- Privacy Policy

Home » Factor Analysis – Steps, Methods and Examples

Factor Analysis – Steps, Methods and Examples
Table of Contents

Factor Analysis
Definition:
Factor analysis is a statistical technique that is used to identify the underlying structure of a relatively large set of variables and to explain these variables in terms of a smaller number of common underlying factors. It helps to investigate the latent relationships between observed variables.
Factor Analysis Steps
Here are the general steps involved in conducting a factor analysis:
1. Define the Research Objective:
Clearly specify the purpose of the factor analysis. Determine what you aim to achieve or understand through the analysis.
2. Data Collection:
Gather the data on the variables of interest. These variables should be measurable and related to the research objective. Ensure that you have a sufficient sample size for reliable results.
3. Assess Data Suitability:
Examine the suitability of the data for factor analysis. Check for the following aspects:
- Sample size: Ensure that you have an adequate sample size to perform factor analysis reliably.
- Missing values: Handle missing data appropriately, either by imputation or exclusion.
- Variable characteristics: Verify that the variables are continuous or at least ordinal in nature. Categorical variables may require different analysis techniques.
- Linearity: Assess whether the relationships among variables are linear.
4. Determine the Factor Analysis Technique:
There are different types of factor analysis techniques available, such as exploratory factor analysis (EFA) and confirmatory factor analysis (CFA). Choose the appropriate technique based on your research objective and the nature of the data.
5. Perform Factor Analysis:
a. Exploratory Factor Analysis (EFA):
- Extract factors: Use factor extraction methods (e.g., principal component analysis or common factor analysis) to identify the initial set of factors.
- Determine the number of factors: Decide on the number of factors to retain based on statistical criteria (e.g., eigenvalues, scree plot) and theoretical considerations.
- Rotate factors: Apply factor rotation techniques (e.g., varimax, oblique) to simplify the factor structure and make it more interpretable.
- Interpret factors: Analyze the factor loadings (correlations between variables and factors) to interpret the meaning of each factor.
- Determine factor reliability: Assess the internal consistency or reliability of the factors using measures like Cronbach’s alpha.
- Report results: Document the factor loadings, rotated component matrix, communalities, and any other relevant information.
b. Confirmatory Factor Analysis (CFA):
- Formulate a theoretical model: Specify the hypothesized relationships among variables and factors based on prior knowledge or theoretical considerations.
- Define measurement model: Establish how each variable is related to the underlying factors by assigning factor loadings in the model.
- Test the model: Use statistical techniques like maximum likelihood estimation or structural equation modeling to assess the goodness-of-fit between the observed data and the hypothesized model.
- Modify the model: If the initial model does not fit the data adequately, revise the model by adding or removing paths, allowing for correlated errors, or other modifications to improve model fit.
- Report results: Present the final measurement model, parameter estimates, fit indices (e.g., chi-square, RMSEA, CFI), and any modifications made.
6. Interpret and Validate the Factors:
Once you have identified the factors, interpret them based on the factor loadings, theoretical understanding, and research objectives. Validate the factors by examining their relationships with external criteria or by conducting further analyses if necessary.
Types of Factor Analysis
Types of Factor Analysis are as follows:
Exploratory Factor Analysis (EFA)
EFA is used to explore the underlying structure of a set of observed variables without any preconceived assumptions about the number or nature of the factors. It aims to discover the number of factors and how the observed variables are related to those factors. EFA does not impose any restrictions on the factor structure and allows for cross-loadings of variables on multiple factors.
Confirmatory Factor Analysis (CFA)
CFA is used to test a pre-specified factor structure based on theoretical or conceptual assumptions. It aims to confirm whether the observed variables measure the latent factors as intended. CFA tests the fit of a hypothesized model and assesses how well the observed variables are associated with the expected factors. It is often used for validating measurement instruments or evaluating theoretical models.
Principal Component Analysis (PCA)
PCA is a dimensionality reduction technique that can be considered a form of factor analysis, although it has some differences. PCA aims to explain the maximum amount of variance in the observed variables using a smaller number of uncorrelated components. Unlike traditional factor analysis, PCA does not assume that the observed variables are caused by underlying factors but focuses solely on accounting for variance.
Common Factor Analysis
It assumes that the observed variables are influenced by common factors and unique factors (specific to each variable). It attempts to estimate the common factor structure by extracting the shared variance among the variables while also considering the unique variance of each variable.
Hierarchical Factor Analysis
Hierarchical factor analysis involves multiple levels of factors. It explores both higher-order and lower-order factors, aiming to capture the complex relationships among variables. Higher-order factors are based on the relationships among lower-order factors, which are in turn based on the relationships among observed variables.
Factor Analysis Formulas
Factor Analysis is a statistical method used to describe variability among observed, correlated variables in terms of a potentially lower number of unobserved variables called factors.
Here are some of the essential formulas and calculations used in factor analysis:
Correlation Matrix :
The first step in factor analysis is to create a correlation matrix, which calculates the correlation coefficients between pairs of variables.
Correlation coefficient (Pearson’s r) between variables X and Y is calculated as:
r(X,Y) = Σ[(xi – x̄)(yi – ȳ)] / [n-1] σx σy
where: xi, yi are the data points, x̄, ȳ are the means of X and Y respectively, σx, σy are the standard deviations of X and Y respectively, n is the number of data points.
Extraction of Factors :
The extraction of factors from the correlation matrix is typically done by methods such as Principal Component Analysis (PCA) or other similar methods.
The formula used in PCA to calculate the principal components (factors) involves finding the eigenvalues and eigenvectors of the correlation matrix.
Let’s denote the correlation matrix as R. If λ is an eigenvalue of R, and v is the corresponding eigenvector, they satisfy the equation: Rv = λv
Factor Loadings :
Factor loadings are the correlations between the original variables and the factors. They can be calculated as the eigenvectors normalized by the square roots of their corresponding eigenvalues.
Communality and Specific Variance :
Communality of a variable is the proportion of variance in that variable explained by the factors. It can be calculated as the sum of squared factor loadings for that variable across all factors.
The specific variance of a variable is the proportion of variance in that variable not explained by the factors, and it’s calculated as 1 – Communality.
Factor Rotation : Factor rotation, such as Varimax or Promax, is used to make the output more interpretable. It doesn’t change the underlying relationships but affects the loadings of the variables on the factors.
For example, in the Varimax rotation, the objective is to minimize the variance of the squared loadings of a factor (column) on all the variables (rows) in a factor matrix, which leads to more high and low loadings, making the factor easier to interpret.
Examples of Factor Analysis
Here are some real-time examples of factor analysis:
- Psychological Research: In a study examining personality traits, researchers may use factor analysis to identify the underlying dimensions of personality by analyzing responses to various questionnaires or surveys. Factors such as extroversion, neuroticism, and conscientiousness can be derived from the analysis.
- Market Research: In marketing, factor analysis can be used to understand consumers’ preferences and behaviors. For instance, by analyzing survey data related to product features, pricing, and brand perception, researchers can identify factors such as price sensitivity, brand loyalty, and product quality that influence consumer decision-making.
- Finance and Economics: Factor analysis is widely used in portfolio management and asset pricing models. By analyzing historical market data, factors such as market returns, interest rates, inflation rates, and other economic indicators can be identified. These factors help in understanding and predicting investment returns and risk.
- Social Sciences: Factor analysis is employed in social sciences to explore underlying constructs in complex datasets. For example, in education research, factor analysis can be used to identify dimensions such as academic achievement, socio-economic status, and parental involvement that contribute to student success.
- Health Sciences: In medical research, factor analysis can be utilized to identify underlying factors related to health conditions, symptom clusters, or treatment outcomes. For instance, in a study on mental health, factor analysis can be used to identify underlying factors contributing to depression, anxiety, and stress.
- Customer Satisfaction Surveys: Factor analysis can help businesses understand the key drivers of customer satisfaction. By analyzing survey responses related to various aspects of product or service experience, factors such as product quality, customer service, and pricing can be identified, enabling businesses to focus on areas that impact customer satisfaction the most.
Factor analysis in Research Example
Here’s an example of how factor analysis might be used in research:
Let’s say a psychologist is interested in the factors that contribute to overall wellbeing. They conduct a survey with 1000 participants, asking them to respond to 50 different questions relating to various aspects of their lives, including social relationships, physical health, mental health, job satisfaction, financial security, personal growth, and leisure activities.
Given the broad scope of these questions, the psychologist decides to use factor analysis to identify underlying factors that could explain the correlations among responses.
After conducting the factor analysis, the psychologist finds that the responses can be grouped into five factors:
- Physical Wellbeing : Includes variables related to physical health, exercise, and diet.
- Mental Wellbeing : Includes variables related to mental health, stress levels, and emotional balance.
- Social Wellbeing : Includes variables related to social relationships, community involvement, and support from friends and family.
- Professional Wellbeing : Includes variables related to job satisfaction, work-life balance, and career development.
- Financial Wellbeing : Includes variables related to financial security, savings, and income.
By reducing the 50 individual questions to five underlying factors, the psychologist can more effectively analyze the data and draw conclusions about the major aspects of life that contribute to overall wellbeing.
In this way, factor analysis helps researchers understand complex relationships among many variables by grouping them into a smaller number of factors, simplifying the data analysis process, and facilitating the identification of patterns or structures within the data.
When to Use Factor Analysis
Here are some circumstances in which you might want to use factor analysis:
- Data Reduction : If you have a large set of variables, you can use factor analysis to reduce them to a smaller set of factors. This helps in simplifying the data and making it easier to analyze.
- Identification of Underlying Structures : Factor analysis can be used to identify underlying structures in a dataset that are not immediately apparent. This can help you understand complex relationships between variables.
- Validation of Constructs : Factor analysis can be used to confirm whether a scale or measure truly reflects the construct it’s meant to measure. If all the items in a scale load highly on a single factor, that supports the construct validity of the scale.
- Generating Hypotheses : By revealing the underlying structure of your variables, factor analysis can help to generate hypotheses for future research.
- Survey Analysis : If you have a survey with many questions, factor analysis can help determine if there are underlying factors that explain response patterns.
Applications of Factor Analysis
Factor Analysis has a wide range of applications across various fields. Here are some of them:
- Psychology : It’s often used in psychology to identify the underlying factors that explain different patterns of correlations among mental abilities. For instance, factor analysis has been used to identify personality traits (like the Big Five personality traits), intelligence structures (like Spearman’s g), or to validate the constructs of different psychological tests.
- Market Research : In this field, factor analysis is used to identify the factors that influence purchasing behavior. By understanding these factors, businesses can tailor their products and marketing strategies to meet the needs of different customer groups.
- Healthcare : In healthcare, factor analysis is used in a similar way to psychology, identifying underlying factors that might influence health outcomes. For instance, it could be used to identify lifestyle or behavioral factors that influence the risk of developing certain diseases.
- Sociology : Sociologists use factor analysis to understand the structure of attitudes, beliefs, and behaviors in populations. For example, factor analysis might be used to understand the factors that contribute to social inequality.
- Finance and Economics : In finance, factor analysis is used to identify the factors that drive financial markets or economic behavior. For instance, factor analysis can help understand the factors that influence stock prices or economic growth.
- Education : In education, factor analysis is used to identify the factors that influence academic performance or attitudes towards learning. This could help in developing more effective teaching strategies.
- Survey Analysis : Factor analysis is often used in survey research to reduce the number of items or to identify the underlying structure of the data.
- Environment : In environmental studies, factor analysis can be used to identify the major sources of environmental pollution by analyzing the data on pollutants.
Advantages of Factor Analysis
Advantages of Factor Analysis are as follows:
- Data Reduction : Factor analysis can simplify a large dataset by reducing the number of variables. This helps make the data easier to manage and analyze.
- Structure Identification : It can identify underlying structures or patterns in a dataset that are not immediately apparent. This can provide insights into complex relationships between variables.
- Construct Validation : Factor analysis can be used to validate whether a scale or measure accurately reflects the construct it’s intended to measure. This is important for ensuring the reliability and validity of measurement tools.
- Hypothesis Generation : By revealing the underlying structure of your variables, factor analysis can help generate hypotheses for future research.
- Versatility : Factor analysis can be used in various fields, including psychology, market research, healthcare, sociology, finance, education, and environmental studies.
Disadvantages of Factor Analysis
Disadvantages of Factor Analysis are as follows:
- Subjectivity : The interpretation of the factors can sometimes be subjective, depending on how the data is perceived. Different researchers might interpret the factors differently, which can lead to different conclusions.
- Assumptions : Factor analysis assumes that there’s some underlying structure in the dataset and that all variables are related. If these assumptions do not hold, factor analysis might not be the best tool for your analysis.
- Large Sample Size Required : Factor analysis generally requires a large sample size to produce reliable results. This can be a limitation in studies where data collection is challenging or expensive.
- Correlation, not Causation : Factor analysis identifies correlational relationships, not causal ones. It cannot prove that changes in one variable cause changes in another.
- Complexity : The statistical concepts behind factor analysis can be difficult to understand and require expertise to implement correctly. Misuse or misunderstanding of the method can lead to incorrect conclusions.
About the author
Muhammad Hassan
Researcher, Academic Writer, Web developer
You may also like

Discriminant Analysis – Methods, Types and...

Histogram – Types, Examples and Making Guide

Graphical Methods – Types, Examples and Guide

Substantive Framework – Types, Methods and...

