition assistance.
Can I become a professor of data science with a PhD.? Yes! If you are interested in teaching at the college or graduate level, a PhD is the degree needed to establish the full expertise expected to be a professor. Some data scientists who hold PhDs start by entering the field of big data and pivot over to teaching after gaining a significant amount of work experience. If you’re driven to teach others or to pursue advanced research in data science, a PhD is the right degree for you.
Do I need a master’s in order to pursue a PhD.? No. Many who pursue PhDs in Data Science do not already hold advanced degrees, and many PhD programs include all the coursework of a master’s program in the first two years of school. For many students, this is the most time-effective option, allowing you to complete your education in a single pass rather than interrupting your studies after your master’s program.
Can I choose to pursue a PhD after already receiving my master’s? Yes. A master’s program can be an opportunity to get the lay of the land and determine the specific career path you’d like to forge in the world of big data. Some schools may allow you to simply extend your academic timeline after receiving your master’s degree, and it is also possible to return to school to receive a PhD if you have been working in the field for some time.
If a PhD. isn’t necessary, is it a waste of time? While not all students are candidates for PhDs, for the right students – who are keen on doing in-depth research, have the time to devote to many years of school, and potentially have an interest in continuing to work in academia – a PhD is a great choice. For more information on this question, take a look at our article Is a Data Science PhD. Worth It?
Complete List of Data Science PhD Programs
Below you will find the most comprehensive list of schools offering a doctorate in data science. Each school listing contains a link to the program specific page, GRE or a master’s degree requirements, and a link to a page with detailed course information.
Note that the listing only contains true data science programs. Other similar programs are often lumped together on other sites, but we have chosen to list programs such as data analytics and business intelligence on a separate section of the website.
Boise State University – Boise, Idaho PhD in Computing – Data Science Concentration
The Data Science emphasis focuses on the development of mathematical and statistical algorithms, software, and computing systems to extract knowledge or insights from data. Â
In 60 credits, students complete an Introduction to Graduate Studies, 12 credits of core courses, 6 credits of data science elective courses, 10 credits of other elective courses, a Doctoral Comprehensive Examination worth 1 credit, and a 30-credit dissertation.
Electives can be taken in focus areas such as Anthropology, Biometry, Ecology/Evolution and Behavior, Econometrics, Electrical Engineering, Earth Dynamics and Informatics, Geoscience, Geostatistics, Hydrology and Hydrogeology, Materials Science, and Transportation Science.
Delivery Method: Campus GRE: Required 2022-2023 Tuition: $7,236 total (Resident), $24,573 total (Non-resident)
View Course Offerings
Bowling Green State University – Bowling Green, Ohio Ph.D. in Data Science
Data Science students at Bowling Green intertwine knowledge of computer science with statistics.
Students learn techniques in analyzing structured, unstructured, and dynamic datasets.
Courses train students to understand the principles of analytic methods and articulating the strengths and limitations of analytical methods.
The program requires 60 credit hours in the studies of Computer Science (6 credit hours), Statistics (6 credit hours), Data Science Exploration and Communication, Ethical Issues, Advanced Data Mining, and Applied Data Science Experience.
Students must also complete 21 credit hours of elective courses, a qualifying exam, a preliminary exam, and a dissertation.
Delivery Method: Campus GRE: Required 2022-2023 Tuition: $8,418 (Resident), $14,410 (Non-resident)
Brown University – Providence, Rhode Island PhD in Computer Science – Concentration in Data Science
Brown University’s database group is a world leader in systems-oriented database research; they seek PhD candidates with strong system-building skills who are interested in researching TupleWare, MLbase, MDCC, Crowd DB, or PIQL.
In order to gain entrance, applicants should consider first doing a research internship at Brown with this group. Other ways to boost an application are to take and do well at massive open online courses, do an internship at a large company, and get involved in a large open-source software project.
Coding well in C++ is preferred.
Delivery Method: Campus GRE: Required 2022-2023 Tuition: $62,680 total
Chapman University – Irvine, California Doctorate in Computational and Data Sciences
Candidates for the doctorate in computational and data science at Chapman University begin by completing 13 core credits in basic methodologies and techniques of computational science.
Students complete 45 credits of electives, which are personalized to match the specific interests and research topics of the student.
Finally, students complete up to 12 credits in dissertation research.
Applicants must have completed courses in differential equations, data structures, and probability and statistics, or take specific foundation courses, before beginning coursework toward the PhD.
Delivery Method: Campus GRE: Required 2022-2023 Tuition: $37,538 per year
Clemson University / Medical University of South Carolina (MUSC) – Joint Program – Clemson, South Carolina & Charleston, South Carolina Doctor of Philosophy in Biomedical Data Science and Informatics – Clemson
The PhD in biomedical data science and informatics is a joint program co-authored by Clemson University and the Medical University of South Carolina (MUSC).
Students choose one of three tracks to pursue: precision medicine, population health, and clinical and translational informatics. Students complete 65-68 credit hours, and take courses in each of 5 areas: biomedical informatics foundations and applications; computing/math/statistics/engineering; population health, health systems, and policy; biomedical/medical domain; and lab rotations, seminars, and doctoral research.
Applicants must have a bachelor’s in health science, computing, mathematics, statistics, engineering, or a related field, and it is recommended to also have competency in a second of these areas.
Program requirements include a year of calculus and college biology, as well as experience in computer programming.
Delivery Method: Campus GRE: Required 2022-2023 Tuition: $10,858 total (South Carolina Resident), $22,566 total (Non-resident)
View Course Offerings – Clemson
George Mason University – Fairfax, Virginia Doctor of Philosophy in Computational Sciences and Informatics – Emphasis in Data Science
George Mason’s PhD in computational sciences and informatics requires a minimum of 72 credit hours, though this can be reduced if a student has already completed a master’s. 48 credits are toward graduate coursework, and an additional 24 are for dissertation research.
Students choose an area of emphasis—either computer modeling and simulation or data science—and completed 18 credits of the coursework in this area. Students are expected to completed the coursework in 4-5 years.
Applicants to this program must have a bachelor’s degree in a natural science, mathematics, engineering, or computer science, and must have knowledge and experience with differential equations and computer programming.
