- Voxco Online
- Voxco Panel Management
- Voxco Panel Portal
- Voxco Audience
- Voxco Mobile Offline
- Voxco Dialer Cloud
- Voxco Dialer On-premise
- Voxco TCPA Connect
- Voxco Analytics
- Voxco Text & Sentiment Analysis

- 40+ question types
- Drag-and-drop interface
- Skip logic and branching
- Multi-lingual survey
- Text piping
- Question library
- CSS customization
- White-label surveys
- Customizable ‘Thank You’ page
- Customizable survey theme
- Reminder send-outs
- Survey rewards
- Social media
- Website surveys
- Correlation analysis
- Cross-tabulation analysis
- Trend analysis
- Real-time dashboard
- Customizable report
- Email address validation
- Recaptcha validation
- SSL security
Take a peek at our powerful survey features to design surveys that scale discoveries.
Download feature sheet.
- Hospitality
- Academic Research
- Customer Experience
- Employee Experience
- Product Experience
- Market Research
- Social Research
- Data Analysis
Explore Voxco
Need to map Voxco’s features & offerings? We can help!
Watch a Demo
Download Brochures
Get a Quote
- NPS Calculator
- CES Calculator
- A/B Testing Calculator
- Margin of Error Calculator
- Sample Size Calculator
- CX Strategy & Management Hub
- Market Research Hub
- Patient Experience Hub
- Employee Experience Hub
- NPS Knowledge Hub
- Market Research Guide
- Customer Experience Guide
- Survey Research Guides
- Survey Template Library
- Webinars and Events
- Feature Sheets
- Try a sample survey
- Professional Services

Get exclusive insights into research trends and best practices from top experts! Access Voxco’s ‘State of Research Report 2024 edition’ .
We’ve been avid users of the Voxco platform now for over 20 years. It gives us the flexibility to routinely enhance our survey toolkit and provides our clients with a more robust dataset and story to tell their clients.
VP Innovation & Strategic Partnerships, The Logit Group
- Client Stories
- Voxco Reviews
- Why Voxco Research?
- Careers at Voxco
- Vulnerabilities and Ethical Hacking
Explore Regional Offices
- Survey Software The world’s leading omnichannel survey software
- Online Survey Tools Create sophisticated surveys with ease.
- Mobile Offline Conduct efficient field surveys.
- Text Analysis
- Close The Loop
- Automated Translations
- NPS Dashboard
- CATI Manage high volume phone surveys efficiently
- Cloud/On-premise Dialer TCPA compliant Cloud on-premise dialer
- IVR Survey Software Boost productivity with automated call workflows.
- Analytics Analyze survey data with visual dashboards
- Panel Manager Nurture a loyal community of respondents.
- Survey Portal Best-in-class user friendly survey portal.
- Voxco Audience Conduct targeted sample research in hours.

Predictive Analytics
- Customer 360
- Customer Loyalty
- Fraud & Risk Management
- AI/ML Enablement Services
- Credit Underwriting

Find the best survey software for you! (Along with a checklist to compare platforms)
Get Buyer’s Guide
- 100+ question types
- SMS surveys
- Financial Services
- Banking & Financial Services
- Retail Solution
- Risk Management
- Customer Lifecycle Solutions
- Net Promoter Score
- Customer Behaviour Analytics
- Customer Segmentation
- Data Unification
Explore Voxco
Watch a Demo
Download Brochures
- CX Strategy & Management Hub
- The Voxco Guide to Customer Experience
- Professional services
- Blogs & White papers
- Case Studies
Find the best customer experience platform
Uncover customer pain points, analyze feedback and run successful CX programs with the best CX platform for your team.
Get the Guide Now