Uniform Histogram – Purpose, Examples and Guide

Correlation Analysis – Types, Methods and...
Root out friction in every digital experience, super-charge conversion rates, and optimise digital self-service
Uncover insights from any interaction, deliver AI-powered agent coaching, and reduce cost to serve
Increase revenue and loyalty with real-time insights and recommendations delivered straight to teams on the ground
Know how your people feel and empower managers to improve employee engagement, productivity, and retention
Take action in the moments that matter most along the employee journey and drive bottom line growth
Whatever they’re saying, wherever they’re saying it, know exactly what’s going on with your people
Get faster, richer insights with qual and quant tools that make powerful market research available to everyone
Run concept tests, pricing studies, prototyping + more with fast, powerful studies designed by UX research experts
Track your brand performance 24/7 and act quickly to respond to opportunities and challenges in your market
Meet the operating system for experience management
- Free Account
- Product Demos
- For Digital
- For Customer Care
- For Human Resources
- For Researchers
- Financial Services
- All Industries
Popular Use Cases
- Customer Experience
- Employee Experience
- Employee Exit Interviews
- Net Promoter Score
- Voice of Customer
- Customer Success Hub
- Product Documentation
- Training & Certification
- XM Institute
- Popular Resources
- Customer Stories
- Artificial Intelligence
- Market Research
- Partnerships
- Marketplace
The annual gathering of the experience leaders at the world’s iconic brands building breakthrough business results.
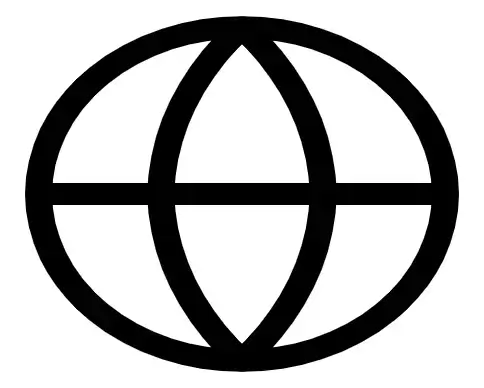
- English/AU & NZ
- Español/Europa
- Español/América Latina
- Português Brasileiro
- REQUEST DEMO
- Experience Management
- Survey Analysis
- Factor Analysis
Try Qualtrics for free
What is factor analysis and how does it simplify research findings.
17 min read There are many forms of data analysis used to report on and study survey data. Factor analysis is best when used to simplify complex data sets with many variables.
What is factor analysis?
Factor analysis is the practice of condensing many variables into just a few, so that your research data is easier to work with.
For example, a retail business trying to understand customer buying behaviours might consider variables such as ‘did the product meet your expectations?’, ‘how would you rate the value for money?’ and ‘did you find the product easily?’. Factor analysis can help condense these variables into a single factor, such as ‘customer purchase satisfaction’.
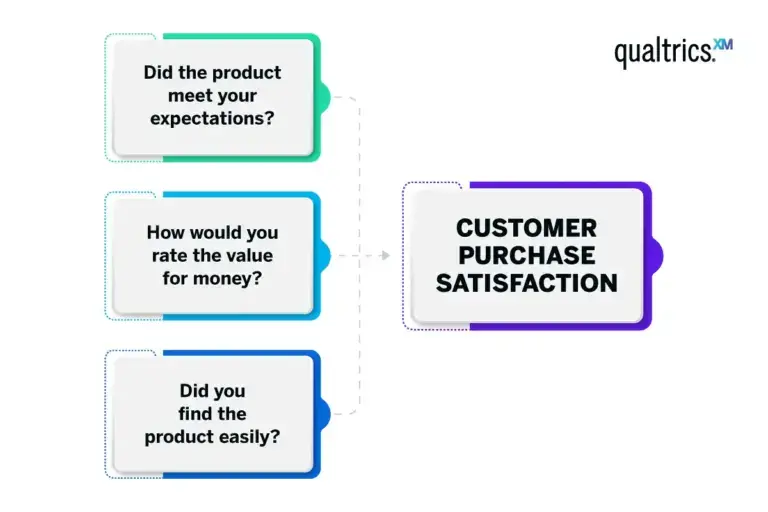
The theory is that there are deeper factors driving the underlying concepts in your data, and that you can uncover and work with them instead of dealing with the lower-level variables that cascade from them. Know that these deeper concepts aren’t necessarily immediately obvious – they might represent traits or tendencies that are hard to measure, such as extraversion or IQ.
Factor analysis is also sometimes called “dimension reduction”: you can reduce the “dimensions” of your data into one or more “super-variables,” also known as unobserved variables or latent variables. This process involves creating a factor model and often yields a factor matrix that organises the relationship between observed variables and the factors they’re associated with.
As with any kind of process that simplifies complexity, there is a trade-off between the accuracy of the data and how easy it is to work with. With factor analysis, the best solution is the one that yields a simplification that represents the true nature of your data, with minimum loss of precision. This often means finding a balance between achieving the variance explained by the model and using fewer factors to keep the model simple.
Factor analysis isn’t a single technique, but a family of statistical methods that can be used to identify the latent factors driving observable variables. Factor analysis is commonly used in market research , as well as other disciplines like technology, medicine, sociology, field biology, education, psychology and many more.
What is a factor?
In the context of factor analysis, a factor is a hidden or underlying variable that we infer from a set of directly measurable variables.
Take ‘customer purchase satisfaction’ as an example again. This isn’t a variable you can directly ask a customer to rate, but it can be determined from the responses to correlated questions like ‘did the product meet your expectations?’, ‘how would you rate the value for money?’ and ‘did you find the product easily?’.
While not directly observable, factors are essential for providing a clearer, more streamlined understanding of data. They enable us to capture the essence of our data’s complexity, making it simpler and more manageable to work with, and without losing lots of information.
Free eBook: 2024 global market research trends report
Key concepts in factor analysis
These concepts are the foundational pillars that guide the application and interpretation of factor analysis.
Central to factor analysis, variance measures how much numerical values differ from the average. In factor analysis, you’re essentially trying to understand how underlying factors influence this variance among your variables. Some factors will explain more variance than others, meaning they more accurately represent the variables they consist of.
The eigenvalue expresses the amount of variance a factor explains. If a factor solution (unobserved or latent variables) has an eigenvalue of 1 or above, it indicates that a factor explains more variance than a single observed variable, which can be useful in reducing the number of variables in your analysis. Factors with eigenvalues less than 1 account for less variability than a single variable and are generally not included in the analysis.
Factor score
A factor score is a numeric representation that tells us how strongly each variable from the original data is related to a specific factor. Also called the component score, it can help determine which variables are most influenced by each factor and are most important for each underlying concept.
Factor loading
Factor loading is the correlation coefficient for the variable and factor. Like the factor score, factor loadings give an indication of how much of the variance in an observed variable can be explained by the factor. High factor loadings (close to 1 or -1) mean the factor strongly influences the variable.
When to use factor analysis
Factor analysis is a powerful tool when you want to simplify complex data, find hidden patterns, and set the stage for deeper, more focused analysis.
It’s typically used when you’re dealing with a large number of interconnected variables, and you want to understand the underlying structure or patterns within this data. It’s particularly useful when you suspect that these observed variables could be influenced by some hidden factors.
For example, consider a business that has collected extensive customer feedback through surveys . The survey covers a wide range of questions about product quality, pricing, customer service and more. This huge volume of data can be overwhelming, and this is where factor analysis comes in. It can help condense these numerous variables into a few meaningful factors, such as ‘product satisfaction’, ‘customer service experience’ and ‘value for money’.
Factor analysis doesn’t operate in isolation – it’s often used as a stepping stone for further analysis. For example, once you’ve identified key factors through factor analysis, you might then proceed to a cluster analysis – a method that groups your customers based on their responses to these factors. The result is a clearer understanding of different customer segments, which can then guide targeted marketing and product development strategies.
By combining factor analysis with other methodologies, you can not only make sense of your data but also gain valuable insights to drive your business decisions.
Factor analysis assumptions
Factor analysis relies on several assumptions for accurate results. Violating these assumptions may lead to factors that are hard to interpret or misleading.
Linear relationships between variables
This ensures that changes in the values of your variables are consistent.
Sufficient variables for each factor
Because if only a few variables represent a factor, it might not be identified accurately.
Adequate sample size
The larger the ratio of cases (respondents, for instance) to variables, the more reliable the analysis.
No perfect multicollinearity and singularity
No variable is a perfect linear combination of other variables, and no variable is a duplicate of another.
Relevance of the variables
There should be some correlation between variables to make a factor analysis feasible.
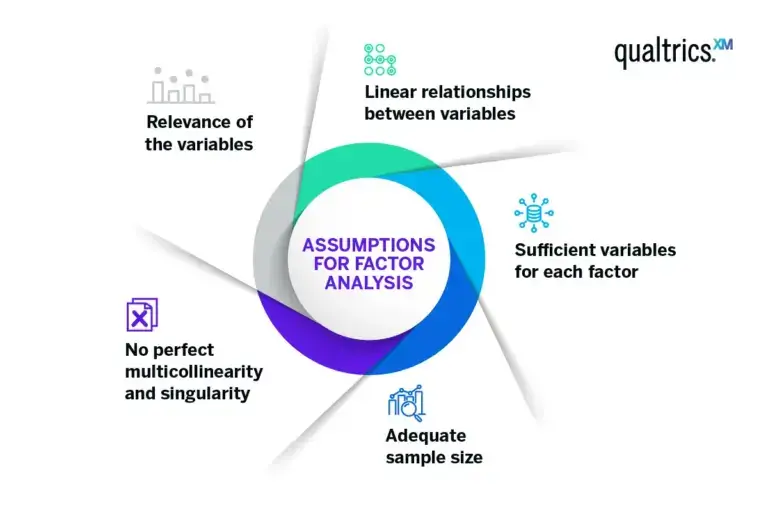
Types of factor analysis
There are two main factor analysis methods: exploratory and confirmatory. Here’s how they are used to add value to your research process.
Confirmatory factor analysis
In this type of analysis, the researcher starts out with a hypothesis about their data that they are looking to prove or disprove. Factor analysis will confirm – or not – where the latent variables are and how much variance they account for.
Principal component analysis (PCA) is a popular form of confirmatory factor analysis. Using this method, the researcher will run the analysis to obtain multiple possible solutions that split their data among a number of factors. Items that load onto a single particular factor are more strongly related to one another and can be grouped together by the researcher using their conceptual knowledge or pre-existing research.
Using PCA will generate a range of solutions with different numbers of factors, from simplified 1-factor solutions to higher levels of complexity. However, the fewer number of factors employed, the less variance will be accounted for in the solution.
Exploratory factor analysis
As the name suggests, exploratory factor analysis is undertaken without a hypothesis in mind. It’s an investigatory process that helps researchers understand whether associations exist between the initial variables, and if so, where they lie and how they are grouped.
How to perform factor analysis: A step-by-step guide
Performing a factor analysis involves a series of steps, often facilitated by statistical software packages like SPSS, Stata and the R programming language . Here’s a simplified overview of the process.
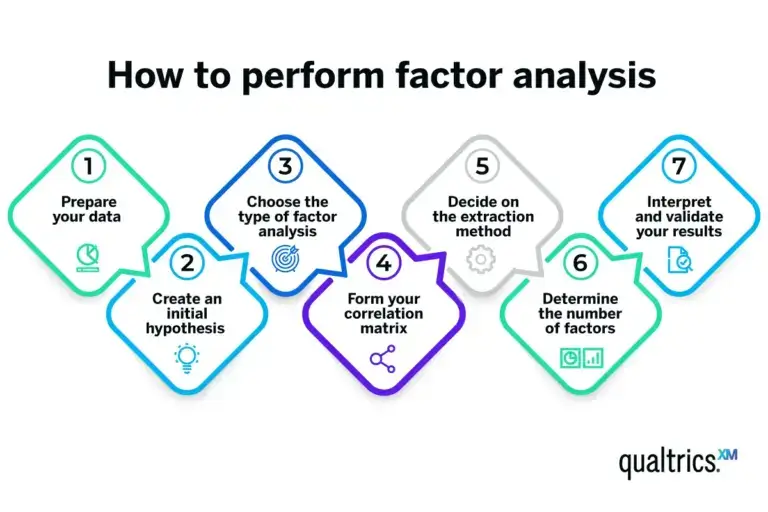
Prepare your data
Start with a dataset where each row represents a case (for example, a survey respondent), and each column is a variable you’re interested in. Ensure your data meets the assumptions necessary for factor analysis.
Create an initial hypothesis
If you have a theory about the underlying factors and their relationships with your variables, make a note of this. This hypothesis can guide your analysis, but keep in mind that the beauty of factor analysis is its ability to uncover unexpected relationships.
Choose the type of factor analysis
The most common type is exploratory factor analysis, which is used when you’re not sure what to expect. If you have a specific hypothesis about the factors, you might use confirmatory factor analysis.
Form your correlation matrix
After you’ve chosen the type of factor analysis, you’ll need to create the correlation matrix of your variables. This matrix, which shows the correlation coefficients between each pair of variables, forms the basis for the extraction of factors. This is a key step in building your factor analysis model.
Decide on the extraction method
Principal component analysis is the most commonly used extraction method. If you believe your factors are correlated, you might opt for principal axis factoring, a type of factor analysis that identifies factors based on shared variance.
Determine the number of factors
Various criteria can be used here, such as Kaiser’s criterion (eigenvalues greater than 1), the scree plot method or parallel analysis. The choice depends on your data and your goals.
Interpret and validate your results
Each factor will be associated with a set of your original variables, so label each factor based on how you interpret these associations. These labels should represent the underlying concept that ties the associated variables together.
Validation can be done through a variety of methods, like splitting your data in half and checking if both halves produce the same factors.
How factor analysis can help you
As well as giving you fewer variables to navigate, factor analysis can help you understand grouping and clustering in your input variables, since they’ll be grouped according to the latent variables.
Say you ask several questions all designed to explore different, but closely related, aspects of customer satisfaction:
- How satisfied are you with our product?
- Would you recommend our product to a friend or family member?
- How likely are you to purchase our product in the future?
But you only want one variable to represent a customer satisfaction score. One option would be to average the three question responses. Another option would be to create a factor dependent variable. This can be done by running a principal component analysis (PCA) and keeping the first principal component (also known as a factor). The advantage of a PCA over an average is that it automatically weights each of the variables in the calculation.
Say you have a list of questions and you don’t know exactly which responses will move together and which will move differently; for example, purchase barriers of potential customers. The following are possible barriers to purchase:
- Price is prohibitive
- Overall implementation costs
- We can’t reach a consensus in our organisation
- Product is not consistent with our business strategy
- I need to develop an ROI, but cannot or have not
- We are locked into a contract with another product
- The product benefits don’t outweigh the cost
- We have no reason to switch
- Our IT department cannot support your product
- We do not have sufficient technical resources
- Your product does not have a feature we require
- Other (please specify)
Factor analysis can uncover the trends of how these questions will move together. The following are loadings for 3 factors for each of the variables.
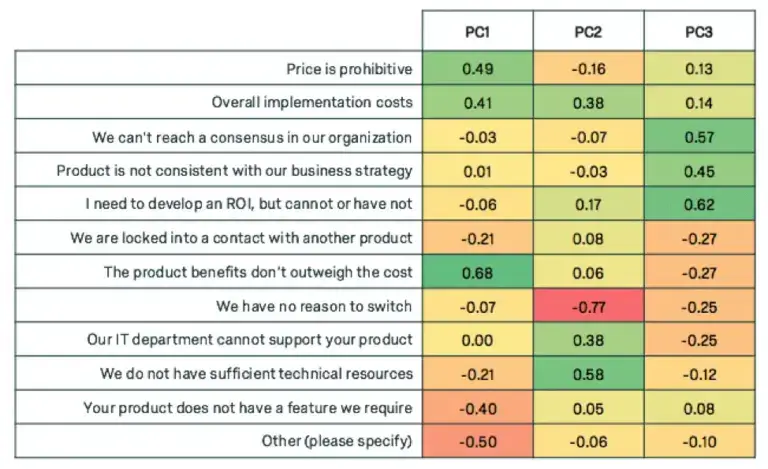
Notice how each of the principal components have high weights for a subset of the variables. Weight is used interchangeably with loading, and high weight indicates the variables that are most influential for each principal component. +0.30 is generally considered to be a heavy weight.
The first component displays heavy weights for variables related to cost, the second weights variables related to IT, and the third weights variables related to organisational factors. We can give our new super variables clever names.
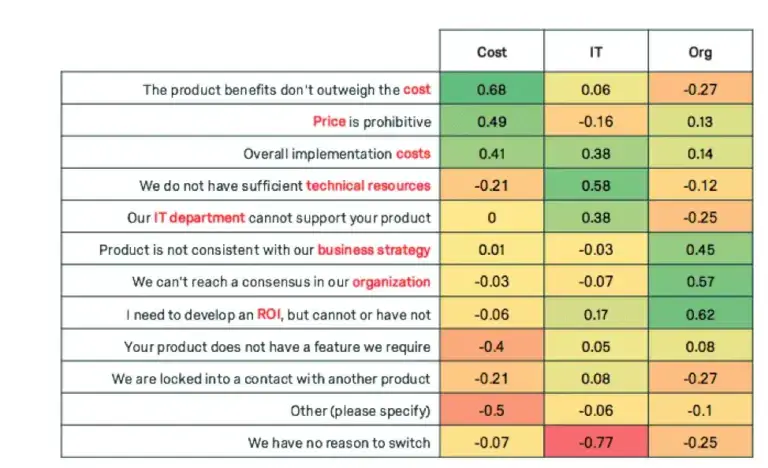
If we were to cluster the customers based on these three components, we can see some trends. Customers tend to be high in cost barriers or organisational barriers, but not both.
The red dots represent respondents who indicated they had higher organisational barriers; the green dots represent respondents who indicated they had higher cost barriers
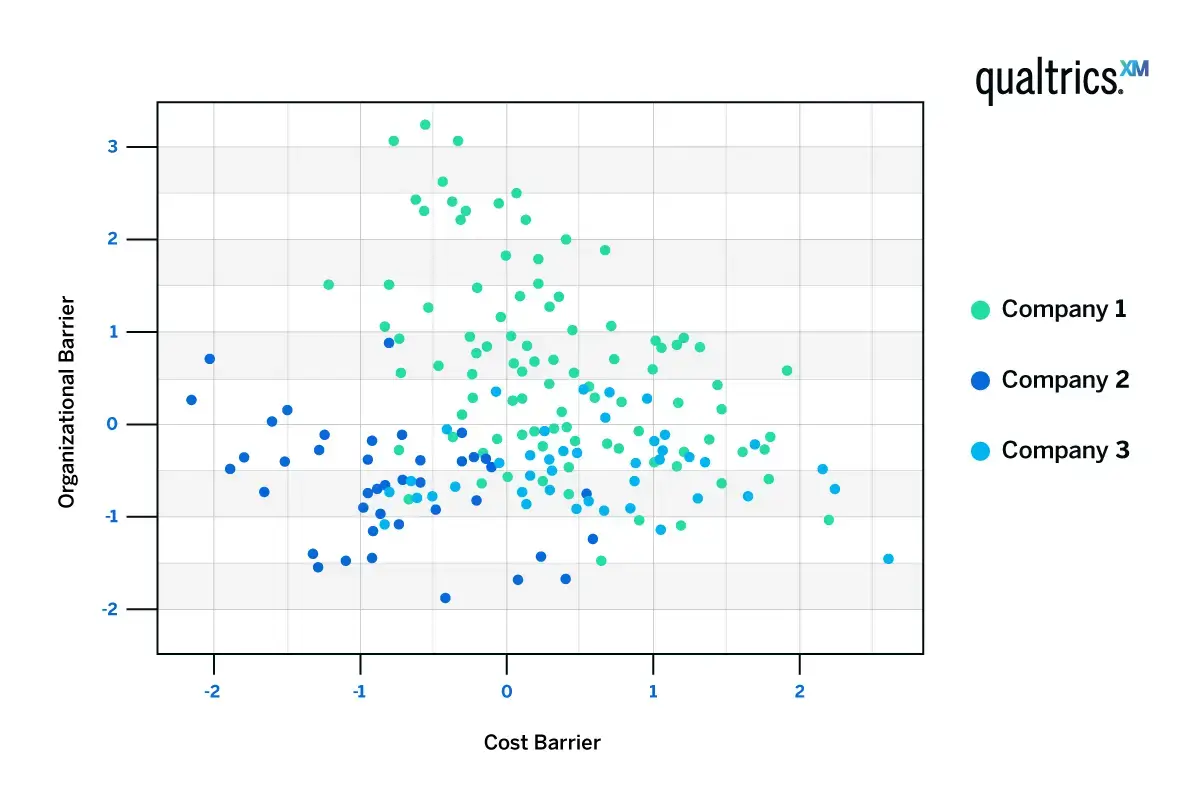
Considerations when using factor analysis
Factor analysis is a tool, and like any tool its effectiveness depends on how you use it. When employing factor analysis, it’s essential to keep a few key considerations in mind.
Oversimplification
While factor analysis is great for simplifying complex data sets, there’s a risk of oversimplification when grouping variables into factors. To avoid this you should ensure the reduced factors still accurately represent the complexities of your variables.
Subjectivity
Interpreting the factors can sometimes be subjective, and requires a good understanding of the variables and the context. Be mindful that multiple analysts may come up with different names for the same factor.
Supplementary techniques
Factor analysis is often just the first step. Consider how it fits into your broader research strategy and which other techniques you’ll use alongside it.
Examples of factor analysis studies
Factor analysis, including PCA, is often used in tandem with segmentation studies . It might be an intermediary step to reduce variables before using KMeans to make the segments.
Factor analysis provides simplicity after reducing variables. For long studies with large blocks of Matrix Likert scale questions, the number of variables can become unwieldy. Simplifying the data using factor analysis helps analysts focus and clarify the results, while also reducing the number of dimensions they’re clustering on.
Sample questions for factor analysis
Choosing exactly which questions to perform factor analysis on is both an art and a science. Choosing which variables to reduce takes some experimentation, patience and creativity. Factor analysis works well on Likert scale questions and Sum to 100 question types.
Factor analysis works well on matrix blocks of the following question genres:
Psychographics (Agree/Disagree):
- I value family
- I believe brand represents value
Behavioural (Agree/Disagree):
- I purchase the cheapest option
- I am a bargain shopper
Attitudinal (Agree/Disagree):
- The economy is not improving
- I am pleased with the product
Activity-Based (Agree/Disagree):
- I love sports
- I sometimes shop online during work hours
Behavioural and psychographic questions are especially suited for factor analysis.
Sample output reports
Factor analysis simply produces weights (called loadings) for each respondent. These loadings can be used like other responses in the survey.
Cost Barrier | IT Barrier | Org Barrier | |
---|---|---|---|
R_3NWlKlhmlRM0Lgb | 0.7 | 1.3 | -0.9 |
R_Wp7FZE1ziZ9czSN | 0.2 | -0.4 | -0.3 |
R_SJlfo8Lpb6XTHGh | -0.1 | 0.1 | 0.4 |
R_1Kegjs7Q3AL49wO | -0.1 | -0.3 | -0.2 |
R_1IY1urS9bmfIpbW | 1.6 | 0.3 | -0.3 |
Related resources
Analysis & Reporting
Margin of Error 11 min read
Text analysis 44 min read, sentiment analysis 21 min read, behavioural analytics 12 min read, descriptive statistics 15 min read, statistical significance calculator 18 min read, zero-party data 12 min read, request demo.
Ready to learn more about Qualtrics?

Contact Us (315) 303-2040
- Market Research Company Blog
Factor Analysis In Research: Types & Examples
by Tim Gell
Posted at: 7/1/2024 12:30 PM