Delivery Method: Campus GRE: Required 2022-2023 Tuition: $13,426 total (Virginia Resident), $35,377 total (Non-resident)
Harrisburg University of Science and Technology – Harrisburg, Pennsylvania Doctor of Philosophy in Data Sciences
Harrisburg University’s PhD in data science is a 4-5 year program, the first 2 of which make up the Harrisburg master’s in analytics.
Beyond this, PhD candidates complete six milestones to obtain the degree, including 18 semester hours in doctoral-level courses, such as multivariate data analysis, graph theory, machine learning.
Following the completion of ANLY 760 Doctoral Research Seminar, students in the program complete their 12 hours of dissertation research bringing the total program hours to 36.
Delivery Method: Campus GRE: Required 2022-2023 Tuition: $14,940 total
Icahn School of Medicine at Mount Sinai – New York, New York Genetics and Data Science, PhD
As part of the Biomedical Science PhD program, the Genetics and Data Science multidisciplinary training offers research opportunities that expand on genetic research and modern genomics. The training also integrates several disciplines of biomedical sciences with machine learning, network modeling, and big data analysis.
Students in the Genetics and Data Science program complete a predetermined course schedule with a total of 64 credits and 3 years of study.
Additional course requirements and electives include laboratory rotations, a thesis proposal exam and thesis defense, Computer Systems, Intro to Algorithms, Machine Learning for Biomedical Data Science, Translational Genomics, and Practical Analysis of a Personal Genome.
Delivery Method: Campus GRE: Not Required 2022-2023 Tuition: $31,303 total
Indiana University-Purdue University Indianapolis – Indianapolis, Indiana PhD in Data Science PhD Minor in Applied Data Science
Doctoral candidates pursuing the PhD in data science at Indiana University-Purdue must display competency in research, data analytics, and at management and infrastructure to earn the degree.
The PhD is comprised of 24 credits of a data science core, 18 credits of methods courses, 18 credits of a specialization, written and oral qualifying exams, and 30 credits of dissertation research. All requirements must be completed within 7 years.
Applicants are generally expected to have a master’s in social science, health, data science, or computer science.Â
Currently a majority of the PhD students at IUPUI are funded by faculty grants and two are funded by the federal government. None of the students are self funded.
IUPUI also offers a PhD Minor in Applied Data Science that is 12-18 credits. The minor is open to students enrolled at IUPUI or IU Bloomington in a doctoral program other than Data Science.
Delivery Method: Campus GRE: Required 2022-2023 Tuition: $9,228 per year (Indiana Resident), $25,368 per year (Non-resident)
Jackson State University –Â Jackson, Mississippi PhD Computational and Data-Enabled Science and Engineering
Jackson State University offers a PhD in computational and data-enabled science and engineering with 5 concentration areas: computational biology and bioinformatics, computational science and engineering, computational physical science, computation public health, and computational mathematics and social science.
Students complete 12 credits of common core courses, 12 credits in the specialization, 24 credits of electives, and 24 credits in dissertation research.
Students may complete the doctoral program in as little as 5 years and no more than 8 years.
Delivery Method: Campus GRE: Required 2022-2023 Tuition: $8,270 total
Kennesaw State University – Kennesaw, Georgia PhD in Analytics and Data Science
Students pursuing a PhD in analytics and data science at Kennesaw State University must complete 78 credit hours: 48 course hours and 6 electives (spread over 4 years of study), a minimum 12 credit hours for dissertation research, and a minimum 12 credit-hour internship.
Prior to dissertation research, the comprehensive examination will cover material from the three areas of study: computer science, mathematics, and statistics.
Successful applicants will have a master’s degree in a computational field, calculus I and II, programming experience, modeling experience, and are encouraged to have a base SAS certification.
Delivery Method: Campus GRE: Required 2022-2023 Tuition: $5,328 total (Georgia Resident), $19,188 total (Non-resident)
New Jersey Institute of Technology – Newark, New Jersey PhD in Business Data Science
Students may enter the PhD program in business data science at the New Jersey Institute of Technology with either a relevant bachelor’s or master’s degree. Students with bachelor’s degrees begin with 36 credits of advanced courses, and those with master’s take 18 credits before moving on to credits in dissertation research.
Core courses include business research methods, data mining and analysis, data management system design, statistical computing with SAS and R, and regression analysis.
Students take qualifying examinations at the end of years 1 and 2, and must defend their dissertations successfully by the end of year 6.
Delivery Method: Campus GRE: Required 2022-2023 Tuition: $21,932 total (New Jersey Resident), $32,426 total (Non-resident)
New York University – New York, New York PhD in Data Science
Doctoral candidates in data science at New York University must complete 72 credit hours, pass a comprehensive and qualifying exam, and defend a dissertation with 10 years of entering the program.
Required courses include an introduction to data science, probability and statistics for data science, machine learning and computational statistics, big data, and inference and representation.
Applicants must have an undergraduate or master’s degree in fields such as mathematics, statistics, computer science, engineering, or other scientific disciplines. Experience with calculus, probability, statistics, and computer programming is also required.
Delivery Method: Campus GRE: Required 2022-2023 Tuition: $37,332 per year
View Course Offering
Northcentral University – San Diego, California PhD in Data Science-TIM
Northcentral University offers a PhD in technology and innovation management with a specialization in data science.
The program requires 60 credit hours, including 6-7 core courses, 3 in research, a PhD portfolio, and 4 dissertation courses.
The data science specialization requires 6 courses: data mining, knowledge management, quantitative methods for data analytics and business intelligence, data visualization, predicting the future, and big data integration.
Applicants must have a master’s already.
Delivery Method: Online GRE: Required 2022-2023 Tuition: $16,794 total
Stevens Institute of Technology –Â Hoboken, New Jersey Ph.D. in Data Science
Stevens Institute of Technology has developed a data science Ph.D. program geared to help graduates become innovators in the space.
The rigorous curriculum emphasizes mathematical and statistical modeling, machine learning, computational systems and data management.
The program is directed by Dr. Ted Stohr, a recognized thought leader in the information systems, operations and business process management arenas.
