- Number System and Arithmetic
- Probability
- Mensuration
- Trigonometry
- Mathematics

Type I and Type II Errors
Type I and Type II Errors are central for hypothesis testing in general, which subsequently impacts various aspects of science including but not limited to statistical analysis. False discovery refers to a Type I error where a true Null Hypothesis is incorrectly rejected. On the other end of the spectrum, Type II errors occur when a true null hypothesis fails to get rejected.
In this article, we will discuss Type I and Type II Errors in detail, including examples and differences.
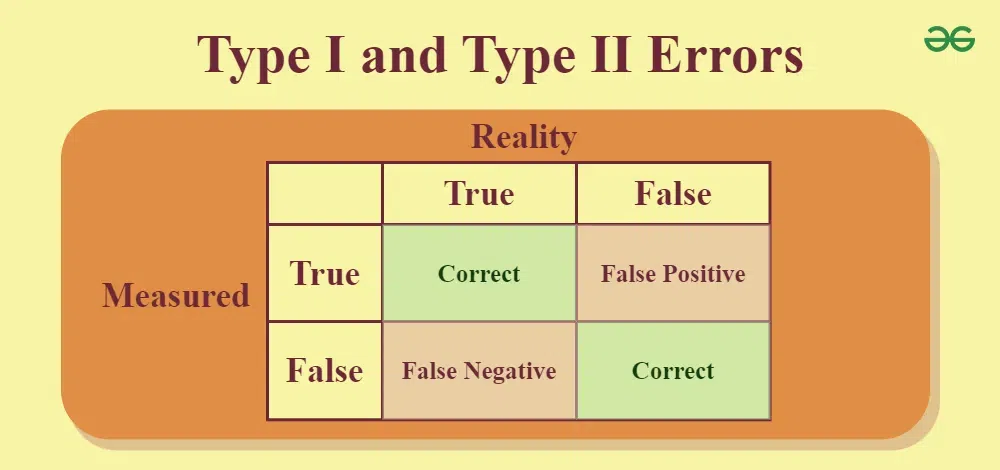
Table of Content
Type I and Type II Error in Statistics
What is error, what is type i error (false positive), what is type ii error (false negative), type i and type ii errors - table, type i and type ii errors examples, examples of type i error, examples of type ii error, factors affecting type i and type ii errors, how to minimize type i and type ii errors, difference between type i and type ii errors.
In statistics , Type I and Type II errors represent two kinds of errors that can occur when making a decision about a hypothesis based on sample data. Understanding these errors is crucial for interpreting the results of hypothesis tests.
In the statistics and hypothesis testing , an error refers to the emergence of discrepancies between the result value based on observation or calculation and the actual value or expected value.
The failures may happen in different factors, such as turbulent sampling, unclear implementation, or faulty assumptions. Errors can be of many types, such as
- Measurement Error
- Calculation Error
- Human Error
- Systematic Error
- Random Error
In hypothesis testing, it is often clear which kind of error is the problem, either a Type I error or a Type II one.
Type I error, also known as a false positive , occurs in statistical hypothesis testing when a null hypothesis that is actually true is rejected. In other words, it's the error of incorrectly concluding that there is a significant effect or difference when there isn't one in reality.
In hypothesis testing, there are two competing hypotheses:
- Null Hypothesis (H 0 ): This hypothesis represents a default assumption that there is no effect, no difference, or no relationship in the population being studied.
- Alternative Hypothesis (H 1 ): This hypothesis represents the opposite of the null hypothesis. It suggests that there is a significant effect, difference, or relationship in the population.
A Type I error occurs when the null hypothesis is rejected based on the sample data, even though it is actually true in the population.
Type II error, also known as a false negative , occurs in statistical hypothesis testing when a null hypothesis that is actually false is not rejected. In other words, it's the error of failing to detect a significant effect or difference when one exists in reality.