Factor analysis in market research is a statistical method used to uncover underlying dimensions, or factors, in a dataset.
By examining patterns of correlation between variables, factor analysis helps to identify groups of variables that are highly interrelated and can be used to explain a common underlying theme.
Factor analysis can be best used for complex situations where there are many data sets and potentially many variables to summarize.
In this blog, we will explore the different types of factor analysis, their benefits, and examples of when to use them.
What is Factor Analysis?
Factor analysis is a commonly used data reduction statistical technique within the context of market research. The goal of factor analysis is to discover relationships between variables within a dataset by looking at correlations.
This advanced technique groups questions that are answered similarly among respondents in a survey.
The output will be a set of latent factors that represent questions that “move” together.
In other words, a resulting factor may consist of several survey questions whose data tend to increase or decrease in unison.
If you don’t need the underlying factors in your dataset and just want to understand the relationship between variables, regression analysis may be a better fit.
Things to Remember for Factor Analysis
There are a few concepts to keep in mind when doing factor analysis. These concepts guide the way that factor analysis is applied to certain projects and it’s interpretation.
- Variance: This measures how much values are off from the average. Since you want to understand the influence of the factors, variance will help understand how much variance there is in the results.
- Factor Score: This is a number representation of how strong each variable is from the original data. This is also related to a specific factor and can also be called the “component score”. It helps determine which variables are most changed by factors and which are most important.
- Factor Loading: this is typically a coefficient for correlation related to the variable/factor combination. Higher factor loadings means there is a stronger influence on the variables.
What are the Different Types of Factor Analysis?
When discussing this topic, it is always good to distinguish between the different types of factor analysis.
There are different approaches that achieve similar results in the end, but it's important to understand that there is different math going on behind the scenes for each method.
Types of factor analysis include:
- Principal component analysis
- Exploratory factor analysis
- Confirmatory factor analysis
1. Principal component analysis
Factor analysis assumes the existence of latent factors within the dataset, and then works backward from there to identify the factors.
In contrast, principal component analysis (also known as PCA) uses the variables within a dataset to create a composite of the other variables.
With PCA, you're starting with the variables and then creating a weighted average called a “component,” similar to a factor.
2. Exploratory factor analysis
In exploratory factor analysis, you're forming a hypothesis about potential relationships between your variables.
You might be using this approach if you're not sure what to expect in the way of factors.
You may need assistance with identifying the underlying themes among your survey questions and in this case, I recommend working with a market research company , like Drive Research.
Exploratory factor analysis ultimately helps understand how many factors are present in the data and what the skeleton of the factors might look like.
The process involves a manual review of factor loadings values for each data input, which are outputs to assess the suitability of the factors.
Do these factors make sense? If they don’t make adjustments to the inputs and try again.
If they do, you often move forward to the next step of confirmatory factor analysis.
3. Confirmatory factor analysis
Exploratory factor analysis and confirmatory factor analysis go hand in hand.
Now that you have a hypothesis from exploratory factor analysis, confirmatory factor analysis is going to test that hypothesis of potential relationships in your variables.
This process is essentially fine-tuning your factors so that you land at a spot where the factors make sense with respect to your objectives.
The sought outcome of confirmatory factor analysis is to achieve statistically sound and digestible factors for yourself or a client.
A best practice for confirmatory factor analysis is testing the model's goodness of fit.
This involves splitting your data into two equal segments: a test set and a training set.
The next step is to test the goodness of fit on that training data set, which includes applying the created factors from the training data set to the test dataset.
If you achieve similar factors in both sets, this then gives you thumbs up that the model is statistically valid.
How Factor Analysis Can Benefit You
1. Spot trends within your data
If you are part of a business and leveraging factor analysis with your data, some of the advantages include the ability to spot trends or themes within your data.
Certain attributes may be connected in a way you wouldn’t have known otherwise.
You may learn that different customer behaviors and attitudes are closely related. This knowledge can be used to inform marketing decisions when it comes to your product or service.
2. Pinpoint the number of factors in a data set
Factor analysis, or exploratory factor analysis more specifically, can also be used to pinpoint the right number of factors within a data set.
Knowing how many overarching factors you need to worry about allows you to spend your time focusing on the aspects of your data that have the greatest impact.
This will save you time, instill confidence in the results, and equip you with more actionable information.
3. Streamlines segmenting data
Lastly, factor analysis can be a great first step and lead-in for a cluster analysis if you are planning a customer segmentation study .
As a prerequisite, factor analysis streamlines the inputs for your segmentation. It helps to eliminate redundancies or irrelevant data, giving you a result that is clearer and easier to understand.
Here are 6 easy steps to conducting customer segmentation . Factor analysis could fit nicely between Step 3 and Step 4 if you are working with a high number of inputs.
When We Recommend Using Factor Analysis
Factor analysis is a great tool when working with large sets of interconnected data.
In our experience, it’s designed to help companies understand the hidden patterns or structures within data collected. It can simplify complex information, which is especially important when managing numerous variables.
For example, if your company gathered extensive customer feedback through surveys, factor analysis can transform those responses into more manageable, meaningful categories.
Factor analysis helps condense variables like customer satisfaction, product quality, and customer service into broader factors like 'product satisfaction' or 'customer experience'.
Examples of Performing a Factor Analysis
With so many types of market research , factor analysis has a wide range of applications.
Although, employee surveys and customer surveys are two of the best examples of when factor analysis is most helpful.
1. Employee surveys
For example, when using a third party for employee surveys, ask if the employee survey company can use factor analysis.
In these surveys, businesses aim to learn what matters most to their employees.
Because there is a myriad of variables that impact the employee experience, factor analysis has the potential to narrow down all these variables into a few manageable latent factors.
You might learn that flexibility, growth opportunities, and compensation are three key factors propelling your employees’ experiences.
Understanding these categories will make the management or hiring process that much easier.
2. Customer surveys
Factor analysis can also be a great application when conducting customer satisfaction surveys .
Let's say you have a lot of distinct variables going on in relation to customer preferences.
Customers are weighing these various product attributes each time before they make a purchase.
Factor analysis can group these attributes into useful factors, enabling you to see the forest through the trees.
You may have a hunch about what the categories would be, but factor analysis gives an approach backed by statistics to say this is how your product attributes should be grouped.
Factor Analysis Best Practices
1. Use the right inputs
For any market research study in which you plan to use factor analysis, you also need to make sure you have the proper inputs.
What it comes down to is asking survey questions that capture ordinal quantitative data.
Open-ended answers are not going to be useful for factor analysis.
Valid input data could involve rating scales, Likert scales, or even Yes/No questions that can be boiled down to binary ones and zeros.
Any combination of these questions could be effectively used for factor analysis.
2. Include enough data points
It is also imperative to include enough data inputs.
Running factor analysis on 50 attributes will tell you a whole lot more than an analysis on 5 attributes.
After all, the idea is to throw an unorganized mass of attributes into the analysis to see what latent factors really exist among them.
3. Large sample sizes are best
A large sample size will also convey more confidence when you share the results of the factor analysis.
At least 100 responses per audience segment in the analysis is a good starting point, if possible.
Contact Drive Research to Perform Factor Analysis
Drive Research is a national market research company that can help with lots of market research projects. Advanced methods like factor analysis are a part of our wheelhouse to get the most out of your data.
Interested in learning more about our market research services ? Reach out through any of the four ways below.
- Message us on our website
- Email us at [email protected]
- Call us at 888-725-DATA
- Text us at 315-303-2040
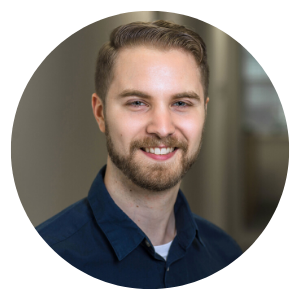
As a Senior Research Analyst, Tim is involved in every stage of a market research project for our clients. He first developed an interest in market research while studying at Binghamton University based on its marriage of business, statistics, and psychology
Learn more about Tim, here .
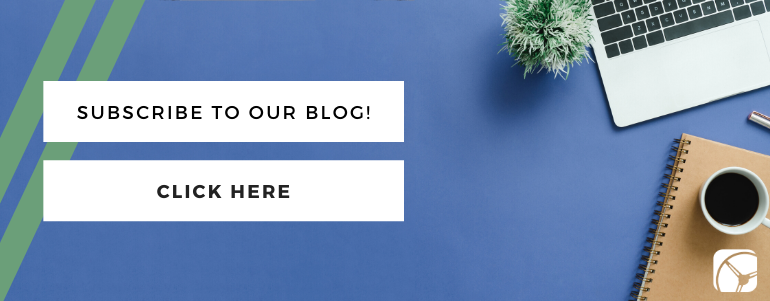
Categories: Market Research Analysis
Need help with your project? Get in touch with Drive Research.
View Our Blog
Factor Analysis in Marketing Research
- Home > What We Do > Research Methods > Statistical Techniques > Factor Analysis in Marketing Research
Factor analysis in marketing research aims to describe a large number of variables or questions by using a reduced set of underlying variables, called factors.
Contact Us >
To find out more about factor analysis and how it can help your business
What is factor analysis in marketing research?
Factor analysis explains a pattern of similarity between observed variables. Questions which belong to one factor are highly correlated with each other; unlike cluster analysis, which classifies respondents, factor analysis groups variables.
There are two types of factor analysis in marketing research: exploratory and confirmatory. Exploratory factor analysis is driven by the data, i.e. the data determines the factors. Confirmatory factor analysis, used in structural equation modelling, tests and confirms hypotheses.
Factor analysis in market research is often used in customer satisfaction studies to identify underlying service dimensions, and in profiling studies to determine core attitudes. For example, as part of a national survey on political opinions, respondents may answer three separate questions regarding environmental policy, reflecting issues at the local, regional and national level. Factor analysis can be used to establish whether the three measures do, in fact, measure the same thing.
It can also prove to be useful when a lengthy questionnaire needs to be shortened, but still retain key questions. Factor analysis indicates which questions can be omitted without losing too much information.
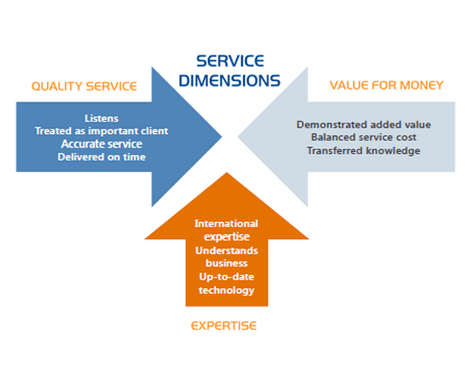
Contact Us >
Privacy Overview
Cookie | Duration | Description |
---|---|---|
__hssrc | session | This cookie is set by Hubspot whenever it changes the session cookie. The __hssrc cookie set to 1 indicates that the user has restarted the browser, and if the cookie does not exist, it is assumed to be a new session. |
cookielawinfo-checkbox-advertisement | 1 year | Set by the GDPR Cookie Consent plugin, this cookie is used to record the user consent for the cookies in the "Advertisement" category . |
cookielawinfo-checkbox-analytics | 11 months | This cookie is set by GDPR Cookie Consent plugin. The cookie is used to store the user consent for the cookies in the category "Analytics". |
cookielawinfo-checkbox-functional | 11 months | The cookie is set by GDPR cookie consent to record the user consent for the cookies in the category "Functional". |
cookielawinfo-checkbox-necessary | 11 months | This cookie is set by GDPR Cookie Consent plugin. The cookies is used to store the user consent for the cookies in the category "Necessary". |
cookielawinfo-checkbox-others | 11 months | This cookie is set by GDPR Cookie Consent plugin. The cookie is used to store the user consent for the cookies in the category "Other. |
cookielawinfo-checkbox-performance | 11 months | This cookie is set by GDPR Cookie Consent plugin. The cookie is used to store the user consent for the cookies in the category "Performance". |
CookieLawInfoConsent | 1 year | Records the default button state of the corresponding category & the status of CCPA. It works only in coordination with the primary cookie. |
viewed_cookie_policy | 11 months | The cookie is set by the GDPR Cookie Consent plugin and is used to store whether or not user has consented to the use of cookies. It does not store any personal data. |
Cookie | Duration | Description |
---|---|---|
__cf_bm | 30 minutes | This cookie, set by Cloudflare, is used to support Cloudflare Bot Management. |
__hssc | 30 minutes | HubSpot sets this cookie to keep track of sessions and to determine if HubSpot should increment the session number and timestamps in the __hstc cookie. |
bcookie | 2 years | LinkedIn sets this cookie from LinkedIn share buttons and ad tags to recognize browser ID. |
bscookie | 2 years | LinkedIn sets this cookie to store performed actions on the website. |
lang | session | LinkedIn sets this cookie to remember a user's language setting. |
lidc | 1 day | LinkedIn sets the lidc cookie to facilitate data center selection. |
UserMatchHistory | 1 month | LinkedIn sets this cookie for LinkedIn Ads ID syncing. |
Cookie | Duration | Description |
---|---|---|
__hstc | 5 months 27 days | This is the main cookie set by Hubspot, for tracking visitors. It contains the domain, initial timestamp (first visit), last timestamp (last visit), current timestamp (this visit), and session number (increments for each subsequent session). |
_ga | 2 years | The _ga cookie, installed by Google Analytics, calculates visitor, session and campaign data and also keeps track of site usage for the site's analytics report. The cookie stores information anonymously and assigns a randomly generated number to recognize unique visitors. |
_gat_gtag_UA_3031018_3 | 1 minute | Set by Google to distinguish users. |
_gid | 1 day | Installed by Google Analytics, _gid cookie stores information on how visitors use a website, while also creating an analytics report of the website's performance. Some of the data that are collected include the number of visitors, their source, and the pages they visit anonymously. |
CONSENT | 2 years | YouTube sets this cookie via embedded youtube-videos and registers anonymous statistical data. |
hubspotutk | 5 months 27 days | HubSpot sets this cookie to keep track of the visitors to the website. This cookie is passed to HubSpot on form submission and used when deduplicating contacts. |
undefined | never | Wistia sets this cookie to collect data on visitor interaction with the website's video-content, to make the website's video-content more relevant for the visitor. |
vuid | 2 years | Vimeo installs this cookie to collect tracking information by setting a unique ID to embed videos to the website. |
Cookie | Duration | Description |
---|---|---|
VISITOR_INFO1_LIVE | 5 months 27 days | A cookie set by YouTube to measure bandwidth that determines whether the user gets the new or old player interface. |
YSC | session | YSC cookie is set by Youtube and is used to track the views of embedded videos on Youtube pages. |
yt-remote-connected-devices | never | YouTube sets this cookie to store the video preferences of the user using embedded YouTube video. |
yt-remote-device-id | never | YouTube sets this cookie to store the video preferences of the user using embedded YouTube video. |
Cookie | Duration | Description |
---|---|---|
AnalyticsSyncHistory | 1 month | No description |
closest_office_location | 1 month | No description |
li_gc | 2 years | No description |
loglevel | never | No description available. |
ssi--lastInteraction | 10 minutes | This cookie is used for storing the date of last secure session the visitor had when visiting the site. |
ssi--sessionId | 1 year | This cookie is used for storing the session ID which helps in reusing the one the visitor had already used. |
user_country | 1 month | No description available. |
- Skip to secondary menu
- Skip to main content
- Skip to primary sidebar
Statistics By Jim
Making statistics intuitive
Factor Analysis Guide with an Example
By Jim Frost 19 Comments
What is Factor Analysis?
Factor analysis uses the correlation structure amongst observed variables to model a smaller number of unobserved, latent variables known as factors. Researchers use this statistical method when subject-area knowledge suggests that latent factors cause observable variables to covary. Use factor analysis to identify the hidden variables.
Analysts often refer to the observed variables as indicators because they literally indicate information about the factor. Factor analysis treats these indicators as linear combinations of the factors in the analysis plus an error. The procedure assesses how much of the variance each factor explains within the indicators. The idea is that the latent factors create commonalities in some of the observed variables.
For example, socioeconomic status (SES) is a factor you can’t measure directly. However, you can assess occupation, income, and education levels. These variables all relate to socioeconomic status. People with a particular socioeconomic status tend to have similar values for the observable variables. If the factor (SES) has a strong relationship with these indicators, then it accounts for a large portion of the variance in the indicators.
The illustration below illustrates how the four hidden factors in blue drive the measurable values in the yellow indicator tags.

Researchers frequently use factor analysis in psychology, sociology, marketing, and machine learning.
Let’s dig deeper into the goals of factor analysis, critical methodology choices, and an example. This guide provides practical advice for performing factor analysis.
Analysis Goals
Factor analysis simplifies a complex dataset by taking a larger number of observed variables and reducing them to a smaller set of unobserved factors. Anytime you simplify something, you’re trading off exactness with ease of understanding. Ideally, you obtain a result where the simplification helps you better understand the underlying reality of the subject area. However, this process involves several methodological and interpretative judgment calls. Indeed, while the analysis identifies factors, it’s up to the researchers to name them! Consequently, analysts debate factor analysis results more often than other statistical analyses.
While all factor analysis aims to find latent factors, researchers use it for two primary goals. They either want to explore and discover the structure within a dataset or confirm the validity of existing hypotheses and measurement instruments.
Exploratory Factor Analysis (EFA)
Researchers use exploratory factor analysis (EFA) when they do not already have a good understanding of the factors present in a dataset. In this scenario, they use factor analysis to find the factors within a dataset containing many variables. Use this approach before forming hypotheses about the patterns in your dataset. In exploratory factor analysis, researchers are likely to use statistical output and graphs to help determine the number of factors to extract.
Exploratory factor analysis is most effective when multiple variables are related to each factor. During EFA, the researchers must decide how to conduct the analysis (e.g., number of factors, extraction method, and rotation) because there are no hypotheses or assessment instruments to guide them. Use the methodology that makes sense for your research.
For example, researchers can use EFA to create a scale, a set of questions measuring one factor. Exploratory factor analysis can find the survey items that load on certain constructs.
Confirmatory Factor Analysis (CFA)
Confirmatory factor analysis (CFA) is a more rigid process than EFA. Using this method, the researchers seek to confirm existing hypotheses developed by themselves or others. This process aims to confirm previous ideas, research, and measurement and assessment instruments. Consequently, the nature of what they want to verify will impose constraints on the analysis.
Before the factor analysis, the researchers must state their methodology including extraction method, number of factors, and type of rotation. They base these decisions on the nature of what they’re confirming. Afterwards, the researchers will determine whether the model’s goodness-of-fit and pattern of factor loadings match those predicted by the theory or assessment instruments.
In this vein, confirmatory factor analysis can help assess construct validity. The underlying constructs are the latent factors, while the items in the assessment instrument are the indicators. Similarly, it can also evaluate the validity of measurement systems. Does the tool measure the construct it claims to measure?
For example, researchers might want to confirm factors underlying the items in a personality inventory. Matching the inventory and its theories will impose methodological choices on the researchers, such as the number of factors.
We’ll get to an example factor analysis in short order, but first, let’s cover some key concepts and methodology choices you’ll need to know for the example.
Learn more about Validity and Construct Validity .
In this context, factors are broader concepts or constructs that researchers can’t measure directly. These deeper factors drive other observable variables. Consequently, researchers infer the properties of unobserved factors by measuring variables that correlate with the factor. In this manner, factor analysis lets researchers identify factors they can’t evaluate directly.
Psychologists frequently use factor analysis because many of their factors are inherently unobservable because they exist inside the human brain.
For example, depression is a condition inside the mind that researchers can’t directly observe. However, they can ask questions and make observations about different behaviors and attitudes. Depression is an invisible driver that affects many outcomes we can measure. Consequently, people with depression will tend to have more similar responses to those outcomes than those who are not depressed.
For similar reasons, factor analysis in psychology often identifies and evaluates other mental characteristics, such as intelligence, perseverance, and self-esteem. The researchers can see how a set of measurements load on these factors and others.
Method of Factor Extraction
The first methodology choice for factor analysis is the mathematical approach for extracting the factors from your dataset. The most common choices are maximum likelihood (ML), principal axis factoring (PAF), and principal components analysis (PCA).
You should use either ML or PAF most of the time.
Use ML when your data follow a normal distribution. In addition to extracting factor loadings, it also can perform hypothesis tests, construct confidence intervals, and calculate goodness-of-fit statistics .
Use PAF when your data violates multivariate normality. PAF doesn’t assume that your data follow any distribution, so you could use it when they are normally distributed. However, this method can’t provide all the statistical measures as ML.
PCA is the default method for factor analysis in some statistical software packages, but it isn’t a factor extraction method. It is a data reduction technique to find components. There are technical differences, but in a nutshell, factor analysis aims to reveal latent factors while PCA is only for data reduction. While calculating the components, PCA doesn’t assess the underlying commonalities that unobserved factors cause.
PCA gained popularity because it was a faster algorithm during a time of slower, more expensive computers. If you’re using PCA for factor analysis, do some research to be sure it’s the correct method for your study. Learn more about PCA in, Principal Component Analysis Guide and Example .
There are other methods of factor extraction, but the factor analysis literature has not strongly shown that any of them are better than maximum likelihood or principal axis factoring.
Number of Factors to Extract
You need to specify the number of factors to extract from your data except when using principal component components. The method for determining that number depends on whether you’re performing exploratory or confirmatory factor analysis.
Exploratory Factor Analysis
In EFA, researchers must specify the number of factors to retain. The maximum number of factors you can extract equals the number of variables in your dataset. However, you typically want to reduce the number of factors as much as possible while maximizing the total amount of variance the factors explain.
That’s the notion of a parsimonious model in statistics. When adding factors, there are diminishing returns. At some point, you’ll find that an additional factor doesn’t substantially increase the explained variance. That’s when adding factors needlessly complicates the model. Go with the simplest model that explains most of the variance.
Fortunately, a simple statistical tool known as a scree plot helps you manage this tradeoff.
Use your statistical software to produce a scree plot. Then look for the bend in the data where the curve flattens. The number of points before the bend is often the correct number of factors to extract.
The scree plot below relates to the factor analysis example later in this post. The graph displays the Eigenvalues by the number of factors. Eigenvalues relate to the amount of explained variance.

The scree plot shows the bend in the curve occurring at factor 6. Consequently, we need to extract five factors. Those five explain most of the variance. Additional factors do not explain much more.
Some analysts and software use Eigenvalues > 1 to retain a factor. However, simulation studies have found that this tends to extract too many factors and that the scree plot method is better. (Costello & Osborne, 2005).
Of course, as you explore your data and evaluate the results, you can use theory and subject-area knowledge to adjust the number of factors. The factors and their interpretations must fit the context of your study.
Confirmatory Factor Analysis
In CFA, researchers specify the number of factors to retain using existing theory or measurement instruments before performing the analysis. For example, if a measurement instrument purports to assess three constructs, then the factor analysis should extract three factors and see if the results match theory.
Factor Loadings
In factor analysis, the loadings describe the relationships between the factors and the observed variables. By evaluating the factor loadings, you can understand the strength of the relationship between each variable and the factor. Additionally, you can identify the observed variables corresponding to a specific factor.
Interpret loadings like correlation coefficients . Values range from -1 to +1. The sign indicates the direction of the relations (positive or negative), while the absolute value indicates the strength. Stronger relationships have factor loadings closer to -1 and +1. Weaker relationships are close to zero.
Stronger relationships in the factor analysis context indicate that the factors explain much of the variance in the observed variables.
Related post : Correlation Coefficients
Factor Rotations
In factor analysis, the initial set of loadings is only one of an infinite number of possible solutions that describe the data equally. Unfortunately, the initial answer is frequently difficult to interpret because each factor can contain middling loadings for many indicators. That makes it hard to label them. You want to say that particular variables correlate strongly with a factor while most others do not correlate at all. A sharp contrast between high and low loadings makes that easier.
Rotating the factors addresses this problem by maximizing and minimizing the entire set of factor loadings. The goal is to produce a limited number of high loadings and many low loadings for each factor.
This combination lets you identify the relatively few indicators that strongly correlate with a factor and the larger number of variables that do not correlate with it. You can more easily determine what relates to a factor and what does not. This condition is what statisticians mean by simplifying factor analysis results and making them easier to interpret.
Graphical illustration
Let me show you how factor rotations work graphically using scatterplots .
Factor analysis starts by calculating the pattern of factor loadings. However, it picks an arbitrary set of axes by which to report them. Rotating the axes while leaving the data points unaltered keeps the original model and data pattern in place while producing more interpretable results.
To make this graphable in two dimensions, we’ll use two factors represented by the X and Y axes. On the scatterplot below, the six data points represent the observed variables, and the X and Y coordinates indicate their loadings for the two factors. Ideally, the dots fall right on an axis because that shows a high loading for that factor and a zero loading for the other.

For the initial factor analysis solution on the scatterplot, the points contain a mixture of both X and Y coordinates and aren’t close to a factor’s axis. That makes the results difficult to interpret because the variables have middling loads on all the factors. Visually, they’re not clumped near axes, making it difficult to assign the variables to one.
Rotating the axes around the scatterplot increases or decreases the X and Y values while retaining the original pattern of data points. At the blue rotation on the graph below, you maximize one factor loading while minimizing the other for all data points. The result is that the loads are high on one indicator but low on the other.

On the graph, all data points cluster close to one of the two factors on the blue rotated axes, making it easy to associate the observed variables with one factor.
Types of Rotations
Throughout these rotations, you work with the same data points and factor analysis model. The model fits the data for the rotated loadings equally as well as the initial loadings, but they’re easier to interpret. You’re using a different coordinate system to gain a different perspective of the same pattern of points.
There are two fundamental types of rotation in factor analysis, oblique and orthogonal.
Oblique rotations allow correlation amongst the factors, while orthogonal rotations assume they are entirely uncorrelated.
Graphically, orthogonal rotations enforce a 90° separation between axes, as shown in the example above, where the rotated axes form right angles.
Oblique rotations are not required to have axes forming right angles, as shown below for a different dataset.