Delivery Method: Campus GRE: Required 2022-2023 Tuition: $39,408 per year
University at Buffalo –Â Buffalo, New York PhD Computational and Data-Enabled Science and Engineering
The curriculum for the University of Buffalo’s PhD in computational and data-enabled science and engineering centers around three areas: data science, applied mathematics and numerical methods, and high performance and data intensive computing. 9 credit course of courses must be completed in each of these three areas. Altogether, the program consists of 72 credit hours, and should be completed in 4-5 years. A master’s degree is required for admission; courses taken during the master’s may be able to count toward some of the core coursework requirements.
Delivery Method: Campus GRE: Required 2022-2023 Tuition: $11,310 per year (New York Resident), $23,100 per year (Non-resident)
University of Colorado Denver –Â Denver, Colorado PhD in Big Data Science and Engineering
The University of Colorado – Denver offers a unique program for those students who have already received admission to the computer science and information systems PhD program.
The Big Data Science and Engineering (BDSE) program is a PhD fellowship program that allows selected students to pursue research in the area of big data science and engineering. This new fellowship program was created to train more computer scientists in data science application fields such as health informatics, geosciences, precision and personalized medicine, business analytics, and smart cities and cybersecurity.
Students in the doctoral program must complete 30 credit hours of computer science classes beyond a master’s level, and 30 credit hours of dissertation research.
The BDSE fellowship requires students to have an advisor both in the core disciplines (either computer science or mathematics and statistics) as well as an advisor in the application discipline (medicine and public health, business, or geosciences).
In addition, the fellowship covers full stipend, tuition, and fees up to ~50k for BDSE fellows annually. Important eligibility requirements can be found here.
Delivery Method: Campus GRE: Required 2022-2023 Tuition: $55,260 total
University of Marylan d – College Park, Maryland PhD in Information Studies
Data science is a potential research area for doctoral candidates in information studies at the University of Maryland – College Park. This includes big data, data analytics, and data mining.
Applicants for the PhD must have taken the following courses in undergraduate studies: programming languages, data structures, design and analysis of computer algorithms, calculus I and II, and linear algebra.
Students must complete 6 qualifying courses, 2 elective graduate courses, and at least 12 credit hours of dissertation research.
Delivery Method: Campus GRE: Required 2022-2023 Tuition: $16,238 total (Maryland Resident), $35,388 total (Non-resident)
University of Massachusetts Boston – Boston, Massachusetts PhD in Business Administration – Information Systems for Data Science Track
The University of Massachusetts – Boston offers a PhD in information systems for data science. As this is a business degree, students must complete coursework in their first two years with a focus on data for business; for example, taking courses such as business in context: markets, technologies, and societies.
Students must take and pass qualifying exams at the end of year 1, comprehensive exams at the end of year 2, and defend their theses at the end of year 4.
Those with a degree in statistics, economics, math, computer science, management sciences, information systems, and other related fields are especially encouraged, though a quantitative degree is not necessary.
Students accepted by the program are ordinarily offered full tuition credits and a stipend ($25,000 per year) to cover educational expenses and help defray living costs for up to three years of study.
During the first two years of coursework, they are assigned to a faculty member as a research assistant; for the third year students will be engaged in instructional activities. Funding for the fourth year is merit-based from a limited pool of program funds
Delivery Method: Campus GRE: Required 2022-2023 Tuition: $18,894 total (in-state), $36,879 (out-of-state)
University of Nevada Reno –Â Reno, Nevada PhD in Statistics and Data Science
The University of Nevada – Reno’s doctoral program in statistics and data science is comprised of 72 credit hours to be completed over the course of 4-5 years. Coursework is all within the scope of statistics, with titles such as statistical theory, probability theory, linear models, multivariate analysis, statistical learning, statistical computing, time series analysis.
The completion of a Master’s degree in mathematics or statistics prior to enrollment in the doctoral program is strongly recommended, but not required.
Delivery Method: Campus GRE: Required 2022-2023 Tuition: $5,814 total (in-state), $22,356 (out-of-state)
University of Southern California –Â Los Angles, California PhD in Data Sciences & Operations
USC Marshall School of Business offers a PhD in data sciences and operations to be completed in 5 years.
Students can choose either a track in operations management or in statistics. Both tracks require 4 courses in fall and spring of the first 2 years, as well as a research paper and courses during the summers. Year 3 is devoted to dissertation preparation and year 4 and/or 5 to dissertation defense.
A bachelor’s degree is necessary for application, but no field or further experience is required.
Students should complete 60 units of coursework. If the students are admitted with Advanced Standing (e.g., Master’s Degree in appropriate field), this requirement may be reduced to 40 credits.
Delivery Method: Campus GRE: Required 2022-2023 Tuition: $63,468 total
University of Tennessee-Knoxville – Knoxville, Tennessee The Data Science and Engineering PhD
The data science and engineering PhD at the University of Tennessee – Knoxville requires 36 hours of coursework and 36 hours of dissertation research. For those entering with an MS degree, only 24 hours of course work is required.
The core curriculum includes work in statistics, machine learning, and scripting languages and is enhanced by 6 hours in courses that focus either on policy issues related to data, or technology entrepreneurship.
Students must also choose a knowledge specialization in one of these fields: health and biological sciences, advanced manufacturing, materials science, environmental and climate science, transportation science, national security, urban systems science, and advanced data science.
Applicants must have a bachelor’s or master’s degree in engineering or a scientific field.Â
All students that are admitted will be supported by a research fellowship and tuition will be included.
Many students will perform research with scientists from Oak Ridge national lab, which is located about 30 minutes drive from campus.
Delivery Method: Campus GRE: Required 2022-2023 Tuition: $11,468 total (Tennessee Resident), $29,656 total (Non-resident)
University of Vermont –Â Burlington, Vermont Complex Systems and Data Science (CSDS), PhD
Through the College of Engineering and Mathematical Sciences, the Complex Systems and Data Science (CSDS) PhD program is pan-disciplinary and provides computational and theoretical training. Students may customize the program depending on their chosen area of focus.
Students in this program work in research groups across campus.
Core courses include Data Science, Principles of Complex Systems and Modeling Complex Systems. Elective courses include Machine Learning, Complex Networks, Evolutionary Computation, Human/Computer Interaction, and Data Mining.
The program requires at least 75 credits to graduate with approval by the student graduate studies committee.