A Type II error occurs when the null hypothesis is not rejected based on the sample data, even though it is actually false in the population. In other words, it's a failure to recognize a real effect or difference.
Suppose a medical researcher is testing a new drug to see if it's effective in treating a certain condition. The null hypothesis (H 0 ) states that the drug has no effect, while the alternative hypothesis (H 1 ) suggests that the drug is effective. If the researcher conducts a statistical test and fails to reject the null hypothesis (H 0 ), concluding that the drug is not effective, when in fact it does have an effect, this would be a Type II error.
The table given below shows the relationship between True and False:
Some of examples of type I error include:
- Medical Testing : Suppose a medical test is designed to diagnose a particular disease. The null hypothesis ( H 0 ) is that the person does not have the disease, and the alternative hypothesis ( H 1 ) is that the person does have the disease. A Type I error occurs if the test incorrectly indicates that a person has the disease (rejects the null hypothesis) when they do not actually have it.
- Legal System : In a criminal trial, the null hypothesis ( H 0 ) is that the defendant is innocent, while the alternative hypothesis ( H 1 ) is that the defendant is guilty. A Type I error occurs if the jury convicts the defendant (rejects the null hypothesis) when they are actually innocent.
- Quality Control : In manufacturing, quality control inspectors may test products to ensure they meet certain specifications. The null hypothesis ( H 0 ) is that the product meets the required standard, while the alternative hypothesis ( H 1 ) is that the product does not meet the standard. A Type I error occurs if a product is rejected (null hypothesis is rejected) as defective when it actually meets the required standard.
Using the same H 0 and H 1 , some examples of type II error include:
- Medical Testing : In a medical test designed to diagnose a disease, a Type II error occurs if the test incorrectly indicates that a person does not have the disease (fails to reject the null hypothesis) when they actually do have it.
- Legal System : In a criminal trial, a Type II error occurs if the jury acquits the defendant (fails to reject the null hypothesis) when they are actually guilty.
- Quality Control : In manufacturing, a Type II error occurs if a defective product is accepted (fails to reject the null hypothesis) as meeting the required standard.
Some of the common factors affecting errors are:
- Sample Size: In statistical hypothesis testing, larger sample sizes generally reduce the probability of both Type I and Type II errors. With larger samples, the estimates tend to be more precise, resulting in more accurate conclusions.
- Significance Level: The significance level (α) in hypothesis testing determines the probability of committing a Type I error. Choosing a lower significance level reduces the risk of Type I error but increases the risk of Type II error, and vice versa.
- Effect Size: The magnitude of the effect or difference being tested influences the probability of Type II error. Smaller effect sizes are more challenging to detect, increasing the likelihood of failing to reject the null hypothesis when it's false.
- Statistical Power: The power of Statistics (1 – β) dictates that the opportunity for rejecting a wrong null hypothesis is based on the inverse of the chance of committing a Type II error. The power level of the test rises, thus a chance of the Type II error dropping.
To minimize Type I and Type II errors in hypothesis testing, there are several strategies that can be employed based on the information from the sources provided:
- By setting a lower significance level, the chances of incorrectly rejecting the null hypothesis decrease, thus minimizing Type I errors.
- Increasing the sample size reduces the variability of the statistic, making it less likely to fall in the non-rejection region when it should be rejected, thus minimizing Type II errors.
Some of the key differences between Type I and Type II Errors are listed in the following table:
Conclusion - Type I and Type II Errors
In conclusion, type I errors occur when we mistakenly reject a true null hypothesis, while Type II errors happen when we fail to reject a false null hypothesis. Being aware of these errors helps us make more informed decisions, minimizing the risks of false conclusions.
People Also Read:
Difference between Null and Alternate Hypothesis Z-Score Table
Type I and Type II Errors - FAQs
What is type i error.
Type I Error occurs when a null hypothesis is incorrectly rejected, indicating a false positive result, concluding that there is an effect or difference when there isn't one.
What is an Example of a Type 1 Error?