Notice how the freedom for each axis to take any orientation allows them to fit the data more closely than when enforcing the 90° constraint. Consequently, oblique rotations can produce simpler structures than orthogonal rotations in some cases. However, these results can contain correlated factors.
Promax | Varimax |
Oblimin | Equimax |
Direct Quartimin | Quartimax |
In practice, oblique rotations produce similar results as orthogonal rotations when the factors are uncorrelated in the real world. However, if you impose an orthogonal rotation on genuinely correlated factors, it can adversely affect the results. Despite the benefits of oblique rotations, analysts tend to use orthogonal rotations more frequently, which might be a mistake in some cases.
When choosing a rotation method in factor analysis, be sure it matches your underlying assumptions and subject-area knowledge about whether the factors are correlated.
Factor Analysis Example
Imagine that we are human resources researchers who want to understand the underlying factors for job candidates. We measured 12 variables and perform factor analysis to identify the latent factors. Download the CSV dataset: FactorAnalysis
The first step is to determine the number of factors to extract. Earlier in this post, I displayed the scree plot, which indicated we should extract five factors. If necessary, we can perform the analysis with a different number of factors later.
For the factor analysis, we’ll assume normality and use Maximum Likelihood to extract the factors. I’d prefer to use an oblique rotation, but my software only has orthogonal rotations. So, we’ll use Varimax. Let’s perform the analysis!
Interpreting the Results

In the bottom right of the output, we see that the five factors account for 81.8% of the variance. The %Var row along the bottom shows how much of the variance each explains. The five factors are roughly equal, explaining between 13.5% to 19% of the variance. Learn about Variance .
The Communality column displays the proportion of the variance the five factors explain for each variable. Values closer to 1 are better. The five factors explain the most variance for Resume (0.989) and the least for Appearance (0.643).
In the factor analysis output, the circled loadings show which variables have high loadings for each factor. As shown in the table below, we can assign labels encompassing the properties of the highly loading variables for each factor.
1 | Relevant Background | Academic record, Potential, Experience |
2 | Personal Characteristics | Confidence, Likeability, Appearance |
3 | General Work Skills | Organization, Communication |
4 | Writing Skills | Letter, Resume |
5 | Overall Fit | Company Fit, Job Fit |
In summary, these five factors explain a large proportion of the variance, and we can devise reasonable labels for each. These five latent factors drive the values of the 12 variables we measured.
Hervé Abdi (2003), “Factor Rotations in Factor Analyses,” In: Lewis-Beck M., Bryman, A., Futing T. (Eds.) (2003). Encyclopedia of Social Sciences Research Methods . Thousand Oaks (CA): Sage.
Brown, Michael W., (2001) “ An Overview of Analytic Rotation in Exploratory Factor Analysis ,” Multivariate Behavioral Research , 36 (1), 111-150.
Costello, Anna B. and Osborne, Jason (2005) “ Best practices in exploratory factor analysis: four recommendations for getting the most from your analysis ,” Practical Assessment, Research, and Evaluation : Vol. 10 , Article 7.
Share this:

Reader Interactions
May 26, 2024 at 8:51 am
Good day Jim, I am running in troubles in terms of the item analysis on the 5 point Likert scale that I am trying to create. The thing is, is that my CFI is around 0.9 and TLI is around 0.8 which is good but my RMSEA and SRMR has a awful result as the RMSEA is around 0.1 and SRMR is 0.2. And it is a roadblock for me, I want to ask on how I can improve my RMSEA and SRMR? so that it would reach the cut off.
I hope that his message would reach you and thank you for taking the time and reading and responding to my troubled question.
May 15, 2024 at 11:27 am
Good day, Sir Jim. I am currently trying to create a 5-Likert scale that tries to measure National Identity Conformity in three ways: (1) Origin – (e.g., Americans are born in/from America), (2) Culture (e.g., Americans are patriotic) and (3) Belief (e.g., Americans embrace being Americans).
In the process of establishing the scale’s validity, I was told to use Exploratory Factor Analysis, and I would like to ask what methods of extraction and rotation can be best used to ensure that the inter-item validity of my scale is good. I would also like to understand how I can avoid crossloading or limit crossloading factors.
May 15, 2024 at 3:13 pm
I discuss those issues in this post. I’d recommend PAF as the method of extraction because your data being Likert scale won’t be normally distribution. Read the Method of Factor Extraction section for more information.
As for cross-loading, the method of rotation can help with that. The choice depends largely on subject-area knowledge and what works best for your data, so I can’t provide a suggested method. Read the Factor Rotations section for more information about that. For instance, if you get cross-loadings with orthogonal rotations, using an oblique rotation might help.
If factor rotation doesn’t sufficiently reduce cross-loading, you might need to rework your questions so they’re more distinct, remove problematic items, or increase your sample size (can provide more stable factor solutions and clearer patterns of loadings). In this scenario where changing rotations doesn’t help, you’ll need to determine whether the underlying issue is with your questions or having to small of a sample size.
I hope that helps!
March 6, 2024 at 10:20 pm
What does negative loadings mean? How to proceed further with these loadings?
March 6, 2024 at 10:44 pm
Loadings are like correlation coefficients and range from -1 to +1. More extreme positive and negative values indicate stronger relationships. Negative loadings indicate a negative relationship between the latent factors and observed variables. Highly negative values are as good as highly positive values. I discuss this in detail in the the Factor Loadings section of this post.
March 6, 2024 at 10:10 am
Good day Jim,
The methodology seems loaded with opportunities for errors. So often we are being asked to translate a nebulous English word into some sort of mathematical descriptor. As an example, in the section labelled ‘Interpreting the Results’, what are we to make of the words ‘likeability’ or ‘self-confidence’ ? How can we possibly evaluate those things…and to three significant decimal places ?
You Jim, understand and use statistical methods correctly. Yet, too often people who apply statistics fail to examine the language of their initial questions and end up doing poor analysis. Worse, many don’t understand the software they use.
On a more cheery note, keep up the great work. The world needs a thousand more of you.
March 6, 2024 at 5:08 pm
Thanks for the thoughtful comment. I agree with your concerns.
Ideally, all of those attributes are measured using validated measurement scales. The field of psychology is pretty good about that for terms that seem kind of squishy. For instance, they usually have thorough validation processes for personality traits, etc. However, your point is well taken, you need to be able to trust your data.
All statistical analyses depend on thorough subject-area knowledge, and that’s very true for factor analysis. You must have a solid theoretical understanding of these latent factors from extensive research before considering FA. Then FA can see if there’s evidence that they actually exist. But, I do agree with you that between the rotations and having to derive names to associate with the loadings, it can be a fairly subjective process.
Thanks so much for your kind words! I appreciate them because I do strive for accuracy.
March 2, 2024 at 8:44 pm
sir, i want to know that after successfully identifying my 3 factors with above give method now i want to regress on the data how to get single value for each factor rather than these number of values
February 28, 2024 at 7:48 am
Hello, Thanks for your effort on this post, it really helped me a lot. I want your recommendation for my case if you don’t mind.
I’m working on my research and I’ve 5 independent variables and 1 dependent variable, I want to use a factor analysis method in order to know which variable contributes the most in the dependent variable.
Also, what kind of data checks and preparations shall I make before starting the analysis.
Thanks in advance for your consideration.
February 28, 2024 at 1:46 pm
Based on the information you provided, I don’t believe factor analysis is the correct analysis for you.
Factor analysis is primarily used for understanding the structure of a set of variables and for reducing data dimensions by identifying underlying latent factors. It’s particularly useful when you have a large number of observed variables and believe that they are influenced by a smaller number of unobserved factors.
Instead, it sounds like you have the IVs and DV and want to understand the relationships between them. For that, I recommend multiple regression. Learn more in my post about When to Use Regression . After you settle on a model, there are several ways to Identify the Most Important Variables in the Model .
In terms of checking assumptions, familiarize yourself with the Ordinary Least Squares Regression Assumptions . Least squares regression is the most common and is a good place to start.
Best of luck with your analysis!
December 1, 2023 at 1:01 pm
What would be the eign value in efa
November 1, 2023 at 4:42 am
Hi Jim, this is an excellent yet succinct article on the topic. A very basic question, though: the dataset contains ordinal data. Is this ok? I’m a student in a Multivariate Statistics course, and as far as I’m aware, both PCA and common factor analysis dictate metric data. Or is it assumed that since the ordinal data has been coded into a range of 0-10, then the data is considered numeric and can be applied with PCA or CFA?
Sorry for the dumb question, and thank you.
November 1, 2023 at 8:00 pm
That’s a great question.
For the example in this post, we’re dealing with data on a 10 point scale where the differences between all points are equal. Consequently, we can treat discrete data as continuous data.
Now, to your question about ordinal data. You can use ordinal data with factor analysis however you might need to use specific methods.
For ordinal data, it’s often recommended to use polychoric correlations instead of Pearson correlations. Polychoric correlations estimate the correlation between two latent continuous variables that underlie the observed ordinal variables. This provides a more accurate correlation matrix for factor analysis of ordinal data.
I’ve also heard about categorical PCA and nonlinear Factor Analysis that use a monotonical transformation of ordinal data.
I hope that helps clarify it for you!
September 2, 2023 at 4:14 pm
Once identifying how much each variability the factors contribute, what steps could we take from here to make predictions about variables ?
September 2, 2023 at 6:53 pm
Hi Brittany,
Thanks for the great question! And thanks for you kind words in your other comment! 🙂
What you can do is calculate all the factor scores for each observation. Some software will do this for you as an option. Or, you can input values into the regression equations for the factor scores that are included in the output.
Then use these scores as the independent variables in regression analysis. From there, you can use the regression model to make predictions .
Ideally, you’d evaluate the regression model before making predictions and use cross validation to be sure that the model works for observations outside the dataset you used to fit the model.
September 2, 2023 at 4:13 pm
Wow! This was really helpful and structured very well for interpretation. Thank you!
October 6, 2022 at 10:55 am
I can imagine that Prof will have further explanations on this down the line at some point in future. I’m waiting… Thanks Prof Jim for your usual intuitive manner of explaining concepts. Funsho
September 26, 2022 at 8:08 am
Thanks for a very comprehensive guide. I learnt a lot. In PCA, we usually extract the components and use it for predictive modeling. Is this the case with Factor Analysis as well? Can we use factors as predictors?
September 26, 2022 at 8:27 pm
I have not used factors as predictors, but I think it would be possible. However, PCA’s goal is to maximize data reduction. This process is particularly valuable when you have many variables, low sample size and/or collinearity between the predictors. Factor Analysis also reduces the data but that’s not its primary goal. Consequently, my sense is that PCA is better for that predictive modeling while Factor Analysis is better for when you’re trying to understand the underlying factors (which you aren’t with PCA). But, again, I haven’t tried using factors in that way nor I have compared the results to PCA. So, take that with a grain of salt!
Comments and Questions Cancel reply

- > Machine Learning
Factor Analysis: Types & Applications
- Soumyaa Rawat
- Sep 14, 2021
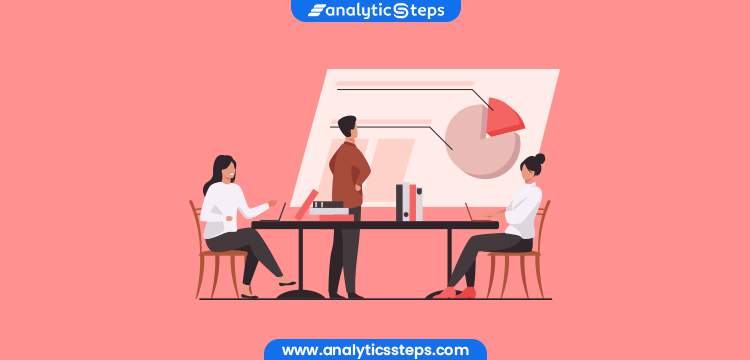
What is Factor Analysis ?
Data is everywhere. From data research to artificial intelligence technology, data has become an essential commodity that is being perceived as a link between our past and future. Is an organization willing to collect its past records?
Data is the key solution to this problem. Is any programmer willing to formulate a Machine Learning algorithm ? Data is what s/he needs to begin with.
While the world has moved on to technology, it still is unaware of the fact that data is the building block of all these technological advancements that have together made the world so advanced.
When it comes to data, a number of tools and techniques are put to work to arrange, organize, and accumulate data the way one wants to. Factor Analysis is one of them. A data reduction technique, Factor Analysis is a statistical method used to reduce the number of observed factors for a much better insight into a given dataset.
But first, we shall understand what is a factor. A factor is a set of observed variables that have similar responses to an action. Since variables in a given dataset can be too much to deal with, Factor Analysis condenses these factors or variables into fewer variables that are actionable and substantial to work upon.
A technique of dimensionality reduction in data mining, Factor Analysis works on narrowing the availability of variables in a given data set, allowing deeper insights and better visibility of patterns for data research.
Most commonly used to identify the relationship between various variables in statistics , Factor Analysis can be thought of as a compressor that compresses the size of variables and produces a much enhanced, insightful, and accurate variable set.
“FA is considered an extension of principal component analysis since the ultimate objective for both techniques is a data reduction.” Factor Analysis in Data Reduction
Types of Factor Analysis
Developed in 1904 by Spearman, Factor Analysis is broadly divided into various types based upon the approach to detect underlying variables and establish a relationship between them.
While there are a variety of techniques to conduct factor analysis like Principal Component Analysis or Independent Component Analysis , Factor Analysis can be divided into 2 types which we will discuss below. Let us get started.
Confirmatory Factor Analysis
As the name of this concept suggests, Confirmatory Factor Analysis (CFA) lets one determine whether a relationship between factors or a set of overserved variables and their underlying components exists.
It helps one confirm whether there is a connection between two components of variables in a given dataset. Usually, the purpose of CFA is to test whether certain data fit the requirements of a particular hypothesis.
The process begins with a researcher formulating a hypothesis that is made to fit along the lines of a certain theory. If the constraints imposed on a model do not fit well with the data, then the model is rejected, and it is confirmed that no relationship exists between a factor and its underlying construct. Perhaps hypothetical testing also finds a space in the world of Factor Analysis.
Exploratory Factor Analysis
In the case of Exploratory Factor Statistical Analysis , the purpose is to determine/explore the underlying latent structure of a large set of variables. EFA, unlike CFA, tends to uncover the relationship, if any, between measured variables of an entity (for example - height, weight, etc. in a human figure).
While CFA works on finding a relationship between a set of observed variables and their underlying structure, this works to uncover a relationship between various variables within a given dataset.
Conducting Exploratory Factor Analysis involves figuring the total number of factors involved in a dataset.
“EFA is generally considered to be more of a theory-generating procedure than a theory-testing procedure. In contrast, confirmatory factor analysis (CFA) is generally based on a strong theoretical and/or empirical foundation that allows the researcher to specify an exact factor model in advance.” EFA in Hypothesis Testing
Applications of Factor Analysis
With immense use in various fields in real life, this segment presents a list of applications of Factor Analysis and the way FA is used in day-to-day operations.
Applications of factor analysis
Marketing is defined as the act of promoting a good or a service or even a brand. When it comes to Factor Analysis in marketing, one can benefit immensely from this statistical method.
In order to boost marketing campaigns and accelerate success, in the long run, companies employ Factor Analysis techniques that help to find a correlation between various variables or factors of a marketing campaign.
Moreover, FA also helps to establish connections with customer satisfaction and consequent feedback after a marketing campaign in order to check its efficacy and impact on the audiences.
That said, the realm of marketing can largely benefit from Factor Analysis and trigger sales with respect to much-enhanced feedback and customer satisfaction reports.
(Must read: Marketing management guide )
Data Mining
In data mining, Factor Analysis can play a role as important as that of artificial intelligence. Owing to its ability to transform a complex and vast dataset into a group of filtered out variables that are related to each other in some way or the other, FA eases out the process of data mining.
For data scientists, the tedious task of finding relationships and establishing correlation among various variables has always been full of obstacles and errors.
However, with the help of this statistical method, data mining has become much more advanced.
(Also read: Data mining tools )
Machine Learning
Machine Learning and data mining tools go hand in hand. Perhaps this is the reason why Factor Analysis finds a place among Machine Learning tools and techniques.
As Factor Analysis in machine learning helps in reducing the number of variables in a given dataset to procure a more accurate and enhanced set of observed factors, various machine learning algorithms are put to use to work accordingly.
They are trained well with humongous data to rightly work in order to give way to other applications. An unsupervised machine learning algorithm, FA is largely used for dimensionality reduction in machine learning.
Thereby, machine learning can very well collaborate with Factor Analysis to give rise to data mining techniques and make the task of data research massively efficient.
(Recommended blog: Data mining software )
Nutritional Science
Nutritional Science is a prominent field of work in the contemporary scenario. By focusing on the dietary practices of a given population, Factor Analysis helps to establish a relationship between the consumption of nutrients in an adult’s diet and the nutritional health of that person.
Furthermore, an individual’s nutrient intake and consequent health status have helped nutritionists to compute the appropriate quantity of nutrients one should intake in a given period of time.
The application of Factor Analysis in business is rather surprising and satisfactory.
Remember the times when business firms had to employ professionals to dig out patterns from past records in order to lay a road ahead for strategic business plans?
Well, gone are the days when so much work had to be done. Thanks to Factor Analysis, the world of business can use it for eliminating the guesswork and formulating more accurate and straightforward decisions in various aspects like budgeting, marketing, production, and transport.
Pros and Cons of Factor Analysis
Having learned about Factor Analysis in detail, let us now move on to looking closely into the pros and cons of this statistical method.
Pros of Factor Analysis
Measurable attributes.
The first and foremost pro of FA is that it is open to all measurable attributes. Be it subjective or objective, any kind of attribute can be worked upon when it comes to this statistical technique.
Unlike some statistical models that only work on objective attributes, Factor Analysis goes well with both subjective and objective attributes.
Cost-Effective
While data research and data mining algorithms can cost a lot due to the extraordinary charges, this statistical model is surprisingly cost-effective and does not take many resources to work with.
That said, it can be incorporated by any beginner or an experienced professional in light of its cost-effective and easy approach towards data mining and data reduction.
Flexible Approach
While many machine learning algorithms are rigid and constricted to a single approach, Factor Analysis does not work that way.
Rather, this statistical model has a flexible approach towards multivariate datasets that let one obtain relationships or correlations between various variables and their underlying components.
(Must read: AI algorithms )

Cons of Factor Analysis
Incomprehensive results.
While there are many pros of Factor Analysis, there are various cons of this method as well. Primarily, Factor Analysis can procure incompetent results due to incomprehensive datasets.
While various data points can have similar traits, some other variables or factors can go unnoticed due to being isolated in a vast dataset. That said, the results of this method could be incomprehensive.
Non-Identification of Complicated Factors
Another drawback of Factor Analysis is that it does not identify complicated factors that underlie a dataset.
While some results could clearly indicate a correlation between two variables, some complicated correlations can go unnoticed in such a method.
Perhaps the non-identification of complicated factors and their relationships could be an issue for data research.
Reliant on Theory
Even though Factor Analysis skills can be imitated by machine learning algorithms, this method is still reliant on theory and thereby data researchers.
While many components of a dataset can be handled by a computer, some other details are required to be looked into by humans.
Thus, one of the major drawbacks of Factor Analysis is that it is somehow reliant on theory and cannot fully function without manual assistance.
(Suggested reading: Deep learning algorithms )
Summing Up
To sum up, Factor Analysis is an extensive statistical model that is used to reduce dimensions of a given dataset with the help of condensing observed variables in a smaller size.
(Top reading: Statistical data distribution models )
By arranging observed variables in groups of super-variables, Factor Analysis has immensely impacted the way data mining is done. With numerous fields relying on this technique for better performance, FA is the need of the hour.
Share Blog :