Delivery Method: Campus GRE: Not Required 2022-2023 Tuition: $12,204 total (Vermont Resident), $30,960 total (Non-resident)
University of Washington Seattle Campus –Â Seattle, Washington PhD in Big Data and Data Science
The University of Washington’s PhD program in data science has 2 key goals: training of new data scientists and cyberinfrastructure development, i.e., development of open-source tools and services that scientists around the world can use for big data analysis.
Students must take core courses in data management, machine learning, data visualization, and statistics.
Students are also required to complete at least one internship that covers practical work in big data.
Delivery Method: Campus GRE: Required 2022-2023 Tuition: $17,004 per year (Washington resident), $30,477 (non-resident)
University of Wisconsin-Madison –Â Madison, Wisconsin PhD in Biomedical Data Science
The PhD program in Biomedical Data Science offered by the Department of Biostatistics and Medical Informatics at UW-Madison is unique, in blending the best of statistics and computer science, biostatistics and biomedical informatics.Â
Students complete three year-long course sequences in biostatistics theory and methods, computer science/informatics, and a specialized sequence to fit their interests.
Students also complete three research rotations within their first two years in the program, to both expand their breadth of knowledge and assist in identifying a research advisor.
Delivery Method: Campus GRE: Required 2022-2023 Tuition: $10,728 total (in-state), $24,054 total (out-of-state)
Vanderbilt University –Â Nashville, Tennessee Data Science Track of the BMI PhD Program
The PhD in biomedical informatics at Vanderbilt has the option of a data science track.
Students complete courses in the areas of biomedical informatics (3 courses), computer science (4 courses), statistical methods (4 courses), and biomedical science (2 courses). Students are expected to complete core courses and defend their dissertations within 5 years of beginning the program.
Applicants must have a bachelor’s degree in computer science, engineering, biology, biochemistry, nursing, mathematics, statistics, physics, information management, or some other health-related field.
Delivery Method: Campus GRE: Required 2022-2023 Tuition: $53,160 per year
Washington University in St. Louis –Â St. Louis, Missouri Doctorate in Computational & Data Sciences
Washington University now offers an interdisciplinary Ph.D. in Computational & Data Sciences where students can choose from one of four tracks (Computational Methodologies, Political Science, Psychological & Brain Sciences, or Social Work & Public Health).
Students are fully funded and will receive a stipend for at least five years contingent on making sufficient progress in the program.
Delivery Method: Campus GRE: Required 2022-2023 Tuition: $59,420 total
Worcester Polytechnic Institute –Â Worcester, Massachusetts PhD in Data Science
The PhD in data science at Worcester Polytechnic Institute focuses on 5 areas: integrative data science, business intelligence and case studies, data access and management, data analytics and mining, and mathematical analysis.
Students first complete a master’s in data science, and then complete 60 credit hours beyond the master’s, including 30 credit hours of research.
Delivery Method: Campus GRE: Required 2022-2023 Tuition: $28,980 per year
Yale University –Â New Haven, Connecticut PhD Program – Department of Stats and Data Science
The PhD in statistics and data science at Yale University offers broad training in the areas of statistical theory, probability theory, stochastic processes, asymptotics, information theory, machine learning, data analysis, statistical computing, and graphical methods. Students complete 12 courses in the first year in these topics.
Students are required to teach one course each semester of their third and fourth years.
Most students complete and defend their dissertations in their fifth year.
Applicants should have an educational background in statistics, with an undergraduate major in statistics, mathematics, computer science, or similar field.
Delivery Method: Campus GRE: Required 2022-2023 Tuition: $46,900 total
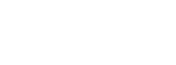
- Related Programs
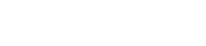
PhD in Data Science: Program Overview
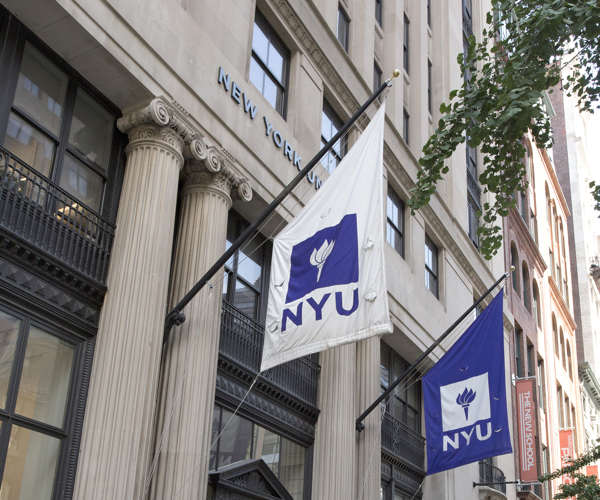
The field of data science has emerged from advances in computational speed, data availability, and novel analysis methods. It demands a new type of researcher: the rigorously trained, cross-disciplinary, and ethically responsible data scientist.
Launched in Fall 2017, the pioneering CDS PhD Data Science program seeks to produce such researchers. It has gained recognition as one of the top and most selective data science doctoral programs globally, earning an NSF NRT training grant.
CDS PhD students are also being recognized for their outstanding contributions to the field of data science through fellowships and awards that range from industry-sponsored programs like Microsoft Research, Google, and Apple to academic honors such as the National Science Foundation Graduate Research Fellowship.
The CDS PhD program rigorously trains future data scientists to:
- Develop methodologies and use statistical tools to answer cross-disciplinary questions
- Clearly communicate to extract precise questions from big, heterogeneous, uncertain data
- Translate research insights into practical applications across sciences, medicine, industry, and government
- Understand the ethical implications of their work
Our mission is to nurture this new generation of data scientists in an environment where methodological innovations are developed and successfully applied to various domains. We believe that combining fundamental research on the principles of data science with translational projects involving domain experts creates a virtuous cycle, advancing both data science methodology and real-world applications.
The program has an innovative core curriculum, a novel data assistantship mechanism that provides training of skills transfer through rotations and internships, and communication and entrepreneurship modules. Students are exposed to a wider range of fields than in traditional PhD programs while working with our interdisciplinary faculty. We also offer a medical school track for those interested in healthcare applications or developing novel theoretical models from medical questions.