An example of Type I Error is that convicting an innocent person (null hypothesis: innocence) based on insufficient evidence, incorrectly rejecting the null hypothesis of innocence.
What is Type II Error?
Type II Error happens when a null hypothesis is incorrectly accepted, failing to detect a true effect or difference when one actually exists.
What is an Example of a Type 2 Error?
An example of type 2 error is that failing to diagnose a disease in a patient (null hypothesis: absence of disease) despite them actually having the disease, incorrectly failing to reject the null hypothesis.
What is the difference between Type 1 and Type 2 Errors?
Type I error involves incorrectly rejecting a true null hypothesis, while Type II error involves failing to reject a false null hypothesis. In simpler terms, Type I error is a false positive, while Type II error is a false negative.

What is Type 3 Error?
Type 3 Error is not a standard statistical term. It's sometimes informally used to describe situations where the researcher correctly rejects the null hypothesis but for the wrong reason, often due to a flaw in the experimental design or analysis.
How are Type I and Type II Errors related to hypothesis testing?
In hypothesis testing, Type I Error relates to the significance level (α), which represents the probability of rejecting a true null hypothesis. Type II Error relates to the power of the test (β), which represents the probability of failing to reject a false null hypothesis.
What are some examples of Type I and Type II Errors?
Type I Error: Rejecting a null hypothesis that a new drug has no side effects when it actually does (false positive). Type II Error: Failing to reject a null hypothesis that a new drug has no effect when it actually does (false negative).
How can one minimize Type I and Type II Errors?
Type I Error can be minimized by choosing a lower significance level (α) for hypothesis testing. Type II Error can be minimized by increasing the sample size or improving the sensitivity of the test.
What is the relationship between Type I and Type II Errors?
There is often a trade-off between Type I and Type II Errors. Decreasing the probability of one type of error typically increases the probability of the other.
How do Type I and Type II Errors impact decision-making?
Type I Errors can lead to false conclusions, such as mistakenly believing a treatment is effective when it's not. Type II Errors can result in missed opportunities, such as failing to identify an effective treatment.
In which fields are Type I and Type II Errors commonly encountered?
Type I and Type II Errors are encountered in various fields, including medical research, quality control, criminal justice, and market research.
Similar Reads
- Type I and Type II Errors Type I and Type II Errors are central for hypothesis testing in general, which subsequently impacts various aspects of science including but not limited to statistical analysis. False discovery refers to a Type I error where a true Null Hypothesis is incorrectly rejected. On the other end of the spe 10 min read
- Type I Error in R In statistical hypothesis testing, a Type I error occurs when the null hypothesis is incorrectly rejected when it is true. This error, often denoted by the Greek letter alpha (α), is a critical concept in the fields of statistics, data analysis, and scientific research. Understanding Type I errors i 6 min read
- Top 10 errors in R and how to fix them R is a powerful language for statistical computing and graphics, but like any programming language, it comes with its own set of common errors that can trip up both novice and experienced users. Understanding these errors and knowing how to fix them can save a lot of time and frustration. Here are t 5 min read
- Explain Type assertions in TypeScript In Typescript, Type assertion is a technique that informs the compiler about the type of a variable. Type assertion is similar to typecasting but it doesn't reconstruct code. You can use type assertion to specify a value's type and tell the compiler not to deduce it. When we want to change a variabl 3 min read
- TypeScript Assertions Type TypeScript Assertions Type, also known as Type Assertion, is a feature that lets developers manually override the inferred or expected type of a value, providing more control over type checking in situations where TypeScript's automatic type inference may not be sufficient. Syntaxlet variableName: A 2 min read
- Address Errors in 'if' Statements in R Error handling is super important when writing code, no matter which language are using even in R Programming Language. Errors can happen for all sorts of reasons, like when a user gives the computer the wrong kind of information, something happens that the user didn't expect, or the user just makes 5 min read