Be a part of our Instagram community
Trending blogs
5 Factors Influencing Consumer Behavior
Elasticity of Demand and its Types
An Overview of Descriptive Analysis
What is PESTLE Analysis? Everything you need to know about it
What is Managerial Economics? Definition, Types, Nature, Principles, and Scope
5 Factors Affecting the Price Elasticity of Demand (PED)
6 Major Branches of Artificial Intelligence (AI)
Scope of Managerial Economics
Dijkstra’s Algorithm: The Shortest Path Algorithm
Different Types of Research Methods
Latest Comments
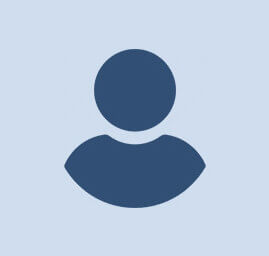
All Subjects
study guides for every class
That actually explain what's on your next test, factor analysis, from class:, principles of marketing.
Factor analysis is a statistical technique used to identify underlying factors or latent variables that explain the relationships among a set of observed variables. It is a data reduction method that aims to find the minimum number of factors that can account for the observed variance in a dataset.
congrats on reading the definition of Factor Analysis . now let's actually learn it.
5 Must Know Facts For Your Next Test
- Factor analysis is commonly used in marketing research to identify the underlying dimensions or factors that drive consumer behavior, attitudes, and perceptions.
- The technique can help researchers uncover the latent variables that influence customer satisfaction, brand loyalty, or purchase intentions.
- Factor analysis can also be used to reduce a large number of variables into a smaller set of factors, making it easier to interpret and model complex marketing phenomena.
- The results of factor analysis can inform the development of marketing strategies, segmentation approaches, and the design of marketing research instruments.
- Proper implementation of factor analysis requires careful consideration of sample size, variable selection, and the interpretation of factor loadings and communalities.
Review Questions
- Factor analysis can play a crucial role in a marketing research plan by helping researchers uncover the underlying dimensions or factors that drive consumer behavior, attitudes, and perceptions. By identifying the latent variables that influence key marketing outcomes, such as customer satisfaction, brand loyalty, or purchase intentions, factor analysis can inform the development of effective marketing strategies, segmentation approaches, and the design of marketing research instruments. The technique can also be used to reduce a large number of variables into a smaller set of factors, making it easier to interpret and model complex marketing phenomena.
- The results of factor analysis can provide valuable insights that enhance the interpretation and analysis of marketing research data. By identifying the underlying factors that explain the relationships among observed variables, factor analysis can help researchers better understand the key drivers of consumer behavior, attitudes, and perceptions. The factor loadings and communalities generated by the analysis can reveal the relative importance and interrelationships of different variables, enabling researchers to develop more accurate and meaningful models of marketing phenomena. Additionally, the reduced set of factors can be used as input variables in subsequent analyses, such as regression or structural equation modeling, to explore the relationships between these latent constructs and other marketing outcomes of interest.
- Factor analysis is a powerful statistical technique that can significantly contribute to the success of a comprehensive marketing research plan. By identifying the underlying factors that drive consumer behavior, attitudes, and perceptions, factor analysis can provide researchers with a deeper understanding of the complex phenomena they are studying. This knowledge can inform the development of more effective marketing strategies, segmentation approaches, and the design of marketing research instruments. Moreover, the results of factor analysis can enhance the interpretation and analysis of marketing research data, enabling researchers to develop more accurate and meaningful models of marketing phenomena. When properly implemented, factor analysis can be a crucial component of a successful marketing research plan, helping researchers uncover valuable insights and make more informed decisions that ultimately drive business success.
Related terms
Principal Component Analysis (PCA) : A related technique that reduces the dimensionality of a dataset by transforming the original variables into a smaller set of uncorrelated principal components that explain the maximum amount of variance.
Exploratory Factor Analysis (EFA) : A form of factor analysis that is used to uncover the underlying structure of a relatively large set of variables without imposing a preconceived structure on the outcome.
Confirmatory Factor Analysis (CFA) : A more theory-driven approach to factor analysis that tests whether the data fits a hypothesized measurement model based on prior theory or research.
" Factor Analysis " also found in:
Subjects ( 37 ).
- AP Psychology
- Abnormal Psychology
- Advanced Communication Research Methods
- Advanced Design Strategy and Software
- Advanced quantitative methods
- Advertising Management
- Advertising Strategy
- Advertising and Society
- Art Market Economics
- Biostatistics
- Business Anthropology
- Communication Research Methods
- Data Visualization
- Data, Inference, and Decisions
- Engineering Applications of Statistics
- Experimental Design
- Financial Technology
- Gamification in Business
- International Public Relations
- Intro to Psychology
- Introduction to Political Research
- Introduction to Public Health
- Market Research: Tools and Techniques for Data Collection and Analysis
- Marketing Research
- Marketing Strategy
- Media Expression and Communication
- Media Strategies and Management
- Media Strategy
- Music Psychology
- Predictive Analytics in Business
- Professionalism and Research in Nursing
- Public Policy Analysis
- Reproducible and Collaborative Statistical Data Science
- Sampling Surveys
- Social Psychology
- Statistical Inference
- Strategic Improvisation in Business
© 2024 Fiveable Inc. All rights reserved.
Ap® and sat® are trademarks registered by the college board, which is not affiliated with, and does not endorse this website..
Factor analysis: decipher complex data and make better decisions
Appinio Research · 05.12.2023 · 16min read
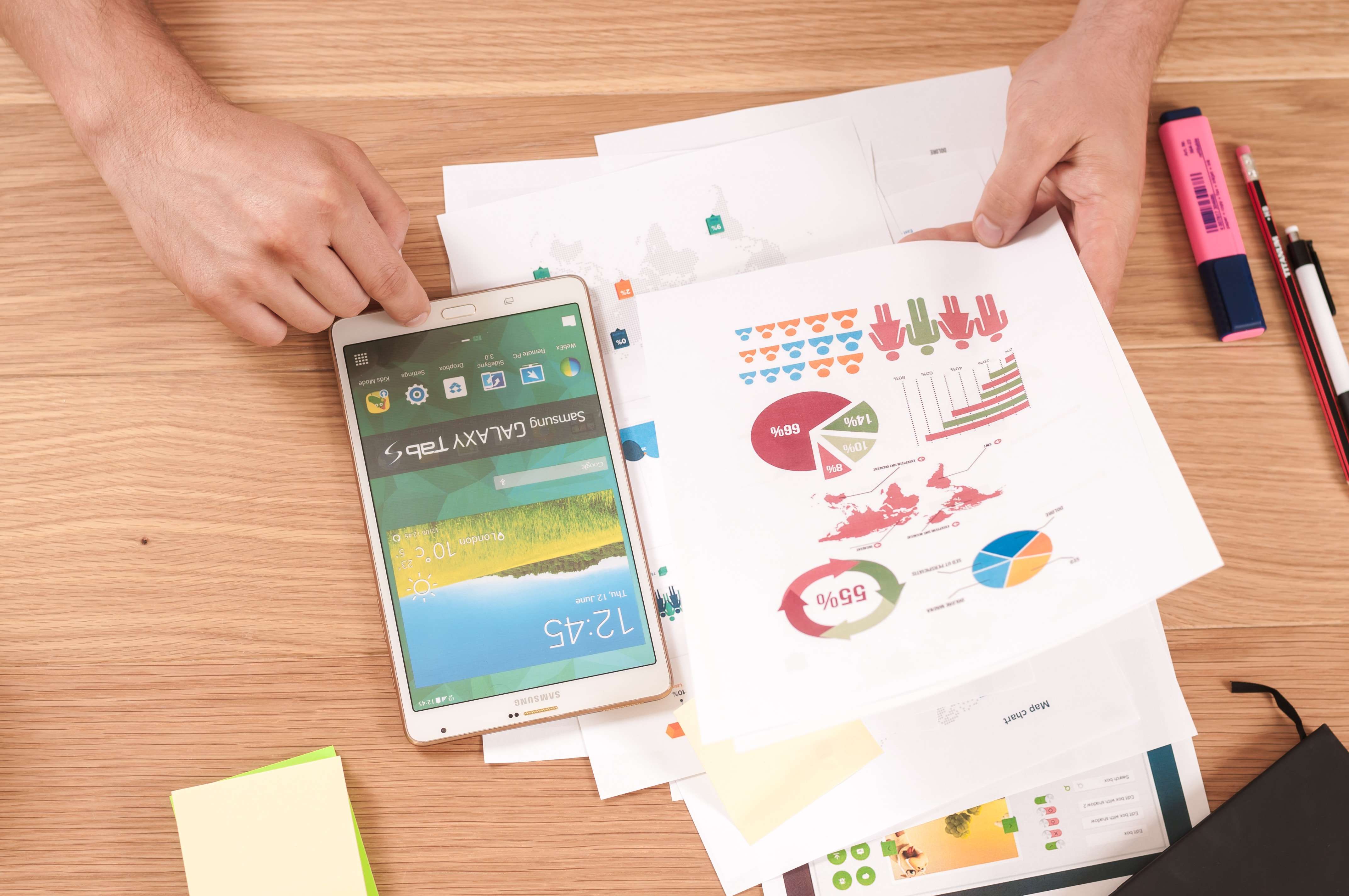
In today's fast-paced marketing world, making decisions based on data gives companies a real edge. But let's face it, collecting data is one thing, figuring out what it all means is another ball game. That's where factor analysis swoops in like a superhero for your complex data sets.
Imagine it like this: it's like a magic trick to make big, messy data sets simpler and spot the cool patterns. In this guide, we're going to chat about where factor analysis comes from, how it does its thing, the good stuff it brings to the table, and even its not-so-great sides. Plus, we'll spill the beans on the difference between exploratory and confirmatory factor analysis and a bunch more exciting tips.
What is factor analysis?
Factor analysis, a statistical method tailored for unraveling complex data sets, serves as a crucial analytical tool in modern decision-making processes.
At its core, factor analysis strives to streamline extensive datasets by distilling them into a few essential factors. The ultimate goal is to enhance clarity and manageability in handling substantial amounts of data.
Additionally, factor analysis plays a pivotal role in unearthing concealed patterns and structures within datasets, shedding light on intricate phenomena and relationships.
To appreciate the significance of this methodology, let's delve into a concise historical overview
A Historical Perspective
Originating in the late 19th century within the realm of psychology, factor analysis found its early roots during the endeavors of Francis Galton and Charles Spearman. Their pursuit revolved around developing tests to gauge intelligence.
Spearman, in 1904, introduced the first mathematical approach to factor analysis, laying the groundwork for its subsequent applications across diverse disciplines such as statistics, social sciences, economics, and market research.
Advancements in technology, coupled with the advent of sophisticated data analysis software, have propelled factor analysis into a more accessible and precise realm.
Far from a relic of the past, this methodology stands as a contemporary powerhouse for pattern recognition and information extraction from intricate data sets.
What is factor analysis used for?
Whether deployed in a confirmatory or exploratory manner, factor analysis proves instrumental in simplifying market segmentation, devising targeted marketing strategies, and optimizing products and services.
Its ability to facilitate data-driven decision-making processes positions factor analysis as a catalyst for organizations seeking a competitive advantage.
By distilling complex data sets, factor analysis provides valuable insights into consumer behavior and preferences.
Market researchers leverage this methodology to identify patterns and structures in surveys and customer evaluations, offering a deeper understanding of purchasing behavior.
Ultimately, the reduction of factors and variables to their core elements enhances comprehension of the needs and preferences of target groups .
When is a factor analysis useful?
Factor analysis proves its worth in various scenarios, offering a multifaceted approach to data exploration. Let's break down its utility:
- Streamlining variables In instances where datasets boast an extensive array of variables, factor analysis steps in to categorize and simplify the data. By doing so, it transforms a potentially overwhelming set into a clearer and more interpretable format.
- Unveiling patterns Factor analysis, particularly in its explorative form, plays the role of a detective, unveiling concealed patterns and structures within the data. This process brings hidden connections to light, providing a richer understanding of the underlying dynamics.
Variable classification Ever wondered which factors carry the most weight in a dataset? Factor analysis shines a light on the variance within datasets, elucidating the influence each factor wields. This classification proves invaluable in discerning the pivotal elements within complex data structures.
- Testing theories and hypotheses For situations where concrete assumptions or hypotheses already exist regarding data content and structure, confirmatory factor analysis steps in. It systematically checks these assumptions, serving as a validation tool that either affirms or challenges existing theories.
- Segmentation mastery Factor analysis serves as a keen observer in the realm of customer profiles. By scrutinizing similarities and differences among these profiles, it becomes a linchpin in the definition of distinct customer segments. This segmentation approach provides nuanced insights into diverse consumer behaviors.
In essence, factor analysis transcends the mere simplification of data; it acts as a versatile analytical ally, unveiling the intricacies of datasets and offering a structured lens through which to understand, validate, and strategically utilize information.
Where does factor analysis reach its limits?
While factor analysis stands as a formidable tool, it's imperative to acknowledge its limits and exercise discretion based on specific objectives and data quality. This method, powerful as it may be, isn't a one-size-fits-all solution. Let's explore the situations where factor analysis might take a backseat:
- Not a panacea Factor analysis excels at reducing variables and exploring relationships within a dataset. However, when the goal is to pinpoint and form groups of similar data for a more specific segmentation, cluster analysis takes the lead. It's tailored for the task of identifying distinct groups and segments within a dataset, surpassing factor analysis in this particular arena.
- The power of regression When the emphasis shifts to understanding the relationship between variables, regression analysis often emerges as the more fitting choice. Unlike factor analysis, which hones in on complex patterns and hidden structures, regression analysis specifically delves into the influence of one or more independent variables on a dependent variable.
- Navigating customer preferences Factor analysis encounters its limitations when the focus zeroes in on discerning customer preferences or specific values tied to various product characteristics and features. In such cases, where a detailed understanding of customer preferences is key, conjoint analysis is a more tailored alternative.
In essence, while factor analysis stands as a robust methodology, its effectiveness is context-dependent.
Recognizing the unique strengths of other analytical tools, such as cluster analysis and regression analysis, ensures a comprehensive approach to data exploration and interpretation. The selection of the most suitable method hinges on a nuanced understanding of the specific objectives and the intricacies of the dataset at hand.
Where is factor analysis used?
Despite its roots in psychology, factor analysis has evolved into a versatile tool, finding applications across a spectrum of disciplines.
- Market research insights In the realm of market research , factor analysis takes center stage. Its primary role involves segmenting target and buyer groups, offering a nuanced understanding of product preferences. By deciphering these preferences, businesses can craft targeted marketing strategies that resonate with specific consumer segments.
- Economic and financial analysis Factor analysis extends its reach into the economic and financial sectors. Here, it delves into variables such as interest rates, inflation, and share prices. The objective is clear: to analyze and determine the risk-return profiles of investment portfolios. By identifying and understanding these key factors, financial analysts can make informed decisions, optimizing investment strategies.
- Healthcare insights The medical and healthcare domains leverage factor analysis for a different purpose. Here, it plays a crucial role in identifying associated symptoms or risk factors for diseases, particularly in fields like cardiovascular health. By discerning these factors, medical professionals can enhance diagnostic accuracy and refine treatment approaches.
- Tech-driven advancements Beyond traditional sectors, factor analysis steps into the realms of image and speech processing. In image processing, it excels at extracting features from large datasets, contributing to advancements in facial recognition technology. Similarly, in speech processing, factor analysis aids in enhancing the extraction of key features, refining the capabilities of speech recognition systems.
Exploratory vs. confirmatory factor analysis
Factor analysis adapts to the specific needs of research objectives and data scenarios, presenting two distinct methodologies: exploratory and confirmatory factor analysis.
- Exploratory Factor Analysis (EFA) Positioned at the inception of research endeavors, exploratory factor analysis shines when the terrain is uncharted or information is incomplete. It becomes the investigator's tool, delving into large datasets to unearth latent patterns that were previously unknown. In this phase, exploratory factor analysis not only simplifies data but also acts as a catalyst for generating new hypotheses, providing a solid foundation for subsequent stages of research.
- Confirmatory Factor Analysis (CFA) As research progresses into more advanced stages, confirmatory factor analysis takes the spotlight. This method is tailor-made for situations where existing hypotheses need validation. To employ confirmatory factor analysis effectively, a well-defined model is crucial, built upon established theoretical frameworks or previous studies. It operates as a precision tool, rigorously testing specific relationships between variables, aligning research outcomes with pre-existing theories.
In essence, the choice between exploratory and confirmatory factor analysis hinges on the stage of research and the clarity of existing hypotheses .
Exploratory factor analysis pioneers the exploration of unknown territories, unraveling patterns and generating new insights.
On the flip side, confirmatory factor analysis stands as the sentinel of validation, ensuring that research findings align with established theoretical constructs.
Together, these dual approaches empower researchers to navigate the intricate landscape of data analysis with precision and purpose.
What are the advantages and disadvantages of factor analysis?
Factor analysis, like any analytical tool, comes with a set of advantages and drawbacks, shaping its utility in various contexts.
- Data simplification and focus Factor analysis excels in simplifying intricate datasets, distilling them to their essential components. By highlighting the most crucial points, it transforms data complexity into a more manageable and insightful form.
- Unearthing hidden patterns The method serves as a detective, uncovering latent patterns that might otherwise slip through the cracks. This capability brings valuable insights, contributing to a more comprehensive understanding of the data.
- Clarity amidst complexity In the midst of the data jungle, factor analysis brings clarity. It acts as a guide, simplifying interpretation and making the data more accessible, aiding in effective decision-making.
- Testing ideas and theories Factor analysis provides a robust platform for hypothesis testing. By comparing data and checking for plausibility, it becomes a reliable tool for scrutinizing ideas and theories against empirical evidence.
Disadvantages
- Dependency on data quality The reliability of factor analysis is contingent upon the quality and comprehensiveness of the input data. In cases where data is lacking in these aspects, the results may be compromised.
- Assumption sensitivity The outcomes of factor analysis are sensitive to the clarity of data summaries and the assumptions underlying them. Ambiguities in these aspects can introduce uncertainties into the results.
- Incompatibility with poor data Factor analysis is not the go-to approach when dealing with incomplete or poor-quality data. Its effectiveness is contingent upon a robust dataset, and deviations from this standard may undermine its validity.
- Human judgment in factor selection The method relies on human judgment for factor selection, introducing an element of subjectivity. This reliance on interpretation can lead to errors, impacting the accuracy of the analysis.
- Risk of over-extraction Factor analysis, in its quest for patterns, runs the risk of extracting excessive detail from the data. This unintended consequence may increase complexity, potentially hindering rather than aiding understanding.
7 steps to optimal factor analysis
Wie funktioniert eine Faktorenanalyse in der Marktforschung? Dazu braucht es lediglich sieben Schritte – ohne dabei zu sehr ins Detail zu gehen:
The integration of factor analysis into market research involves a systematic process, encompassing seven key steps that pave the way for valuable insights:
Data preparation Begin by collecting comprehensive datasets, ensuring that the data is normalized for effective comparison. The choice between exploratory and confirmatory factor analysis is a critical decision at this stage and sets the tone for the subsequent analytical journey.
Determining factors The next step involves determining the number of factors to be extracted. This decision is driven by research objectives or can be guided by statistical methods, aligning the analysis with the specific goals of the study.
Implementation Employing statistical software, such as SPSS, becomes essential in this phase. These tools perform intricate mathematical calculations to extract factors, unveiling the underlying patterns within the datasets.
Factor rotation Factor rotation, while not altering the data's content, enhances interpretability. This step simplifies the factors' interpretation, making the results more accessible without modifying the intrinsic meaning of the data.
Interpretation of factors and factor loadings With factors in hand, attention turns to interpreting factor loadings. This analysis unveils the relationships between factors and original variables, paving the way to name and interpret these factors. It's the bridge to understanding the intricate interplay within the data.
Interpretation of results Delving into the interpreted factors provides insights into the underlying structures and correlations of data and variables. This critical phase aids in forming a cohesive narrative around the relationships discovered during factor analysis.
Use of results The derived factors, beyond merely simplifying datasets, become powerful tools. They serve as a foundation for developing hypotheses or, in the context of market research, segmenting target groups. The insights gained from factor analysis become integral to shaping strategic decisions and refining marketing approaches.
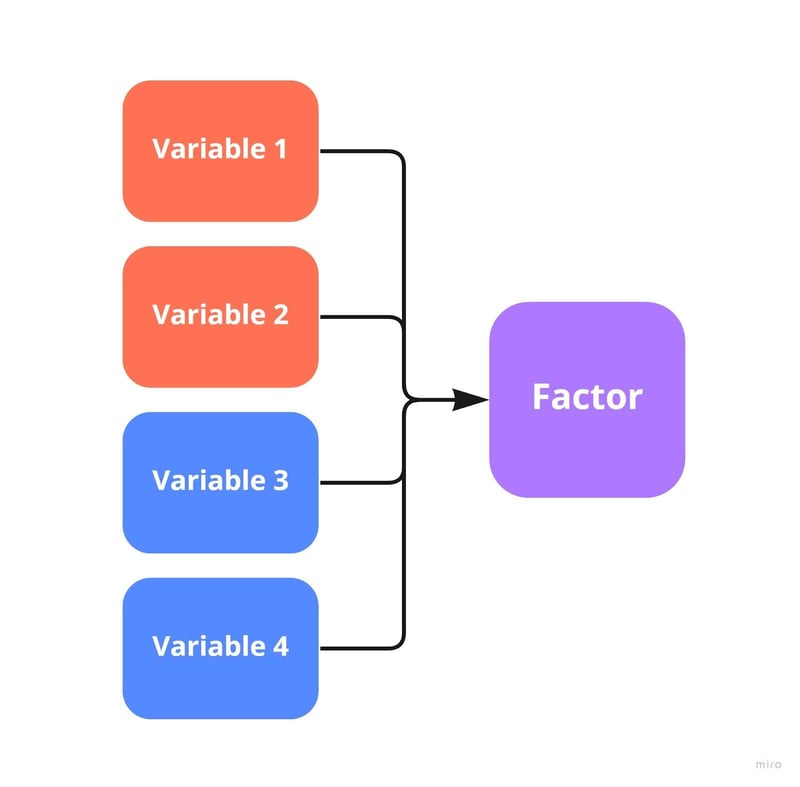
Example of a factor analysis: Reasons for buying a car
Let's shift from theory to the real-world application of factor analysis, exemplified in the automotive industry where understanding the factors influencing car buyers becomes paramount.
In this scenario, a company embarks on a data collection journey, gathering insights from hundreds of customers regarding various vehicle features. These features encompass a spectrum, ranging from engine performance and fuel efficiency to price, design, and safety features.
- Data collection The first step involves collecting a diverse dataset that encapsulates the multifaceted aspects of a car. Engine performance, fuel efficiency, price, design, and safety features are meticulously cataloged, forming the foundation for factor analysis.
- Factor identification Employing factor analysis, the company identifies the underlying factors exerting the most significant influence on the purchase decision. Through this analytical lens, factors like "environment" and "safety" emerge as the driving forces shaping customers' choices.
- Strategic decision-making Armed with these insights, the company gains a nuanced understanding of customer preferences. This clarity enables the development of targeted marketing strategies, tailoring messages to highlight the environmentally conscious and safety-oriented aspects of their vehicles.
- Product development Beyond marketing, the findings guide decisions in product development. Understanding that environmental concerns and safety features top the priority list, the company can focus on integrating and enhancing these elements in their vehicles, aligning their offerings with the genuine needs and desires of their customer base.
Conclusion: Understanding customers and target groups better with factor analysis
In the contemporary landscape of data-driven decision-making, factor analysis stands out as an invaluable asset for companies and market researchers alike.
Its ability in distilling clarity from complex data sets, unearthing concealed patterns, and facilitating informed decision-making positions it as an indispensable tool. By simplifying data and enhancing its structural organization, factor analysis empowers companies to decipher customer preferences, fine-tune marketing strategies, and align products with market needs.
The insights garnered extend beyond mere statistical analysis , delving into the intricate realm of consumer behaviors and preferences.
Factor analysis emerges not just as a methodology but as a powerful ally in navigating the competitive terrain, enabling businesses to not only survive but thrive by better serving the evolving needs of their customers.
Interested in running your own study?
In our dashboard, you will find questionnaire templates that you can customize and get the insights you need to bring your brand to the next level.
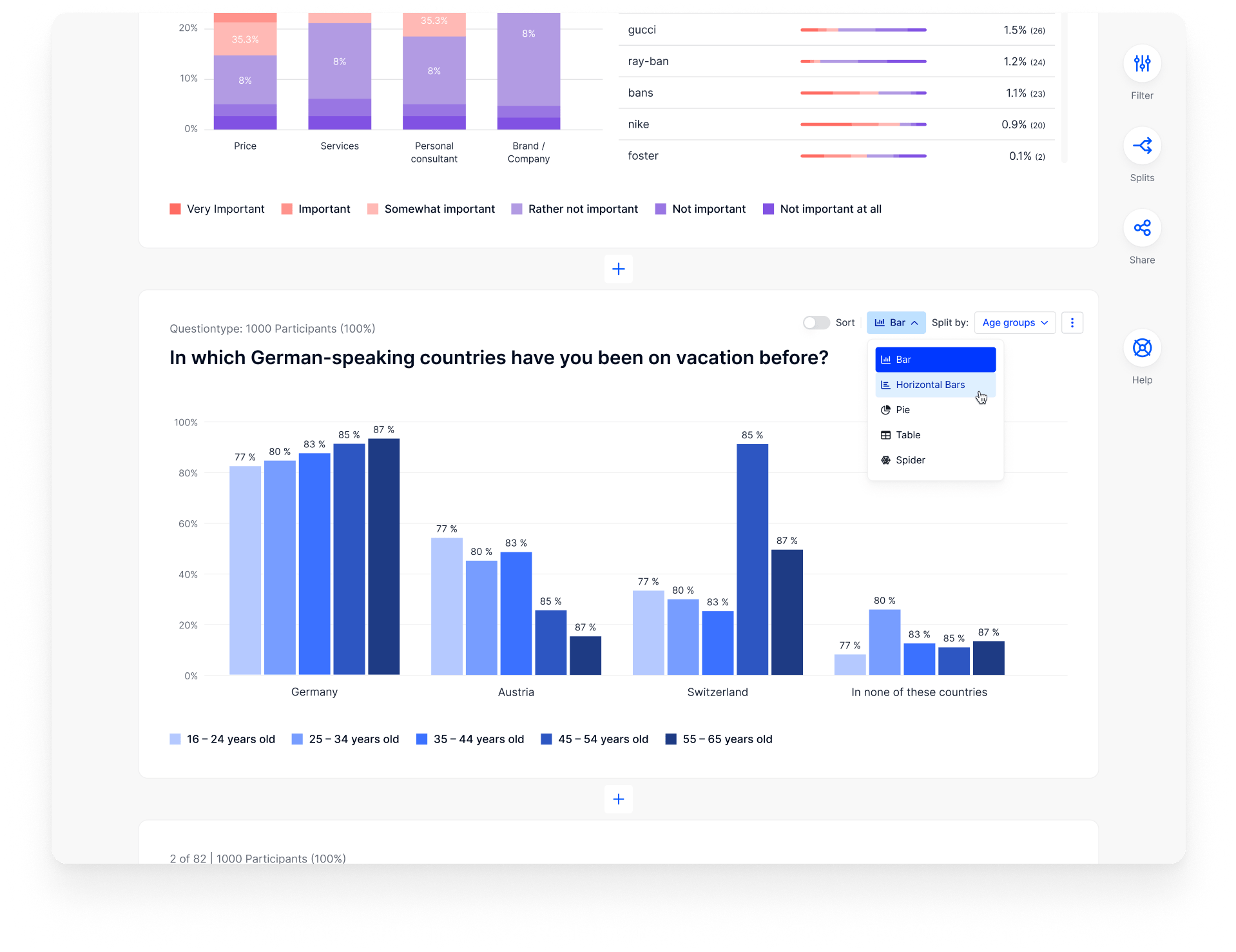
Get free access to the platform!
Join the loop 💌
Be the first to hear about new updates, product news, and data insights. We'll send it all straight to your inbox.
Get the latest market research news straight to your inbox! 💌
Wait, there's more
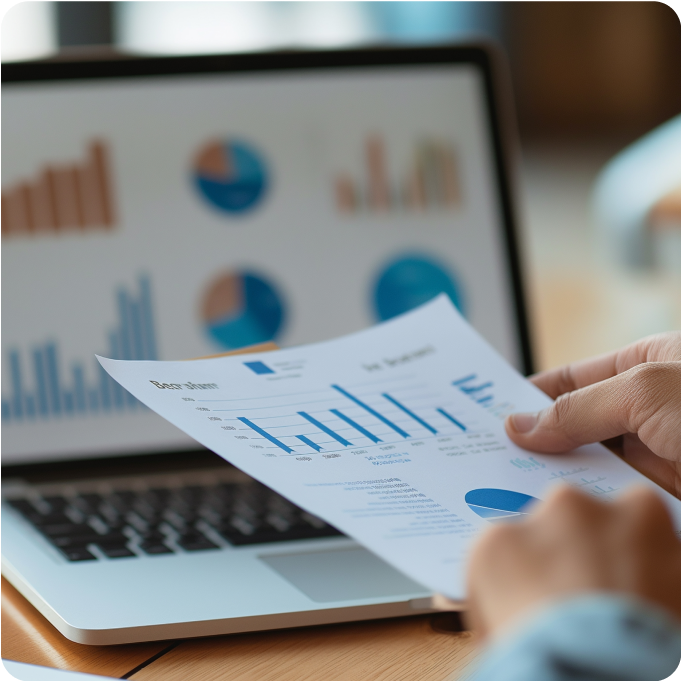
19.09.2024 | 8min read
Track Your Customer Retention & Brand Metrics for Post-Holiday Success
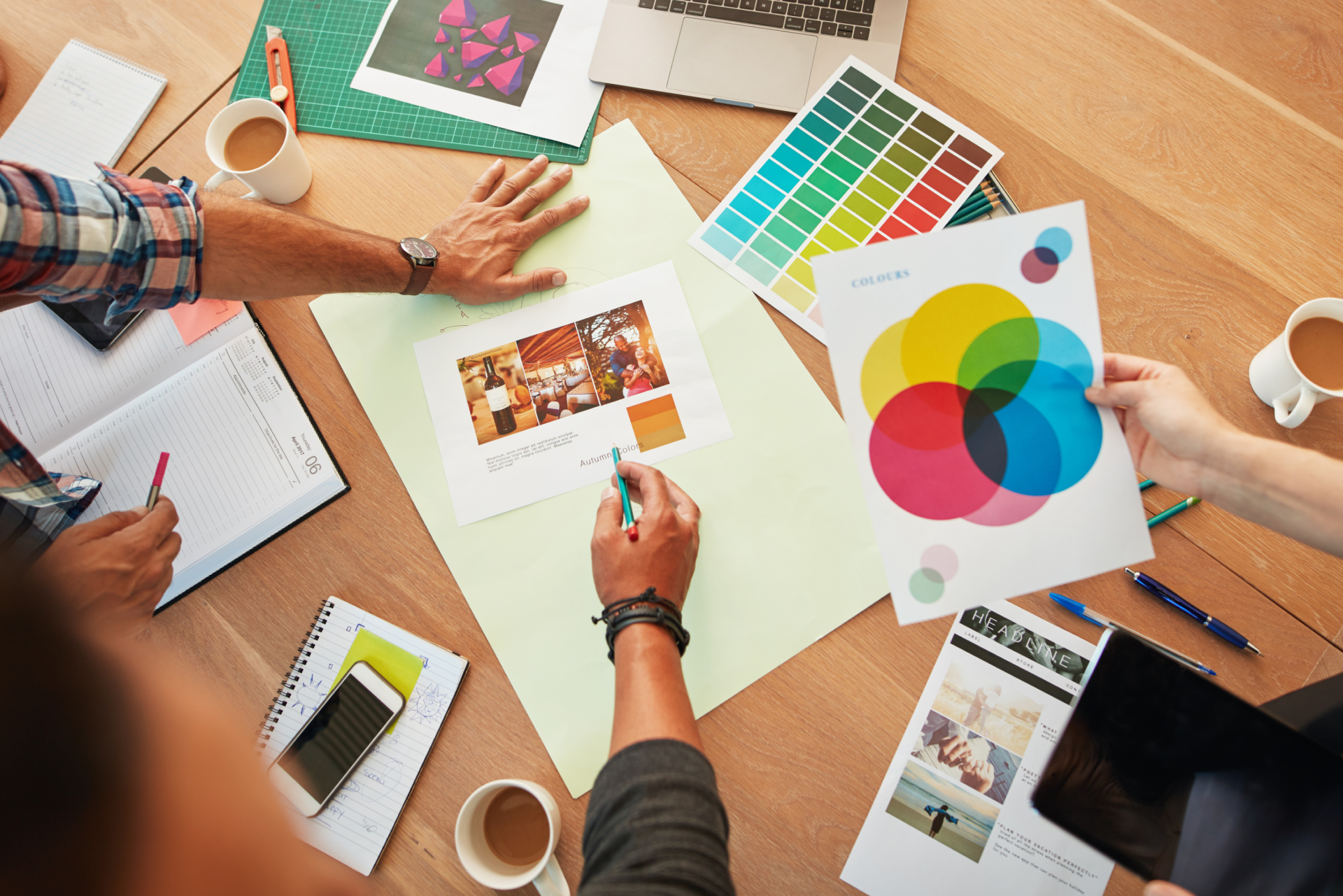
16.09.2024 | 10min read
Creative Checkup – Optimize Advertising Slogans & Creatives for ROI
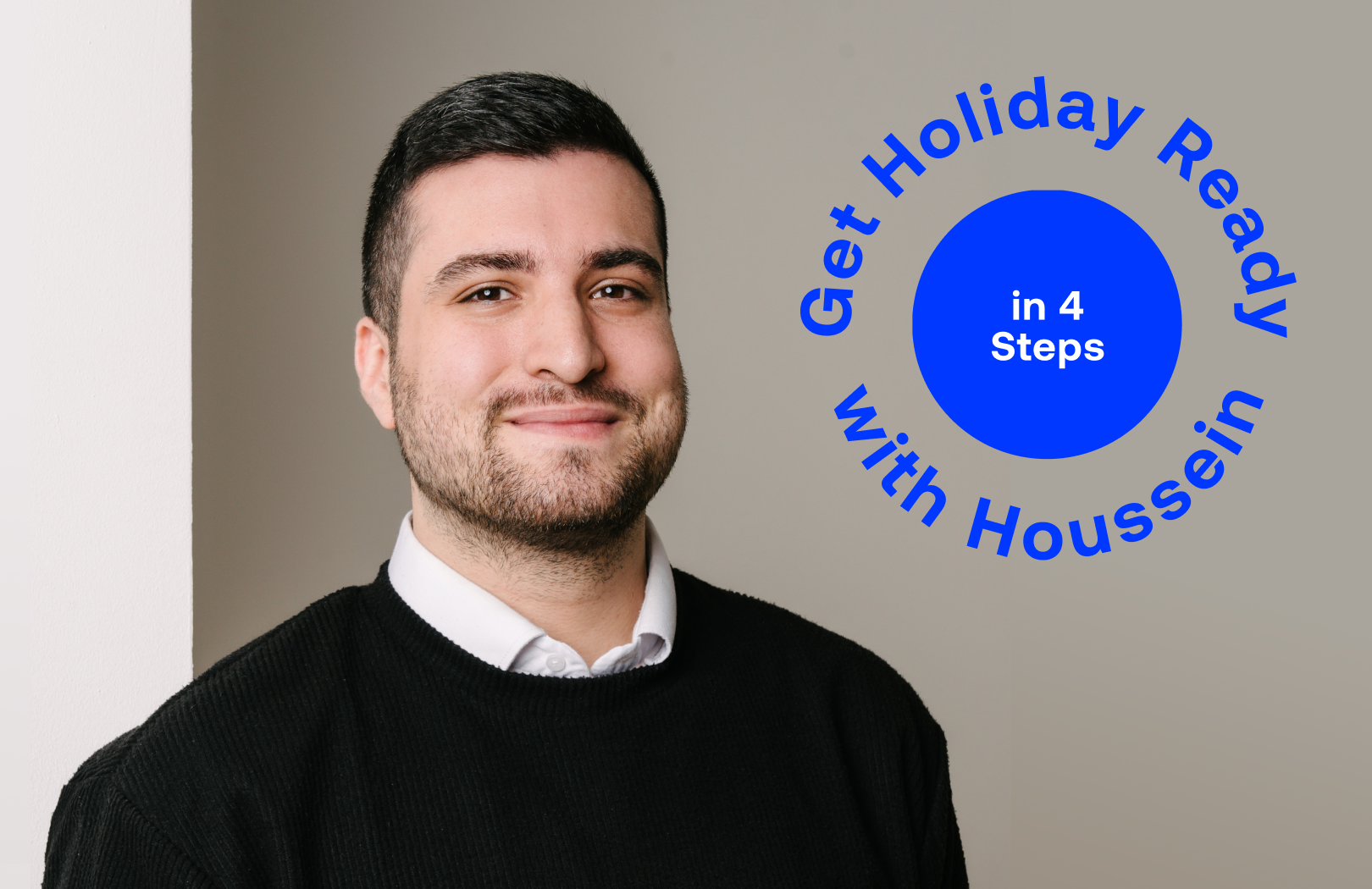
03.09.2024 | 8min read
Get your brand Holiday Ready: 4 Essential Steps to Smash your Q4