Successful candidates are guaranteed financial support, including tuition and a competitive stipend, for up to five years in the fall and spring semesters.*
We invite you to learn more through our webpage or by contacting [email protected] . *Students also have the opportunity to pursue their study and research with Data Science faculty at NYU Shanghai . With this opportunity, students generally complete their coursework in New York City before moving full-time to Shanghai for their research. For more information, please visit the NYU Shanghai Ph.D. page .
Start typing and press enter to search

PhD in Data Science
Students conduct research on cutting edge problems alongside preeminent faculty at UChicago and explore the emerging field of Data Science. As an emerging discipline, Data Science addresses foundational problems across the entire data life cycle. Tackling issues of inequity, climate change, and sustainability will require cutting edge research in artificial intelligence and data usage combined with innovative educational programs to train students in the concepts of information systems. Students of Data Science will not only immerse themselves in a rapidly evolving field; they will help redefine it altogether.
Research Excellence:
As a PhD student in Data Science, you will learn from faculty who have developed research programs that span a wide variety of data science and AI topics, from theory to applications, with a focus on making a societal impact.
Research Topics:
- Artificial Intelligence
- Data, AI, and Society
- Data Systems
- Human-Centered Data Science
- Machine Learning and Statistics
- Use-Inspired Data Science
For more information, including a link to the application, see the Committee on Data Science website .

- Mission and Goals
- DEI Commitment and Resources
- In Memoriam
- The Halıcıoğlu Challenge
- 5-Year Report
- Administration
- Visiting Scholars
- Faculty Affiliates
- Artificial Intelligence and Machine Learning
- Biomedical Data Science
- Data Infrastructure and Systems
- Data Science for Scientific Discovery
- Data and Society
- Theoretical Foundations of Data Science
- Visiting Scholar Program
- MS & PhD Admissions
- MSDS Course Requirements
- Degree Questions
- PhD Students
- PhD Course Requirements
- PhD Student Resources
- Research Rotation
- Spring Evaluation Requirements
- Course Descriptions
- Course Offerings
- Career Services
- Funding Initiatives for Students
- Graduate Advising
- Online Masters Program
- Academic Advising
- Concurrent Enrollment
- Course Descriptions and Prerequisites
- Enrolling in Classes
- Financial Opportunities
- Major Requirements
- Minor Requirements
- OSD Accommodations
- Petition Instructions
- Selective Major Application
- Prospective Double Majors
- Prospective First-Year Students
- Prospective Transfer Students
- Partnership Programs
- Research Collaboration
- Access to Talent
- Professional Development
- UCTV Data Science Channel
- Alumni Relations
- Giving Back
Give us a call or drop by anytime, we endeavor to answer all inquiries within 24 hours.
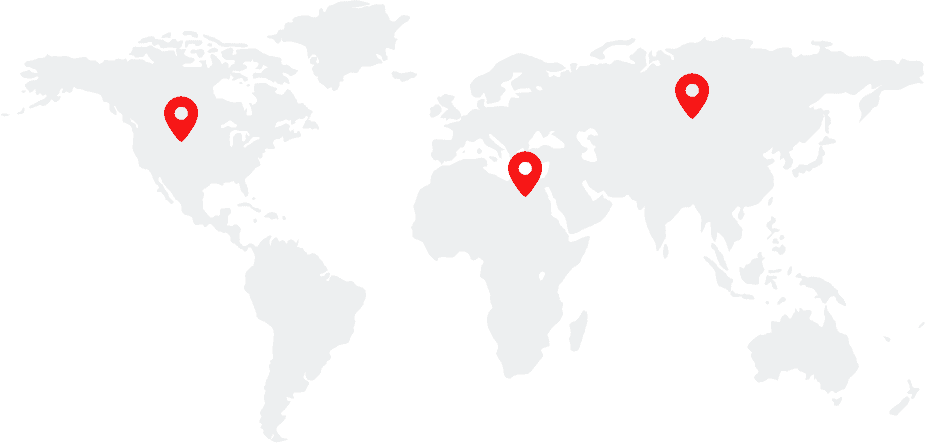
PO Box 16122 Collins Street West Victoria, Australia
[email protected] / [email protected]
Phone support
Phone: + (066) 0760 0260 / + (057) 0760 0560
PhD Program
Requirements for doctor of philosophy (ph.d.) in data science.
The goal of the doctoral program is to create leaders in the field of Data Science who will lay the foundation and expand the boundaries of knowledge in the field. The doctoral program aims to provide a research-oriented education to students, teaching them knowledge, skills and awareness required to perform data driven research, and enabling them to, using this shared background, carry out research that expands the boundaries of knowledge in Data Science. The doctoral program spans from foundational aspects, including computational methods, machine learning, mathematical models and statistical analysis, to applications in data science.
Course Requirements
https://datascience.ucsd.edu/graduate/phd-program/phd-course-requirements/Â
Research Rotation Program
https://datascience.ucsd.edu/graduate/phd-program/research-rotation/
Preliminary Assessment Examination
The goal of the preliminary assessment examination is to assess students’ preparation for pursuing a PhD in data science, in terms of core knowledge and readiness for conducting research. The preliminary assessment is an advisory examination.
The preliminary assessment is an oral presentation that must be completed before the end of Spring quarter of the second academic year. Students must have a GPA of 3.0 or above to qualify for the assessment and have completed three of four core required courses . The student will choose a committee consisting of three members, one of which will be the HDSI academic advisor of the student. The other two committee members must be HDSI faculty members with 0% or more appointments; we encourage the student to select the second faculty member based on compatibility of research interests and topic of the presentation. The student is responsible for scheduling the meeting and making a room reservation.Â
The student may choose to be evaluated based on (A) a scientific literature survey and data analysis or (B) based on a previous rotation project. The student will propose the topic of the presentation.Â
- If the student chooses the survey theme, they should select a broad area that is well represented among HDSI faculty members, such as causal inference, responsible AI, optimization, etc. The student should survey at least 10 peer-reviewed conference or journal papers representative of the last (at least) 5 years of the field. The student should present a novel and rigorous original analysis using publicly available data from the surveyed literature: this analysis may aim to answer a related or new research question.