- TypeScript Less Common Primitives Type TypeScript Less Common Primitives Type offers a rich set of primitive types to represent data. While most developers are familiar with types like number, string, boolean, and symbol, TypeScript also provides less common primitive types that can be incredibly useful in specific scenarios. These are s 2 min read
- Recursive Type Guards In TypeScript In TypeScript type guards help determine the type of a variable at runtime, they are especially useful when dealing with complex types like unions, discriminated unions or even recursive structures and a recursive type guard is a type guard function that can handle complex nested types, including th 6 min read
- How to use Type Guards in TypeScript ? Type Guards in TypeScript are features that allow developers to narrow the type of a variable within conditional blocks. They enhance type safety by checking variable types using typeof, instanceof, or custom type guard functions, ensuring accurate type inference and preventing runtime errors. Type 4 min read
- TypeScript Narrowing typeof type guards In this article, we are going to learn about Narrowing typeof type guards. TypeScript is a popular programming language used for building scalable and robust applications. In TypeScript, the typeof type guard allows you to narrow down a variable's type based on the result of the typeof operator. Thi 3 min read
- TypeScript Truthiness Narrowing Type In this article, we are going to learn about Truthiness narrowing Type in Typescript. TypeScript is a popular programming language used for building scalable and robust applications. In TypeScript, truthiness narrowing is a concept that allows you to narrow down the type of a variable based on its t 3 min read
- Rust - Multiple Error Types In Rust, sometimes we have scenarios where there is more than one error type. In Rust, the way of handling errors is different compared to other Object Oriented / System languages. Rust specifically uses 'Result' for returning something. The Result <T, E> is basically an enum that has - Ok(T) 3 min read
- How to Fix Error in colMeans in R R Programming Language is widely used for statistical computing and data analysis. Like any other programming language, R users often encounter errors while working with functions. One common function that users may encounter errors with is colMeans, which is used to calculate column-wise means in m 5 min read
- TypeScript any Type In TypeScript, any type is a dynamic type that can represent values of any data type. It allows for flexible typing but sacrifices type safety, as it lacks compile-time type checking, making it less recommended in strongly typed TypeScript code. It allows developers to specify types for variables, f 4 min read
- TypeScript Interfaces Type TypeScript Interfaces Type offers an alternative method for defining an object's type, allowing for a distinct naming approach. Syntax:interface InterfaceName { property1: type1; property2?: type2; readonly property3: type3; // ... method1(): returnType1; method2(): returnType2; // ...}Parameters:in 2 min read
- Error Handling with the Either type in TypeScript Error handling with the Either type in TypeScript allow a user to manage computations that can result in either a success (Right) or a failure (Left), allowing functions to explicitly handle both outcomes. This pattern helps in avoiding exceptions and promotes explicit handling of both successful an 3 min read
- TypeScript Equality Narrowing Type In this article, we are going to learn about Equality narrowing Type in Typescript. TypeScript is a popular programming language used for building scalable and robust applications. In TypeScript, "equality narrowing" refers to the process of narrowing the type of a variable based on equality checks 4 min read
- Implicit and Explicit type casting In programming, type casting is a way to convert data from one type to another. Implicit type casting happens automatically, while explicit type casting requires manual intervention. This article explores the differences between implicit and explicit type casting, their uses, benefits, and considera 6 min read
- TypeScript Conditional Types TypeScript's Conditional Types enable types to be defined based on a condition. Using the syntax T extends U ? X: Y, the type evaluates to X if T extends U; otherwise, it evaluates to Y. This feature allows for more flexible and dynamic type definitions. Syntaxtype ConditionalType<T> = T exten 6 min read
- How to Check Types in Typescript? Checking types in TypeScript involves methods like typeof for primitive types, instanceof for class instances, and custom type guards for complex type validation. These techniques help ensure variables are correctly typed, improving code safety, and readability, and preventing runtime errors.Here ar 3 min read
Improve your Coding Skills with Practice
What kind of Experience do you want to share?

IMAGES
VIDEO