Factor Analysis 101: The Basics
- Market Research , Survey Tips
What is Factor Analysis?
Factor analysis is a powerful data reduction technique that enables researchers to investigate concepts that cannot easily be measured directly. By boiling down a large number of variables into a handful of comprehensible underlying factors, factor analysis results in easy-to-understand, actionable data.
By applying this method to your research, you can spot trends faster and see themes throughout your datasets, enabling you to learn what the data points have in common.
Unlike statistical methods such as regression analysis , factor analysis does not require defined variables.
Factor analysis is most commonly used to identify the relationship between all of the variables included in a given dataset.
The Objectives of Factor Analysis
Think of factor analysis as shrink wrap. When applied to a large amount of data, it compresses the set into a smaller set that is far more manageable, and easier to understand.
The overall objective of factor analysis can be broken down into four smaller objectives:
- To definitively understand how many factors are needed to explain common themes amongst a given set of variables.
- To determine the extent to which each variable in the dataset is associated with a common theme or factor.
- To provide an interpretation of the common factors in the dataset.
- To determine the degree to which each observed data point represents each theme or factor.
When to Use Factor Analysis
Determining when to use particular statistical methods to get the most insight out of your data can be tricky.
When considering factor analysis, have your goal top-of-mind.
There are three main forms of factor analysis. If your goal aligns to any of these forms, then you should choose factor analysis as your statistical method of choice:
Exploratory Factor Analysi s should be used when you need to develop a hypothesis about a relationship between variables.
Confirmatory Factor Analysis should be used to test a hypothesis about the relationship between variables.
Construct Validity should be used to test the degree to which your survey actually measures what it is intended to measure.
How To Ensure Your Survey is Optimized for Factor Analysis
If you know that you’ll want to perform a factor analysis on response data from a survey, there are a few things you can do ahead of time to ensure that your analysis will be straightforward, informative, and actionable.
Identify and Target Enough Respondents
Large datasets are the lifeblood of factor analysis. You’ll need large groups of survey respondents, often found through panel services , for factor analysis to yield significant results.
While variables such as population size and your topic of interest will influence how many respondents you need, it’s best to maintain a “more respondents the better” mindset.
The More Questions, The Better
While designing your survey , load in as many specific questions as possible. Factor analysis will fall flat if your survey only has a few broad questions.
The ultimate goal of factor analysis is to take a broad concept and simplify it by considering more granular, contextual information, so this approach will provide you the results you’re looking for.
Aim for Quantitative Data
If you’re looking to perform a factor analysis, you’ll want to avoid having open-ended survey questions .
By providing answer options in the form of scales (whether they be Likert Scales , numerical scales, or even ‘yes/no’ scales) you’ll save yourself a world of trouble when you begin conducting your factor analysis. Just make sure that you’re using the same scaled answer options as often as possible.
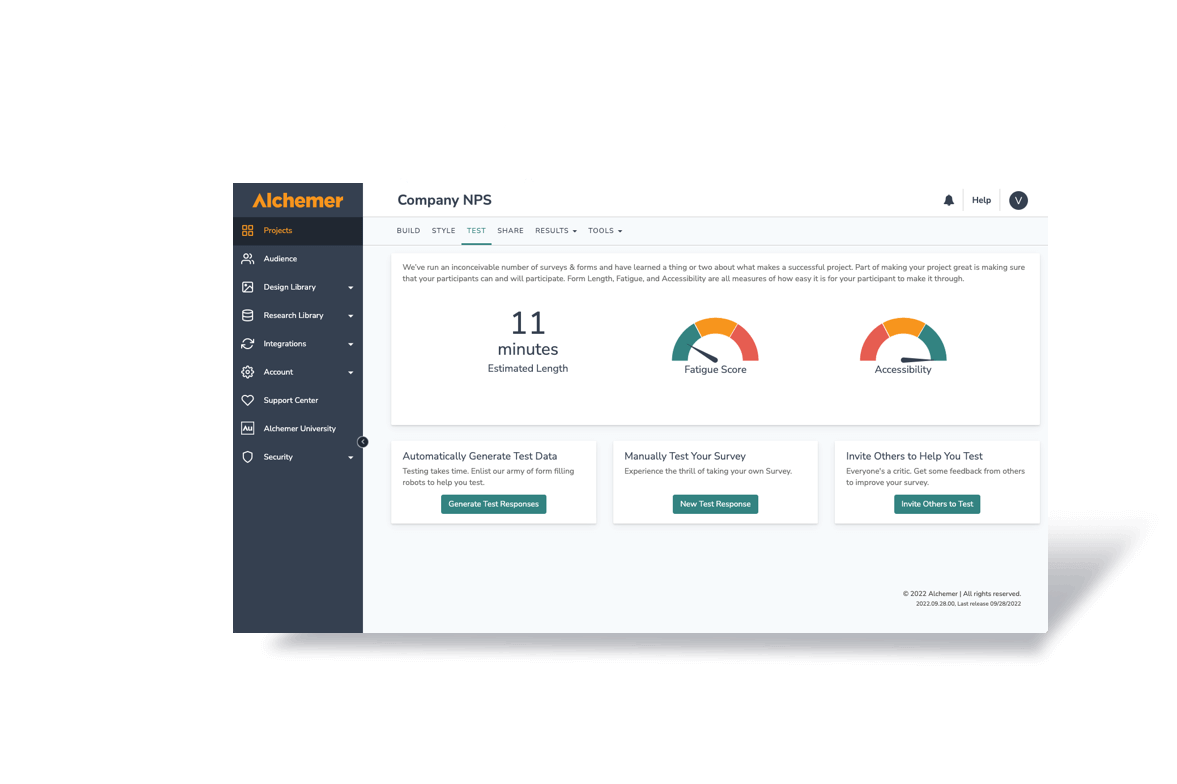
See all blog posts >
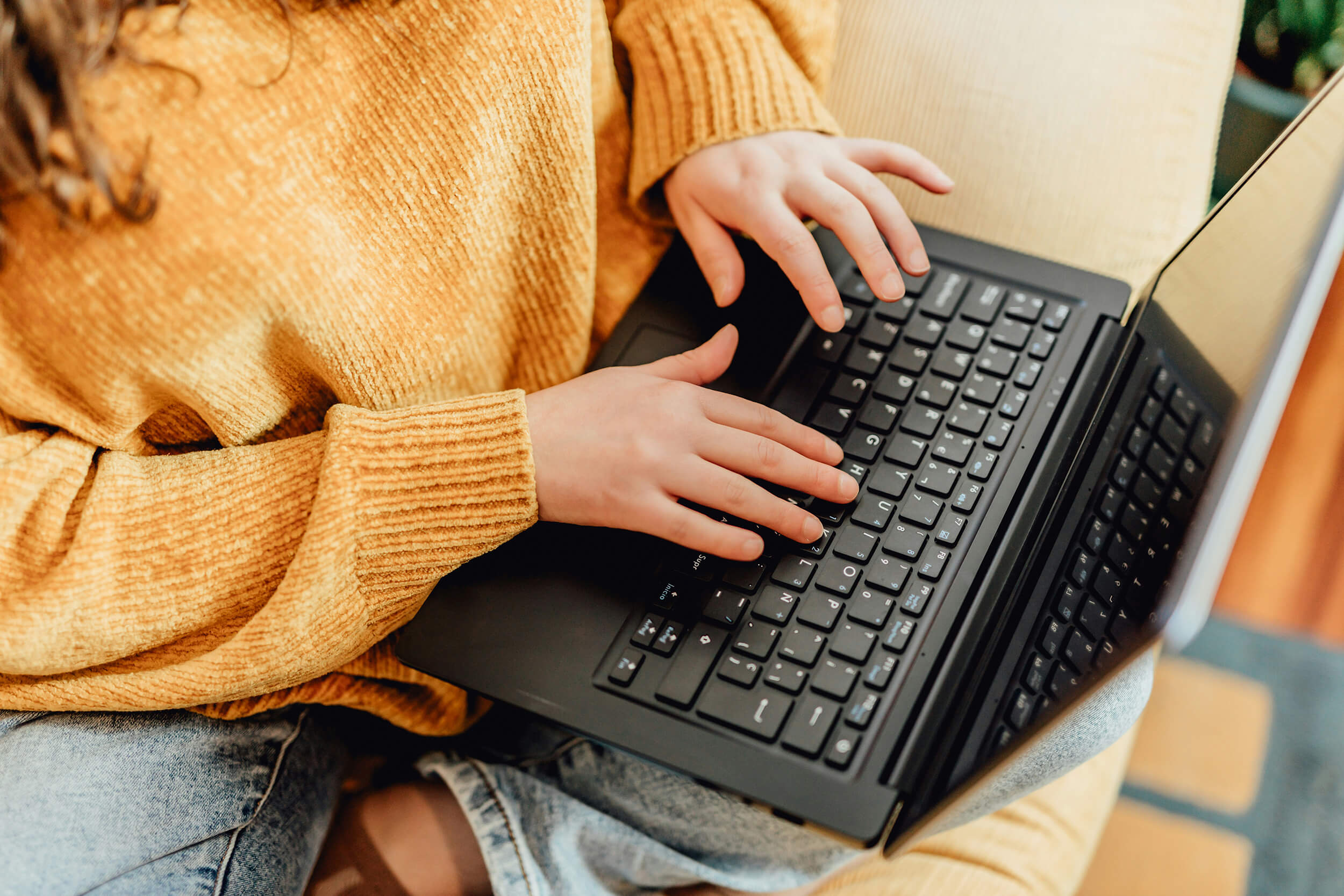
- Alchemer Survey , Survey Tips
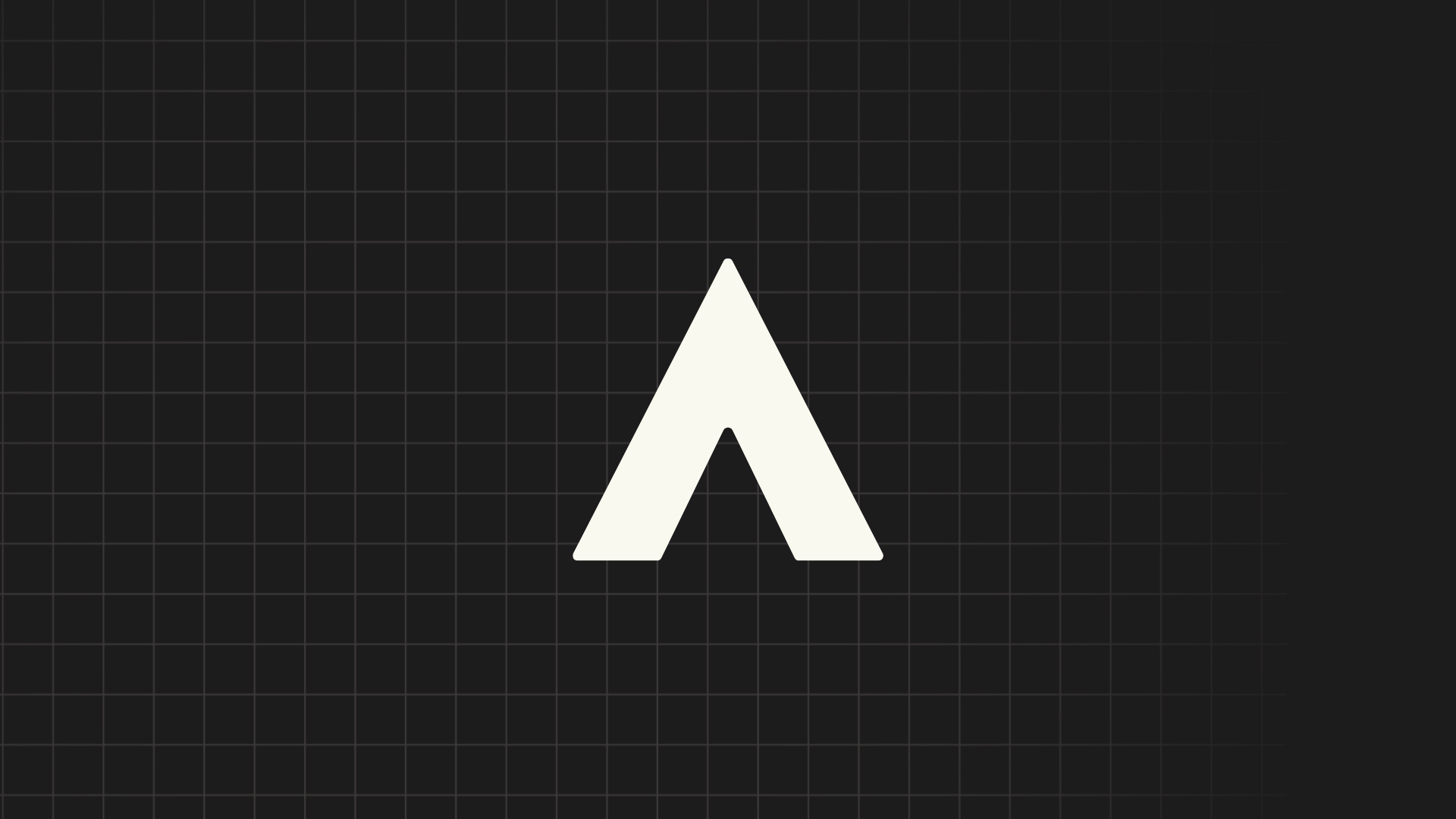
- Company News , Press Release
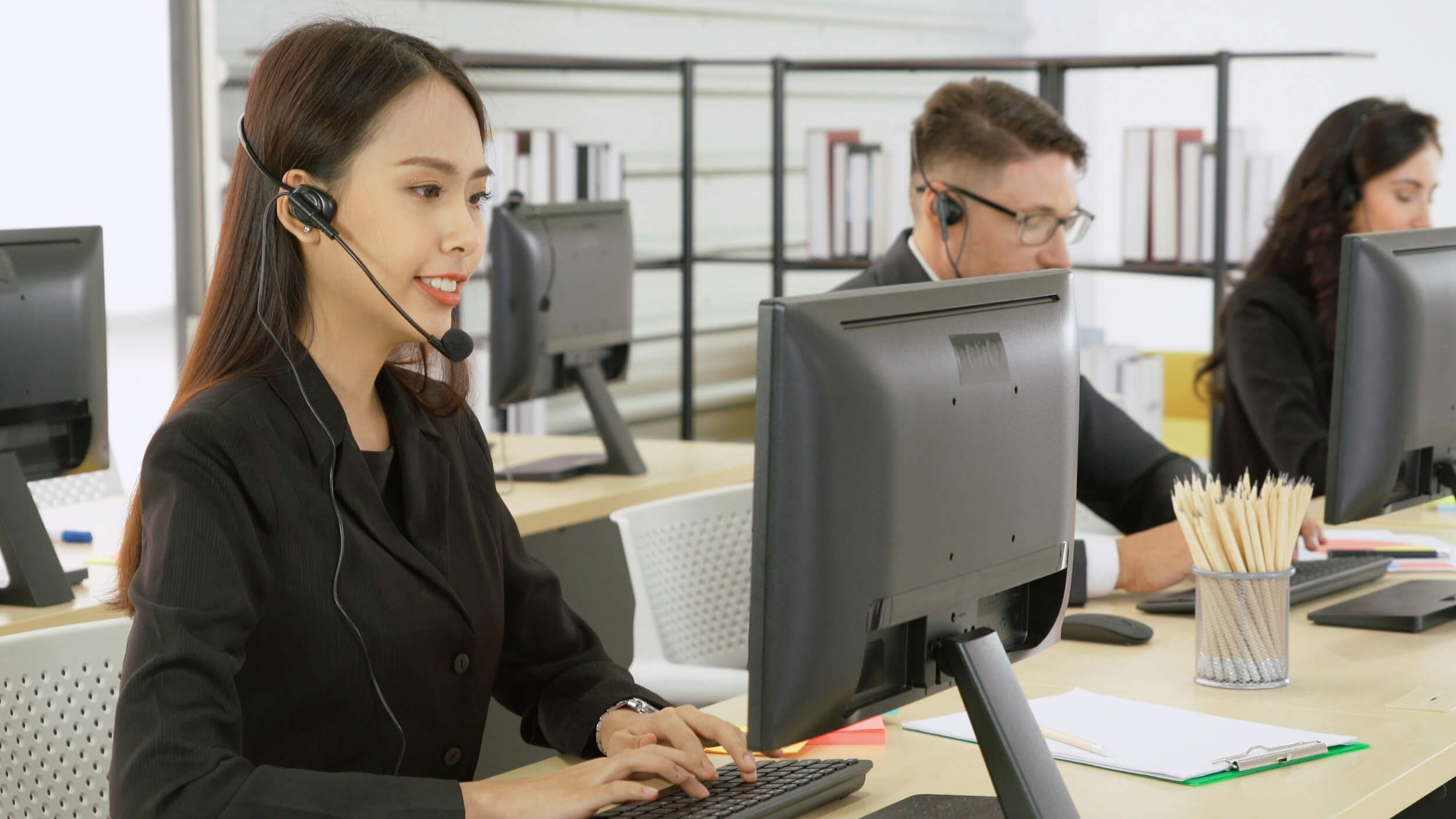
- Customer Experience , Customer Feedback , Sentiment analysis , Text analytics
See it in Action