- Â If the student chooses the rotation project theme, they should prepare to discuss the motivation for the project, the analysis undertaken, and the outcome of the rotation.Â
For both themes, the student will describe their topic to the committee by writing a 1-2 page proposal that must be then approved by the committee. We emphasize that this is not a research proposal. The student will have 50 minutes to give an oral presentation which should include a comprehensive overview of previous work, motivation for the presented work or state-of-the-art studies, a critical assessment of previous work and of their own work, and a future outlook including logical next steps or unanswered questions. The presentation will then be followed by a Q&A session by the committee members; the entire exam is expected to finish within two hours.Â
The committee will assess both the oral presentation as well as the student’s academic performance so far (especially in the required core courses). The committee will evaluate preparedness, technical skills, comprehension, critical thinking, and research readiness. Students who do not receive a satisfactory evaluation will receive a recommendation from the Graduate Program Committee regarding ways to remedy the lacking preparation or an opportunity to receive a terminal MS in Data Science degree provided the student can meet the degree requirements of the MS program . If the lack of preparation is course-based, the committee can require that additional course(s) be taken to pass the exam. If the lack of preparation is research-based, the committee can require an evaluation after another quarter of research with an HDSI faculty member; the faculty member will provide this evaluation. The preliminary assessment must be successfully completed no later than completion of two years (or sixth quarter enrollment) in the Ph.D. program.Â
The oral presentation must be completed in-person. We recommend the following timeline so that students can plan their preliminary assessments:
- Middle of winter quarter of second year: Student selects committee and proposes preliminary exam topic. Â
- Beginning of spring quarter of second year: Scheduling of exam is completed.Â
- End of spring quarter of second year: Exam.Â
Research Qualifying Examination and Advancing to Candidacy
A research qualifying examination (UQE) is conducted by the dissertation committee consisting of five or more members approved by the graduate division as per senate regulation 715(D). One senate faculty member must have a primary appointment in the department outside of HDSI. Faculty with 25% or less partial appointment in HDSI may be considered for meeting this requirement on an exceptional basis upon approval from the graduate division.
The goal of UQE is to assess the ability of the candidate to perform independent critical research as evidenced by a presentation and writing a technical report at the level of a peer-reviewed journal or conference publication. The examination is taken after the student and his or her adviser have identified a topic for the dissertation and an initial demonstration of feasible progress has been made. The candidate is expected to describe his or her accomplishments to date as well as future work. The research qualifying examination must be completed no later than fourth year or 12 quarters from the start of the degree program; the UQE is tantamount to the advancement to PhD candidacy exam.
A petition to the Graduate Committee is required for students who take UQE after the required 12 quarters deadline. Students who fail the research qualifying examination may file a petition to retake it; if the petition is approved, they will be allowed to retake it one (and only one) more time. Students who fail UQE may also petition to transition to a MS in Data Science track.
Dissertation Defense Examination and Thesis Requirements
Students must successfully complete a final dissertation defense oral presentation and examination to the Dissertation Committee consisting of five or more members approved by the graduate division as per senate regulation 715(D). One senate faculty member in the Dissertation Committee must have a primary appointment in a department outside of HDSI. Partially appointed faculty in HDSI (at 25% or less) are acceptable in meeting this outside-department requirement as long as their main (lead) department is not HDSI.
A dissertation in the scope of Data Science is required of every candidate for the PhD degree. HDSI PhD program thesis requirements must meet Regulation 715(D) requirements. The final form of the dissertation document must comply with published guidelines by the Graduate Division.
The dissertation topic will be selected by the student, under the advice and guidance of Thesis Adviser and the Dissertation Committee. The dissertation must contain an original contribution of quality that would be acceptable for publication in the academic literature that either extends the theory or methodology of data science, or uses data science methods to solve a scientific problem in applied disciplines.
The entire dissertation committee will conduct a final oral examination, which will deal primarily with questions arising out of the relationship of the dissertation to the field of Data Science. The final examination will be conducted in two parts. The first part consists of a presentation by the candidate followed by a brief period of questions pertaining to the presentation; this part of the examination is open to the public. The second part of the examination will immediately follow the first part; this is a closed session between the student and the committee and will consist of a period of questioning by the committee members.
Special Requirements: Generalization, Reproducibility and Responsibility A candidate for doctoral degree in data science is expected to demonstrate evidence of generalization skills as well as evidence of reproducibility in research results. Evidence of generalization skills may be in the form of — but not limited to — generalization of results arrived at across domains, or across applications within a domain, generalization of applicability of method(s) proposed, or generalization of thesis conclusions rooted in formal or mathematical proof or quantitative reasoning supported by robust statistical measures. Reproducibility requirement may be satisfied by additional supplementary material consisting of code and data repository. The dissertation will also be reviewed for responsible use of data.
Special Requirements: Professional Training and Communications
All graduate students in the doctoral program are required to complete at least one quarter of experience in the classroom as teaching assistants regardless of their eventual career goals. Effective communications and ability to explain deep technical subjects is considered a key measure of a well-rounded doctoral education. Thus, Ph.D. students are also required to take a 1-unit DSC 295 (Academia Survival Skills) course for a Satisfactory grade.
Obtaining an MS in Data Science
PhD students may obtain an MS Degree in Data Science along the way or a terminal MS degree, provided they complete the requirements for the MS degree.
Course Exceptions: Students with MS in Data Science (or similar field)
If a student has already been granted a Master’s degree in Data Science (or a related field, as determined by the Graduate Program Committee) before entering the HDSI PhD program, the student can submit a “Requirement Substitution” petition for up to 2 courses to be substituted by DSC 299 (up to 8 units).
Further leniency may be granted in exceptional cases in which both the student and their faculty advisor must separately appeal to the Graduate Program Committee. It is up to the Graduate Program Committee to decide whether the appeal is rejected or granted in part or in its entirety.
Table of Contents
What is a phd in data science, phd vs master's degree in data science, why earn a phd in data science, phd benefits, phd disadvantages, careers for data science phd holders, phd in data science curriculum, considerations when choosing a phd in data science program or college, phd in data science preparation courses, how much does a phd in data science cost, phd in data science: a guide to choose a doctoral program.
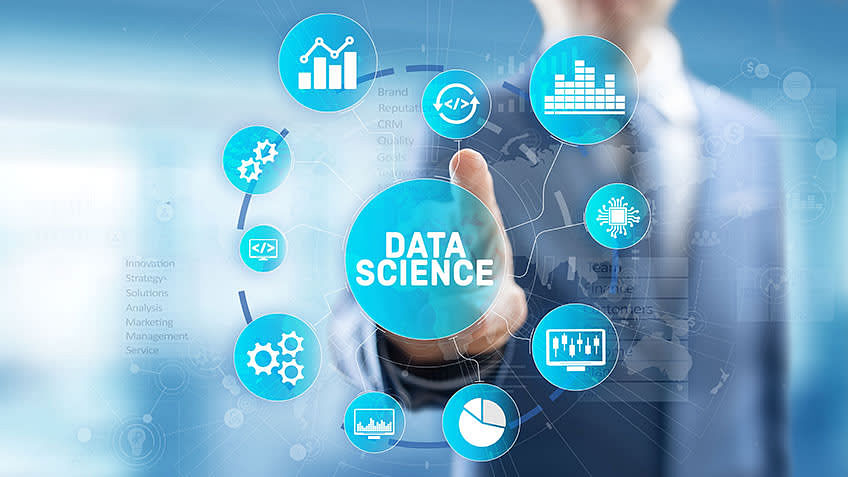
Throughout the world, data is revolutionizing and propelling enterprises. Nearly all industries require data scientists . To excel in data science, aspirants can consider getting a PhD in data science.
This article discusses everything one needs to know about PhD in data science.
A PhD in Data Science is the highest qualification for an aspiring data scientist. For anyone who wants to be at the highest point of their field, getting a PhD is the answer. It demonstrates to employers and fellow professionals that you are an expert in data science. This degree highly relies on research work.
Do you need a PhD in Data Science or a Master’s is enough?
- PhD in Data Science: A PhD in data science emphasizes on conducting deep research on a particular specialized topic. Normally, it takes four to five years to finish, though it often takes longer based on a variety of individual reasons.
- Master’s: Master's in data science program focuses a strong emphasis on giving students the practical skill sets they need to get ready for the workforce. Normally, it takes one to two years to finish.
A Doctorate in data science is perefct for anyone who wants to excel in their career as a data scientist. A PhD is your ideal match if you want to conduct research in specific specialization. Along with studying and researching, you could also work as an instructor in an institution.
These are the benefits of getting a PhD in Data Science:
- It will improve your data analysis abilities and allow you to specialize in areas where no one else can.
- It gets you ready for some of the most innovative research.
- It might aid in finding solutions to genuine issues.
- A data scientist with this degree is highly competent.
These are the drawbacks of getting a PhD in Data Science:
- If you cannot locate or own a means of funding it, it will be pricey.
- It involves reading and writing for long periods alone.
- Time-consuming is a possibility.
PhD programs in data science offer a wide range of professional options:
Data Scientist
- Research Scientist
- Data Engineer
- Data Analyst
- Database Administrator
- Machine Learning Engineer
- Data Architect
- Statistician
- Business Analyst
Become a Data Science & Business Analytics Professional
- 28% Annual Job Growth by 2026
- 11.5 M Expected New Data Science Jobs by 2026
- $86K - $157K Average Annual Salary
Caltech Post Graduate Program in Data Science
- Earn a program completion certificate from Caltech CTME
- Curriculum delivered in live online sessions by industry experts
- Industry-recognized Data Scientist Master’s certificate from Simplilearn
- Dedicated live sessions by faculty of industry experts
Here's what learners are saying regarding our programs:
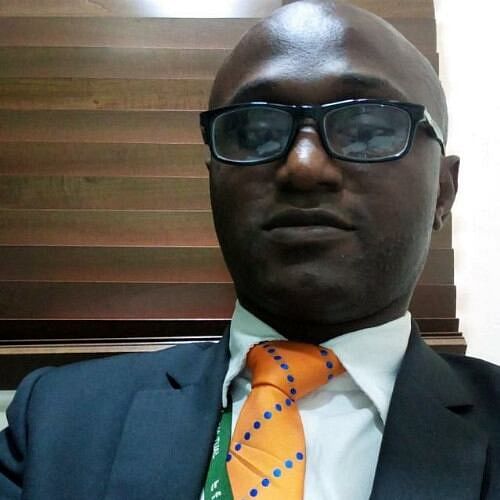
Ikenna Uwaezuoke
Data analyst , healing springs med- care llc.
Enrolling in the Post Graduate Program in Data Science from Caltech CTME transformed my career. Simplilearn's top-notch curriculum and expert trainers far exceeded my expectations, boosting my confidence in handling complex Machine Learning projects. It was the most impactful learning experience learning from the United States.
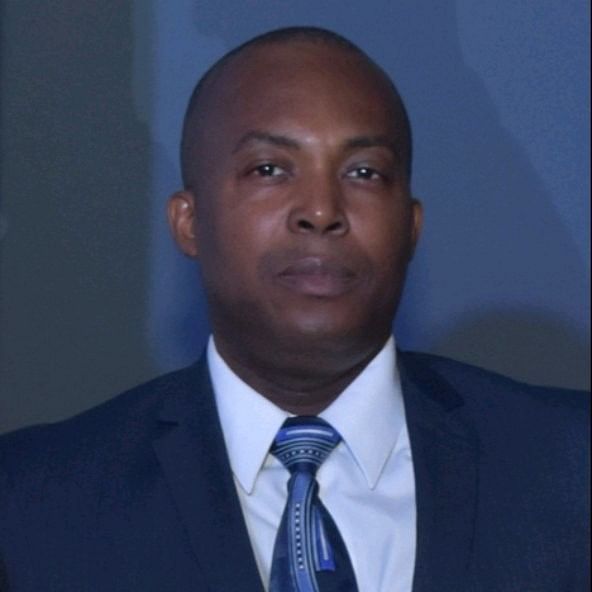
A.Anthony Davis
Simplilearn United States has one of the best programs available online to earn real-world skills that are in demand worldwide. I just completed the Machine Learning Advanced course, and the LMS was excellent.
The basic curriculum of PhD in Data Science includes:
- Complete over 70 credits while keeping your cumulative grade point average at 3.0 each semester.
- For electives, complete almost 40 credits.
- Fulfill the prerequisites for teaching.
- Ace the comprehensive test.
- All requirements for the PhD dissertation's approval must be met.
When applying to practically any graduate school in data science, there are a few important factors to know.
- Admission Requirements: Generally, students should have at least a bachelor's degree; however, some schools require master's degree holders.