- Privacy Overview
- Strictly Necessary Cookies
- 3rd Party Cookies
This website uses cookies so that we can provide you with the best user experience possible. Cookie information is stored in your browser and performs functions such as recognising you when you return to our website and helping our team to understand which sections of the website you find most interesting and useful.
Strictly Necessary Cookie should be enabled at all times so that we can save your preferences for cookie settings.
If you disable this cookie, we will not be able to save your preferences. This means that every time you visit this website you will need to enable or disable cookies again.
This website uses Google Analytics to collect anonymous information such as the number of visitors to the site, and the most popular pages.
Keeping this cookie enabled helps us to improve our website.
Please enable Strictly Necessary Cookies first so that we can save your preferences!
Application of Factor Analysis: To Study the Factor Structure Among Variables
- First Online: 18 October 2012
Cite this chapter
- J. P. Verma 2
7079 Accesses
2 Citations
Buying decision of an individual depends upon large number of product characteristics. But the market strategy cannot be developed on the basis of all those parameters of a product that affect the buying behavior of an individual. The factor analysis, a multivariate technique, comes to our rescue in solving such problems. The factor analysis technique reduces the large number of variables into few underlying factors to explain the variability of the group characteristics. The concept used in factor analysis technique is to investigate the relationship among the group of variables and segregate them in different factors on the basis of their relationship. Thus, each factor consists of those variables which are related among themselves and explain some portion of the group variability. For example, personality characteristics of an individual can be assessed by the large number of parameters. The factor analysis may group these variables into different factors where each factor measure some dimension of personality characteristics. Factors are so formed that the variables included in it are related with each other in some way. The significant factors are extracted to explain the maximum variability of the group under study.
This is a preview of subscription content, log in via an institution to check access.
Access this chapter
Subscribe and save.
- Get 10 units per month
- Download Article/Chapter or eBook
- 1 Unit = 1 Article or 1 Chapter
- Cancel anytime
- Available as PDF
- Read on any device
- Instant download
- Own it forever
- Available as EPUB and PDF
- Compact, lightweight edition
- Dispatched in 3 to 5 business days
- Free shipping worldwide - see info
- Durable hardcover edition
Tax calculation will be finalised at checkout
Purchases are for personal use only
Institutional subscriptions
Similar content being viewed by others
Factor Analysis
Second Order Confirmatory Factor Analysis: An Example
Common factors, author information, authors and affiliations.
Research and Advanced Studies, Lakshmibai National University of Physical Education, Gwalior, MP, India
J. P. Verma
You can also search for this author in PubMed Google Scholar
Corresponding author
Correspondence to J. P. Verma .
Rights and permissions
Reprints and permissions
Copyright information
© 2013 Springer India
About this chapter
Verma, J.P. (2013). Application of Factor Analysis: To Study the Factor Structure Among Variables. In: Data Analysis in Management with SPSS Software. Springer, India. https://doi.org/10.1007/978-81-322-0786-3_11
Download citation
DOI : https://doi.org/10.1007/978-81-322-0786-3_11
Published : 18 October 2012
Publisher Name : Springer, India
Print ISBN : 978-81-322-0785-6
Online ISBN : 978-81-322-0786-3
eBook Packages : Mathematics and Statistics Mathematics and Statistics (R0)
Share this chapter
Anyone you share the following link with will be able to read this content:
Sorry, a shareable link is not currently available for this article.
Provided by the Springer Nature SharedIt content-sharing initiative
- Publish with us
Policies and ethics
- Find a journal
- Track your research
What is factor analysis?
Factor analysis is a statistical technique that reduces a set of variables by extracting all their commonalities into a smaller number of factors. It can also be called data reduction.
When observing vast numbers of variables, some common patterns emerge, which are known as factors. These serve as an index of all the variables involved and can be utilized for later analysis.
Factor analysis uses several assumptions:
- The variables’ linear relationships
- Absence of multicollinearity
- Relevance of the variables
- The existence of a true correlation between factors and variables
Therefore, it becomes a statistical technique used to see how a group shares a common variance. While it is mostly used in psychological research, it can also be applied in areas like business and market study to understand customer satisfaction or employee job satisfaction and in finance, to study the fluctuation of stock prices.
Features of factor analysis
While studying customer satisfaction related to a product, a researcher will usually pose several questions about the product through a survey. These questions will consist of variables regarding the product’s features, ease of purchase, usability, pricing, visual appeal, and so forth. These are typically quantified on a numeric scale. But, what a researcher looks for is the underlying dimensions or “factors” regarding customer satisfaction. These are mostly psychological or emotional factors toward the product that cannot be directly measured. Factor analysis uses the variables from the survey to determine them indirectly.
When a researcher assumes these variables from the survey, they are condensed into one or more factors. Some of the methods used to extract these factors could include:
Principal component analysis
This is the most commonly used method. The first factor is extracted by determining the maximum variance. This variance is then removed and is replaced by the factor. The second factor is then determined by the next highest variance, and the process continues until there are no more variances.
Common factor analysis
In this method, the factors are extracted from commonly-occurring variances and do not include the unique variances of all the variables.
Image factoring
Based on the correlation matrix, this process uses predicted variables using the OLS regression method.
Once the factors are extracted, the questionnaire’s score is assumed to be related to the factors in a linear manner. The margin of error is also taken into consideration, along with all the factors to the equation.
Types of factor analysis
There are essentially two types of factor analysis:
- Exploratory Factor Analysis: In exploratory factor analysis, the researcher does not make any assumptions about prior relationships between factors. In this method, any variable can be related to any factor. This helps identify complex relationships among variables and group them based on common factors.
- Confirmatory Factor Analysis: The confirmatory factor analysis, on the other hand, assumes that variables are related to specific factors and uses pre-established theory to confirm its expectations of the model.
Assumptions of factor analysis
Factor analysis makes use of several assumptions in order to produce the outcomes:
- There will not be any outliers in the data.
- The sample size will be greater than the size of the factor.
- Since the method is interdependent, there will be no perfect multicollinearity between any of the variables.
- When in a sequence of random variables, all the variables have the same finite variance, known as being homoscedastic. Since factor analysis works as a linear function, it will not need homoscedasticity between variables.
- There is the assumption of linearity. This means that even non-linear variables can be used, but once transferred, they become linear variables.
- There is also the assumption of interval data.
How factor analysis is used
Business marketing.
In a business model, factor analysis is used to explain complex variables or data using the matrix of association. It studies the interdependencies of data and assumes that complex variables can be reduced to a few important dimensions. This is possible because of some of the relationships between variables and their dimensions. The attribute of one variable might sometimes be the result of the dimension of another. It breaks down the initial rating, using statistical algorithms on various components and uses these partial scores to extract various factors.
Automotive industry
The use of factor analysis in the automotive industry was mentioned as far back as 1997 in an article by Professor Emeritus Richard B. Darlington of Cornell University. He explained how a study could be used to identify all the variables that apply to the decision-making of purchasing a car—size, pricing, options, accessories, and more. The study could then be used to arrive at a few key variables that actually close a purchase decision. Automotive dealers can then tailor their offerings to cater to the market.
The key to a productive investment portfolio is diversification. To ensure a diverse portfolio, investment professionals use factor analysis to predict movement across a wide sector of industries and provide insights on factors that may be under the radar. For example, the average portfolio contains stocks of industries like technology and commodities. A look at the rise in stock prices of a related industry, like oil, will give investment professionals a good idea on what to sell and retain.
Human resources
There are many factors that go into a company’s hiring process. With statistics, human resource professionals will be able to create a comfortable and productive working environment. Several variables can be compared and analyzed to see which combination in terms of the number of team members, varied skill sets, and contractual or in-house talent works, improving the overall functioning of the organization.
Restaurants
For restaurants, factor analysis can be used to understand demographics and target diners in the creation of menus. A fast-food restaurant opening next to a university campus will have to plan its menu differently than if it was placed in a high-end shopping location. Factors such as surrounding competition, foot-traffic, age-groups, and location all determine success.
When hiring teachers and deciding on a curriculum for the school year, factor analysis plays a huge role. It is used to determine classroom sizes, staffing limits, salary distribution, and a wide range of other requirements necessary for the school year to run smoothly.
Challenges and solutions of factor analysis
While factor analysis is a useful tool for business research and analysis, there are a few challenges to keep in mind in order to ensure the right results. The result entirely depends on the ability of the researcher to gather the right set of variables associated with the business and the product. Neglecting even a small detail might result in the wrong value of the procedure.
If the observed variables for a particular item are similar to each other but distinct from other items, the algorithm might consider this as a single factor to those items, which could result in inaccurate analysis results. Knowledge of the item and its theory is important in naming factors accurately. Even dissimilar variables might have dependencies for no reason.
Gathering information using an accurate survey is the key. Besides the knowledge of the product and its theory, it is also important to know its market.
Research and developments in the field of factor analysis continue to contribute to making more informed decisions in various sectors. Continuous refinement in confirmatory factor analysis techniques makes this one of the most important decision-making tools for every industry in the future.
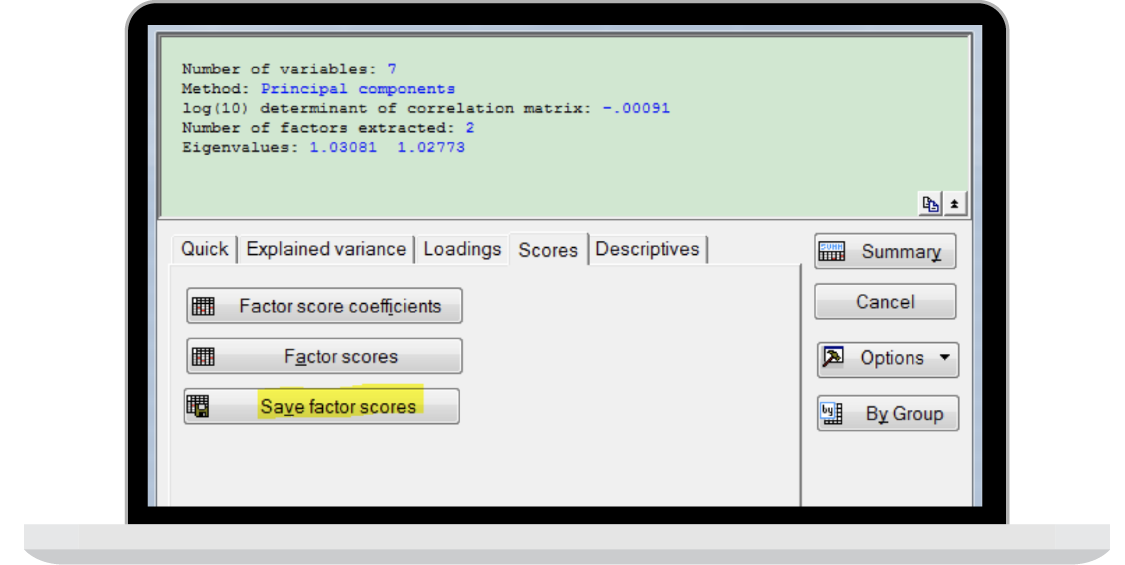
Ready for immersive, real-time insights for everyone?
- Sign-up for a free trial
- Book a demo
3 Types of Factor Analysis
Factor analysis is a statistical technique used to identify the underlying structure of a set of variables.
Market research and analysis of large volumes of data are necessary when it comes to analyzing and determining the right market segment, potential demand, and potential areas of competition, product development requirements and all other facets of the business marketing portfolio. One of the most common tools used to deal with the vast amounts of data is Factor Analysis.
What is Factor Analysis?
Factor analysi s is a statistical technique used to identify the underlying structure of a set of variables. In layman’s terms, it is used to analyze the relationship between two observable variables and how it is affected by another smaller set of unobservable variables. For example, factor analysis can be used in market segmentation to identify the underlying variables according to which customers can be grouped.
Uses of factor analysis in market research and analysis
Factor analysis has proved to be very beneficial in market research and analysis of variables that determine consumer behavior:
- It helps to make sense of large data with interlinked relationships
- It may point out relationships that may not have been obvious
- It can point out to the underlying relationships with respect to consumer tastes, preferences, etc.
- It is easier to condense and correlate data through factor analysis and also to draw conclusions from the data gathered in market research and analysis.
- It can be used to form empirical clusters of variables and underlying factors that affect them
Types of factor analysis
A factor analysis is mainly used for interpretation of data and in analyzing the underlying relationships between variable and other underlying factors that may determine consumer behavior. Instead of grouping responses and response types, factor analysis segregates the variable and groups these according to their co relevance.There are mainly three types of factor analysis that are used for different kinds of market research and analysis.
- Exploratory factor analysis
- Confirmatory factor analysis
- Structural equation modeling
Exploratory factor analysis is used to measure the underlying factors that affect the variables in a data structure without setting any predefined structure to the outcome. Confirmatory factor analysis on the other hand is used as tool in market research and analysis to reconfirm the effects and correlation of an existing set of predetermined factors and variables that affect these factors. Structural equation modeling hypothesizes a relationship between a set of variables and factors and tests these casual relationships on the linear equation model. Structural equation modeling can be used for exploratory and confirmatory modeling alike, and hence it can be used for confirming results as well as testing hypotheses.
Factor analysis will only yield accurate and useful results if done by a researcher who has adequate knowledge to select data and assign attributes. Selecting factors and variables so as to avoid too much similarity of characteristics is also important. Factor analysis, if done correctly, can allow for market research and analysis that helps in various areas of decision making like product features, product development, pricing, market segmentation, penetration and even with targeting.
Applications of Factor Analysis
Factor analysis has several applications in different fields, including:
- Market Research: it is widely used in market research to identify the underlying factors that influence customer preferences and behavior. For example, a market research study may use factor analysis to identify the key factors that influence consumers' purchasing decisions.
- Psychology: it is used in psychology to identify the underlying dimensions of personality traits, intelligence, and cognitive abilities. For example, a factor analysis of a personality test may identify factors such as extraversion, neuroticism, and openness to experience.
- Education: it is used in education to identify the underlying factors that contribute to academic achievement. For example, a factor analysis of academic test scores may identify factors such as verbal reasoning, mathematical ability, and spatial ability.
Factor analysis is a powerful statistical technique that can help in identifying the underlying factors that contribute to the patterns of the data. By reducing large amounts of data into a smaller number of factors, it can simplify the data and help in making meaningful conclusions. Whether you're conducting market research, psychological studies, or educational research, factor analysis can be a valuable tool for understanding the relationships between variables and identifying the underlying factors that explain the patterns in the data.
Recent articles
mTab and Kayne Anderson Partner to Further Innovate Insights
The Key to Streaming Show Loyalty is Audience Insights
Which QSR Tops America’s “Fast Food Favorites” Ranking?
Ready to take the next step with the world’s #1 ai ims.
Make smarter decisions faster with the world's #1 Insight Management System.