- Dissertation: The dissertation proposal must be approved by the faculty.
- Staff: PhD candidates should take into account the qualifications, standing, and diversity of the professors.
- Cost: For many students, choosing a school is heavily influenced by cost and financial aid.
Before writing a research proposal for your PhD program, considering a data science preparation course can be helpful.
- Simplilearn’s PGP in Data Science : Before continuing to the more complex concepts in data science, students who want to begin and complete the basic portion of the curriculum should choose this course.
- Praxis Business School’s PGP in Data Science: Via in-class lectures, assignments, and projects, the curriculum provides the students with skills in business, technology, and other fields.
- Data Science Specialization by John Hopkins University: Across the whole data science workflow, this program covers the ideas and technologies you'll need.
- IBM Data Science Course: The nine courses in this program will provide you with the most recent job-ready methods and skills in a wide range of fields of data science.
The cost of a Doctorate in data science will vary depending on the institution you attend. The average cost of a Doctorate is roughly $30,000. The cost of four years of schooling would be $120,000.
A Doctorate will put you in a good position to pursue this if you want to take part in data science workflow, which involves not only using libraries and ideas but also generating them. You can go for a data science boot camp if you don't want to do a PhD Simplilearn’s Data Science Bootcamp is the best boot camp for data science students.
1. Can You Get a PhD in Data Science Online?
Yes, there are many institutions that offer data science courses online. You need to research thoroughly before choosing the best PhD online program.
2. What Does it Cost to Get a PhD in Data Science?
A PhD in Data Science can be costly if you do not have the right funds. However, you can anticipate spending over $100,000 on this course.
3. Should you really get a PhD in Data Science?
For experts who have already made a name for themselves, a data science PhD may not be required. Yet, this is the greatest certification that can be earned, and it will boost your reputation in the field.
4. What is the length of a data science PhD?
PhD in data science generally takes up to five years.
5. What is the scope of a PhD in data science?
You may develop your career and become prepared for research in the area with a Doctorate in data science. But, your individual objectives and financial capacity will determine if you should pursue one.
6. Which PhD is best for data science?
There are various data science PhD specializations: Statistics, Machine Learning, Data Analytics, etc.
7. Is PhD in data science difficult?
A Doctorate can be challenging and frustrating. Writing up a Doctorate can require several months of diligent labor.
8. What is a good salary after PhD?
After earning a Doctorate, the average pay in data science might reach $141,400 annually according to Glassdoor. That could be increased to $227K or more with extra training.
Data Science & Business Analytics Courses Duration and Fees
Data Science & Business Analytics programs typically range from a few weeks to several months, with fees varying based on program and institution.
Get Free Certifications with free video courses
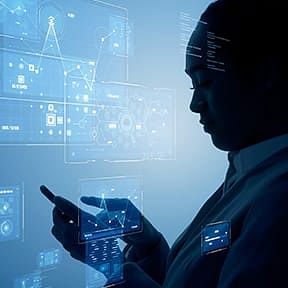
Data Science & Business Analytics
Introduction to Data Science
Learn from Industry Experts with free Masterclasses
Career Masterclass: Learn How to Conquer Data Science in 2023
Program Overview: Turbocharge Your Data Science Career With Caltech CTME
Why Data Science Should Be Your Top Career Choice for 2024 with Caltech University
Recommended Reads
Data Science Career Guide: A Comprehensive Playbook To Becoming A Data Scientist
Data Science vs. Big Data vs. Data Analytics
The Best Introduction to Data Science
Data Science Interview Guide
Data Science Course Syllabus and Subjects
How to Become a Data Scientist
Get Affiliated Certifications with Live Class programs
- PMP, PMI, PMBOK, CAPM, PgMP, PfMP, ACP, PBA, RMP, SP, OPM3 and the PMI ATP seal are the registered marks of the Project Management Institute, Inc.

IMAGES
VIDEO
COMMENTS
Data Science-Related Research Topics. Developing machine learning models for real-time fraud detection in online transactions. The use of big data analytics in predicting and managing urban traffic flow. Investigating the effectiveness of data mining techniques in identifying early signs of mental health issues from social media usage.
The data science landscape changes rapidly, and new techniques and tools are constantly being developed. To keep up with the competition, you need to be aware of the latest trends and topics in data science research. In this article, we will provide an overview of 37 hot research topics in data science.
A Master's in Data Science is a graduate degree between a bachelor's and PhD, which usually takes between one and two years to complete. A master's degree expands on what was learned in undergraduate school through more advanced courses in topics such as machine learning, data analytics, and statistics.
A PhD is the most advanced data science degree you can get, reflecting a depth of knowledge and technical expertise that will put you at the top of your field. ... Students complete 45 credits of electives, which are personalized to match the specific interests and research topics of the student. Finally, students complete up to 12 credits in ...
The field of data science has emerged from advances in computational speed, data availability, and novel analysis methods. It demands a new type of researcher: the rigorously trained, cross-disciplinary, and ethically responsible data scientist. Launched in Fall 2017, the pioneering CDS PhD Data Science program seeks to produce such researchers.
As a PhD student in Data Science, you will learn from faculty who have developed research programs that span a wide variety of data science and AI topics, from theory to applications, with a focus on making a societal impact. Research Topics: Artificial Intelligence; Data, AI, and Society; Data Systems; Human-Centered Data Science
The goal of the preliminary assessment examination is to assess students' preparation for pursuing a PhD in data science, in terms of core knowledge and readiness for conducting research. ... The final form of the dissertation document must comply with published guidelines by the Graduate Division. The dissertation topic will be selected by ...
The PhD-DS degree prepares you to conduct research in data science by exploring each stage of the data science life cycle from both a theoretical and applied perspective. You'll explore a broad range of related topics, including data mining, big data integration, business intelligence, data visualization, critical analysis, and strategic data ...
The Department requires that students in the Statistics and Data Science PhD program: Meet the department minimum residency requirement of 2 years; Complete the following courses: ... This comprehensive examination covers basic topics in statistics and data science and and is typically taken in fall quarter of the second year.
PhD in Data Science: A PhD in data science emphasizes on conducting deep research on a particular specialized topic. Normally, it takes four to five years to finish, though it often takes longer based on a variety of individual reasons.