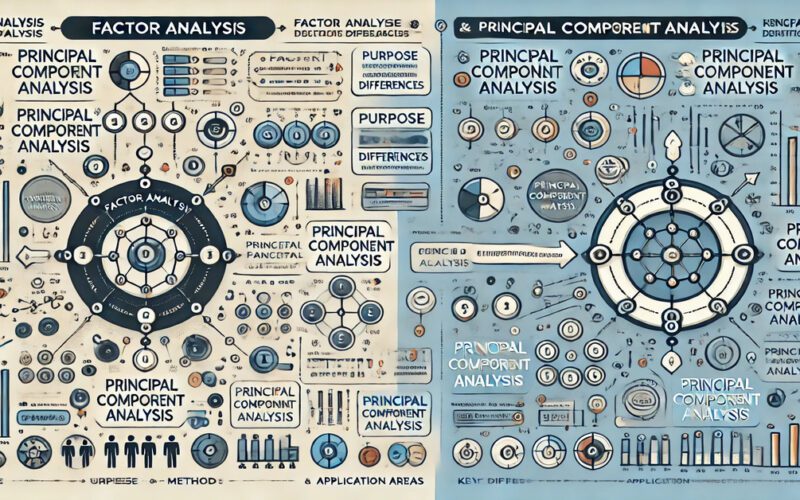
Factor Analysis VS Principal Component Analysis: Crucial Differences
- 9 minute read
- September 23, 2024

Written by:

Aashi Verma has dedicated herself to covering the forefront of enterprise and cloud technologies. As an Passionate researcher, learner, and writer, Aashi Verma interests extend beyond technology to include a deep appreciation for the outdoors, music, literature, and a commitment to environmental and social sustainability.
Summary : Factor Analysis uncovers latent variables that explain correlations, while Principal Component Analysis reduces data dimensionality, preserving variance. Understanding their differences helps you choose the right tool for identifying hidden factors or simplifying data in fields like psychology, market research, genomics, and finance.
Introduction
Factor Analysis (FA) and Principal Component Analysis (PCA) are powerful statistical techniques use for Data Analysis . While both methods aim to simplify complex datasets, they serve distinct purposes.
Factor Analysis seeks to identify underlying factors that explain observed correlations among variables, whereas Principal Component Analysis focuses on reducing the dimensionality of data while preserving variance.
Understanding the differences between FA and PCA is crucial for selecting the appropriate method for your analysis needs. This blog will explore “Factor Analysis vs Principal Component Analysis,” highlighting their key distinctions and helping you choose the right approach for your Data Analysis objectives.
What is Factor Analysis?
Factor Analysis (FA) is a statistical technique identifying underlying relationship between variables. It uncovers latent factors or constructs that explain observed correlations among variables. By reducing data complexity, FA simplifies understanding of how variables relate to one another through fewer underlying factors.
Objectives and Goals
The primary objective of Factor Analysis is to reveal hidden structures within data by grouping correlated variables into factors. These factors represent underlying dimensions that account for the patterns of correlations observed among the original variables. FA aims to:
- Simplify Data : Reduce the number of variables to a smaller set of factors, making the data more manageable and interpretable.
- Identify Underlying Constructs : Discover latent variables that drive the relationships between observed variables, providing insights into the data’s fundamental dimensions.
- Enhance Theoretical Understanding : Uncover the constructs that explain patterns within the data to support theory development. This can guide further research or refinement of theoretical models.
Common Use Cases and Applications
Factor Analysis provides valuable insights by uncovering the hidden structure in complex data, making it a powerful tool for data reduction and interpretation. Factor Analysis widely used across various fields for different purposes:
- Psychology : In psychological research, FA helps identify underlying traits or factors, such as personality dimensions or cognitive abilities, from questionnaire responses.
- Market Research : Businesses use FA to segment consumers based on underlying preferences or behaviours, allowing for more targeted marketing strategies.
- Health Sciences : Researchers apply FA to understand the underlying factors influencing health outcomes or symptoms, aiding in the development of more effective treatments.
- Education : Educators use FA to analyse student performance and identify critical factors affecting learning outcomes, helping to improve educational strategies.
Read Blog: Statistical Tools for Data-Driven Research .
What is Principal Component Analysis?
Principal Component Analysis (PCA) is a dimensionality reduction technique that transforms a set of possibly correlated variables into a set of linearly uncorrelated variables called principal components.
These components capture the maximum variance present in the original data, with the first component accounting for the largest amount of variance, the second component the next largest, and so on. PCA helps simplify complex datasets by reducing their dimensions while retaining the most critical information.
The primary objective of PCA is to simplify data by reducing its dimensions without losing significant information. The goals of PCA include:
- Dimensionality Reduction : PCA reduces the number of variables in a dataset while preserving as much variance as possible. This makes data easier to visualise and analyse without losing significant information.
- Data Compression : PCA compresses the data by focusing on the most significant principal components, leading to more efficient storage and processing.
- Noise Reduction : PCA filters out noise and less relevant information by concentrating on components that explain the most variance, enhancing the signal in the data.
- Feature Extraction : PCA helps identify and extract the most influential features from a dataset, which can improve the performance of Machine Learning models.
Principal Component Analysis is a valuable tool for simplifying complex datasets, enhancing Data Analysis, and improving model efficiency. Principal Component Analysis finds applications across various fields:
- Finance : Analysts use PCA to identify key factors driving stock market movements and to construct risk models.
- Image Processing : PCA employed in image compression and recognition , reducing the dimensionality of image data while preserving important features.
- Genomics : Researchers apply PCA to analyse gene expression data, uncovering patterns and reducing dimensionality for more manageable data interpretation.
- Marketing : PCA helps businesses segment their customers by identifying key patterns in customer behaviour and preferences, aiding in targeted marketing strategies.
Explore More About: An Introduction to Statistical Inference .
Key Differences Between Factor Analysis and Principal Component Analysis
Understanding the distinctions between Factor Analysis (FA) and Principal Component Analysis (PCA) is crucial when examining statistical analysis. Both techniques simplify complex data, but they serve different purposes and employ different methodologies.
This section explores the key differences between FA and PCA across several dimensions: purpose and objectives, methodology, interpretability, assumptions, and factor versus component scores.
Purpose and Objectives
Factor Analysis aims to uncover the underlying relationships between variables. It identifies latent factors or constructs that explain the correlations observed among variables.
For instance, in psychology, FA might reveal underlying personality traits that influence responses to various questions on a questionnaire. The primary objective of FA is to reduce data complexity by grouping variables into factors that reflect the same underlying construct.
In contrast, PCA focuses on reducing the dimensionality of data while preserving as much variance as possible. It transforms original variables into a new set of uncorrelated variables called principal components.
These components capture the directions of maximum variance in the data. PCA is typically use to compress data, simplify models, or visualise data while retaining key information.
Methodology
FA is based on correlation matrices and uses extraction methods to identify factors. It starts by examining the correlation between variables and then extracts factors that explain these correlations.
The method involves techniques such as Maximum Likelihood Estimation or Principal Axis Factoring. The goal is to determine how many factors needed to explain the patterns of correlations among the variables.
PCA, on the other hand, relies on the eigenvalues and eigenvectors of the data’s covariance matrix. It computes principal components by performing an eigendecomposition of the covariance matrix, which reveals the directions of maximum variance.
Each principal component is a linear combination of the original variables, and the first few components usually capture most of the data’s variance. PCA is purely a mathematical approach focused on variance and does not assume an underlying model.
Interpretability
FA provides factors that can be interpreted as underlying variables or constructs. For example, a factor might represent an underlying dimension, such as “job satisfaction”, derived from multiple survey questions. These factors are often used to make sense of complex data by providing a more meaningful representation of relationships between variables.
PCA produces principal components that are linear combinations of the original variables. While PCA helps reduce data complexity, the principal components are not always easily interpretable. They are mathematical constructs rather than concepts tied to specific latent variables.
For instance, the first principal component might be a blend of various original variables, which can sometimes make its interpretation less straightforward.
Assumptions
FA assumes the presence of latent variables that influence the observed variables. It operates on the premise that the observed variables are manifestations of underlying factors. This approach assumes a model where variables are interrelated through these latent constructs, and the goal is to uncover this underlying model.
PCA assumes linearity in the data and focuses primarily on variance. It does not assume any underlying latent structure but seeks to explain the variability in the data through principal components. The technique is more concerned with representing data in fewer dimensions while maintaining as much variance as possible.
Factor vs. Component Scores
In FA, factor scores reflect the degree to which each observation exhibits the underlying latent constructs. These scores help understand how strongly each factor influences the observations and can be utilised in further analysis or prediction.
PCA provides component scores that represent the projection of the original data onto the principal components. These scores indicate how much of each element is present in the observations and help understand data distribution across the principal components.
Understanding these differences helps select the appropriate method based on the research goals, whether to uncover latent constructs with FA or reduce dimensionality and preserve variance with PCA. Each technique offers unique insights and benefits depending on the analysis context.
Discover: Different Types of Statistical Sampling in Data Analytics .
When Should we Use Factor Analysis vs. Principal Component Analysis?
Despite their similarities, Factor Analysis (FA) and Principal Component Analysis (PCA) are two powerful statistical techniques used for different purposes. Knowing when to use one over the other depends on your research goals.
Factor Analysis is primarily concerned with identifying underlying relationships among variables, while PCA focuses on reducing the dimensionality of data by summarising it into principal components. Below, we explore the ideal scenarios for using FA and PCA and examples of how each can be applied effectively.
Factor Analysis: Ideal Scenarios
Factor Analysis is ideal when you aim to discover the underlying constructs or latent variables that explain patterns in your data. It is commonly used in social sciences and psychology to reduce large variables into more meaningful factors representing broader concepts.
For example, when analysing survey data on job satisfaction, you might find that multiple questions measuring work-life balance, salary, and relationships with colleagues load onto broader factors such as “job fulfilment” and “organisational culture.”
In this scenario, Factor Analysis helps researchers understand which factors most significantly contribute to the broader concept of job satisfaction, reducing the complexity of interpreting many individual variables.
Unlike PCA, which focuses on maximising variance, FA uncovers hidden data structures that are not immediately observable. This is particularly useful when you aim to build a model based on latent variables rather than simply reducing data.
Factor Analysis is also frequently applied in psychometric testing, where the goal is to assess the reliability and validity of tests measuring psychological traits such as intelligence, personality, or behaviour. In psychometrics, researchers often use FA to ensure that different items on a questionnaire or test measure the same construct.
For instance, in a personality test designed to measure traits such as extraversion and conscientiousness, FA can determine whether the items meant to measure these traits are correlated and load onto distinct factors. This helps validate that the test accurately measures the intended personality dimensions and is reliable for further study.
Factor Analysis is particularly beneficial when you expect your variables to be interrelated but want to reduce the noise in your data by isolating the most significant factors driving your results.
Principal Component Analysis: Ideal Scenarios
Principal Component Analysis is best suited for situations where you need to reduce the dimensionality of a large dataset while preserving as much of the original variance as possible.
In fields like genetics, marketing, or finance, datasets often contain hundreds or thousands of variables, making interpreting or visualising the data challenging. PCA condenses these variables into smaller uncorrelated principal components summarising the original data.
For example, in a genetic study analysing thousands of genetic markers, PCA can reduce the dataset to a few principal components that explain most of the variance in the data. This enables researchers to analyse and visualise the data without being overwhelmed by the sheer number of variables.
While PCA doesn’t uncover latent factors like FA, it efficiently reduces data complexity, making it easier to interpret and analyse large datasets.
PCA is the go-to method when your primary goal is data compression without losing much information, especially when dealing with high-dimensional datasets.
PCA is also commonly used in exploratory Data Analysis (EDA) when the aim is to detect patterns and relationships between variables before building more complex models. By identifying the principal components that explain the most variance in the dataset, PCA helps researchers understand the underlying structure of the data.
For example, in market research, PCA can reveal the key factors influencing customer preferences or behaviours from a large dataset of survey responses. Once the principal components identified, researchers can focus on them for deeper analysis, such as clustering customers based on their preferences.
PCA is beneficial for exploratory purposes because it provides a simple and interpretable way to visualise high-dimensional data. By projecting the data onto the first two or three principal components, researchers can create scatter plots that reveal the data’s groupings, trends, or outliers. These insights can then guide the next steps in the research process.
Further Read About: Exploring The Top Key Statistical Concepts .
Factor Analysis (FA) and Principal Component Analysis (PCA) are valuable tools for simplifying complex data but serve distinct purposes. FA identifies hidden relationships between variables by uncovering latent factors, while PCA reduces the dimensionality of data, preserving variance through principal components.
Selecting the correct method depends on your analysis objectives—FA is ideal for exploring underlying constructs, whereas PCA excels in dimensionality reduction. Both techniques offer powerful insights for improving Data Analysis, ensuring that you can draw meaningful conclusions and enhance model performance based on the nature of your dataset.
Frequently Asked Questions
What is the main difference between factor analysis and principal component analysis.
Factor Analysis uncovers latent factors that explain correlations among variables, while Principal Component Analysis reduces dimensionality by transforming data into uncorrelated principal components. FA focuses on underlying structures, while PCA preserves variance in the dataset.
When Should You Use Factor Analysis vs Principal Component Analysis?
Use Factor Analysis to identify hidden factors influencing correlations between variables. Choose Principal Component Analysis to reduce data dimensionality while preserving as much variance as possible for more efficient analysis and visualisation.
What are the Key Applications of Factor Analysis and Principal Component Analysis?
Factor Analysis used in psychology, market research, and education to identify underlying constructs. Principal Component Analysis widely used in finance, genomics, and image processing to reduce data complexity and improve visualisation and model efficiency.
Reviewed by:

Post written by: Aashi Verma
Data Science Learn the Basics of Linear Algebra For Data Science
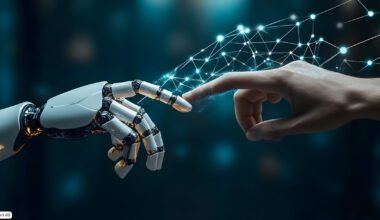
Artificial Intelligence Understanding the Synergy Between Artificial Intelligence & Data Science
You may also like.
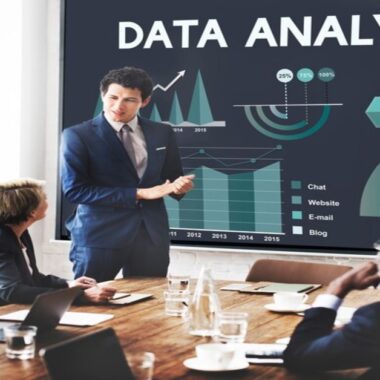
- Data Analysts
Top Data Analytics Projects in 2024 for Beginners to Experienced
- July 20, 2023
- 7 minute read
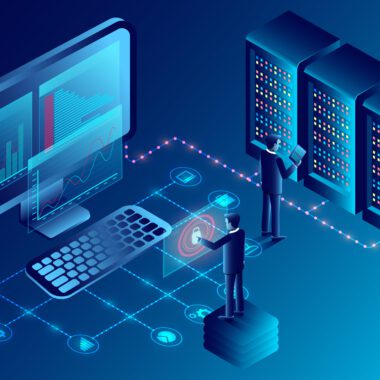
- Data Science
A Deep Dive into Data Mining
- February 21, 2023
- 6 minute read

COMMENTS
Factor analysis is a statistical technique that identifies the underlying structure of a set of variables and explains them in terms of common factors. Learn the general steps, types, formulas and examples of factor analysis for research.
Factor analysis isn't a single technique, but a family of statistical methods that can be used to identify the latent factors driving observable variables. Factor analysis is commonly used in market research, as well as other disciplines like technology, medicine, sociology, field biology, education, psychology and many more.
Factor analysis in market research is a statistical method used to uncover underlying dimensions, or factors, in a dataset. By examining patterns of correlation between variables, factor analysis helps to identify groups of variables that are highly interrelated and can be used to explain a common underlying theme.
Factor analysis is a statistical technique that groups variables by their similarity or difference. It is used in marketing research to identify underlying dimensions, test hypotheses and shorten questionnaires.
Learn what factor analysis is, how it can help identify hidden factors, and how to perform it in psychology and other fields. This guide covers the goals, methods, and steps of factor analysis with an example.
When it comes to Factor Analysis in marketing, one can benefit immensely from this statistical method. In order to boost marketing campaigns and accelerate success, in the long run, companies employ Factor Analysis techniques that help to find a correlation between various variables or factors of a marketing campaign. ... While data research ...
Explain how factor analysis can be used in the context of a successful marketing research plan. Factor analysis can play a crucial role in a marketing research plan by helping researchers uncover the underlying dimensions or factors that drive consumer behavior, attitudes, and perceptions. By identifying the latent variables that influence key ...
The integration of factor analysis into market research involves a systematic process, encompassing seven key steps that pave the way for valuable insights: Data preparation Begin by collecting comprehensive datasets, ensuring that the data is normalized for effective comparison. The choice between exploratory and confirmatory factor analysis ...
Factor Analysis ExpressesPerson OthersOpinion TellsAbout MatchImage InvestigateDepth LearnAboutOptions LookFeatures SomeAreBetter NotImportant NeverThink VeryInterested MR1 0.7 0.7 0.7 0.6 MR2 0.7 0.7 0.6 0.5 0.7 MR3 0.6 0.5
Factor analysis is a powerful data reduction technique that enables researchers to investigate concepts that cannot easily be measured directly. By boiling down a large number of variables into a handful of comprehensible underlying factors, factor analysis results in easy-to-understand, actionable data.
In marketing research, application of factor analysis provides very useful inputs to the decision makers to focus on few factors rather than a large number of parameters in making their products more acceptable in the market. ... salary, exposure to product advertisement, and availability are responsible. Factor analysis may help the market ...
Factor analysis is a statistical method to describe variability among observed variables in terms of unobserved factors. Learn the mathematical model, the example of intelligence factors, and the advantages and disadvantages of factor analysis.
How factor analysis is used Business marketing. ... Besides the knowledge of the product and its theory, it is also important to know its market. Research and developments in the field of factor analysis continue to contribute to making more informed decisions in various sectors. Continuous refinement in confirmatory factor analysis techniques ...
Exploratory factor analysis (EFA) is one of a family of multivariate statistical methods that attempts to identify the smallest number of hypothetical constructs (also known as factors, dimensions, latent variables, synthetic variables, or internal attributes) that can parsimoniously explain the covariation observed among a set of measured variables (also called observed variables, manifest ...
1 In conducting this analysis, we rescaled significant outliers for all three variables similar to the approach described above. Specifically, we calculated z-scores and rescaled large values to the next highest typical value based on the distribution of observations. This included rescaling six sample sizes to a value of 13,327 (z-score = 2.89), five numbers of indicators to a value of 93 (z ...
factor analysis in the marketing community that even its defenders and some prominent reviewers perpet-uate misinformation. Many reviewers have discussed the use of factor analysis as a clustering procedure, at best an extreme perversion of the method. The alleged subjectivity of the technique is part of the folklore of the discipline.
Factor analysis has several applications in different fields, including: Market Research: it is widely used in market research to identify the underlying factors that influence customer preferences and behavior. For example, a market research study may use factor analysis to identify the key factors that influence consumers' purchasing decisions.
The use of factor analysis as a method for examining the dimensional structure of data is contrasted with its frequent misapplication as a tool for identifying clusters and segments. ... The Application and Misapplication of Factor Analysis in Marketing Research Show all authors. David W. Stewart. David W. Stewart. Owen Graduate School of ...
PDF | On Jun 30, 1971, Jagdish N. Sheth published Factor Analysis in Marketing Research | Find, read and cite all the research you need on ResearchGate
The use of factor analysis as a method for examining the dimensional structure of data is contrasted with its frequent misapplication as a tool for identifying clusters and segments. ... The Application and Misapplication of Factor Analysis in Marketing Research. David W. Stewart View all authors and affiliations. Volume 18, Issue 1. https ...
Summary: Factor Analysis uncovers latent variables that explain correlations, while Principal Component Analysis reduces data dimensionality, preserving variance.Understanding their differences helps you choose the right tool for identifying hidden factors or simplifying data in fields like psychology, market research, genomics, and finance.
Stewart D. W. (1981), "The Application and Misapplication of Factor Analysis in Marketing Research," Journal of Marketing Research, 18 (February), 51-62. Crossref Google Scholar
The use of factor analysis as a method for examining the dimensional structure of data is contrasted with its frequent misapplication as a tool for identifying clusters and segments. ... The Application and Misapplication of Factor Analysis in Marketing Research. David W. Stewart View all authors and affiliations. Volume 18, Issue 1. https ...