VP Innovation & Strategic Partnerships, The Logit Group
- Why Voxco Intelligence?
- Our clients
- Client stories
- Featuresheets
True Experimental Design - Types & How to Conduct
SHARE THE ARTICLE ON
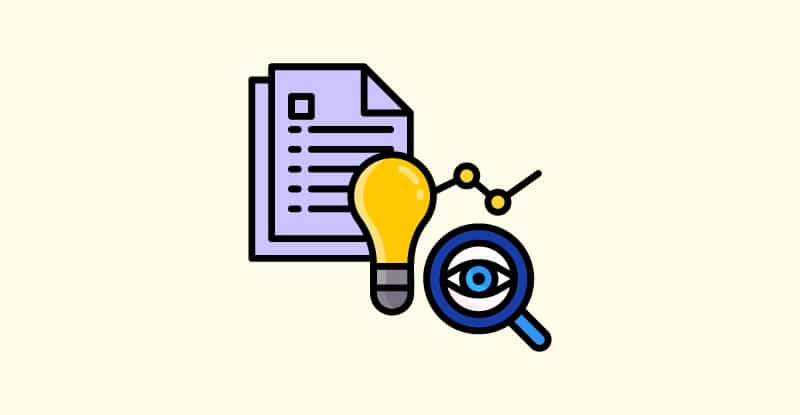
True-experimental research is often considered the most accurate research. A researcher has complete control over the process which helps reduce any error in the result. This also increases the confidence level of the research outcome.
In this blog, we will explore in detail what it is, its various types, and how to conduct it in 7 steps.
What is a true experimental design?
True experimental design is a statistical approach to establishing a cause-and-effect relationship between variables. This research method is the most accurate forms which provides substantial backing to support the existence of relationships.
There are three elements in this study that you need to fulfill in order to perform this type of research:
1. The existence of a control group: The sample of participants is subdivided into 2 groups – one that is subjected to the experiment and so, undergoes changes and the other that does not.
2. The presence of an independent variable: Independent variables that influence the working of other variables must be there for the researcher to control and observe changes.
3. Random assignment: Participants must be randomly distributed within the groups.
Read how Voxco helped Brain Research improve research productivity by 60%.
“The platform extends our ability to productively manage our busy intercept survey projects, and the confidence to support major new clients.”
Laura Ruvalcaba, President & CEO, Brain Research
An example of true experimental design
A study to observe the effects of physical exercise on productivity levels can be conducted using a true experimental design.
Suppose a group of 300 people volunteer for a study involving office workers in their 20s. These 300 participants are randomly distributed into 3 groups.
- 1st Group: A control group that does not participate in exercising and has to carry on with their everyday schedule.
- 2nd Group: Asked to indulge in home workouts for 30-45 minutes every day for one month.
- 3rd Group: Has to work out 2 hours every day for a month. Both groups have to take one rest day per week.
In this research, the level of physical exercise acts as an independent variable while the performance at the workplace is a dependent variable that varies with the change in exercise levels.
Before initiating the true experimental research, each participant’s current performance at the workplace is evaluated and documented. As the study goes on, a progress report is generated for each of the 300 participants to monitor how their physical activity has impacted their workplace functioning.
At the end of two weeks, participants from the 2nd and 3rd groups that are able to endure their current level of workout, are asked to increase their daily exercise time by half an hour. While those that aren’t able to endure, are suggested to either continue with the same timing or fix the timing to a level that is half an hour lower.
So, in this true experimental design a participant who at the end of two weeks is not able to put up with 2 hours of workout, will now workout for 1 hour and 30 minutes for the remaining tenure of two weeks while someone who can endure the 2 hours, will now push themselves towards 2 hours and 30 minutes.
In this manner, the researcher notes the timings of each member from the two active groups for the first two weeks and the remaining two weeks after the change in timings and also monitors their corresponding performance levels at work.
The above example can be categorized as true experiment research since now we have:
- Control group: Group 1 carries on with their schedule without being conditioned to exercise.
- Independent variable : The duration of exercise each day.
- Random assignment: 300 participants are randomly distributed into 3 groups and as such, there are no criteria for the assignment.

What is the purpose of conducting true experimental research?
Both the primary usage and purpose of a true experimental design lie in establishing meaningful relationships based on quantitative surveillance.
True experiments focus on connecting the dots between two or more variables by displaying how the change in one variable brings about a change in another variable. It can be as small a change as having enough sleep improves retention or as large scale as geographical differences affect consumer behavior.
The main idea is to ensure the presence of different sets of variables to study with some shared commonality.
Beyond this, the research is used when the three criteria of random distribution, a control group, and an independent variable to be manipulated by the researcher, are met.
Voxco’s omnichannel survey software helps you collect insights from multiple channels using a single platform
See the true power of using an integrated survey platform to conduct online, offline, and phone surveys along with a built-in analytical suite.
What are the advantages of true experimental design?
Let’s take a look at some advantages that make this research design conclusive and accurate research.
Concrete method of research:
The statistical nature of the experimental design makes it highly credible and accurate. The data collected from the research is subjected to statistical tools.
This makes the results easy to understand, objective and actionable. This makes it a better alternative to observation-based studies that are subjective and difficult to make inferences from.
Easy to understand and replicate:
Since the research provides hard figures and a precise representation of the entire process, the results presented become easily comprehensible for any stakeholder.
Further, it becomes easier for future researchers conducting studies around the same subject to get a grasp of prior takes on the same and replicate its results to supplement their own research.
Establishes comparison:
The presence of a control group in true experimental research allows researchers to compare and contrast. The degree to which a methodology is applied to a group can be studied with respect to the end result as a frame of reference.
Conclusive:
The research combines observational and statistical analysis to generate informed conclusions. This directs the flow of follow-up actions in a definite direction, thus, making the research process fruitful.
What are the disadvantages of true experimental design?
We should also learn about the disadvantages it can pose in research to help you determine when and how you should use this type of research.
This research design is costly. It takes a lot of investment in recruiting and managing a large number of participants which is necessary for the sample to be representative.
The high resource investment makes it highly important for the researcher to plan each aspect of the process to its minute details.
Too idealistic:
The research takes place in a completely controlled environment. Such a scenario is not representative of real-world situations and so the results may not be authentic.
T his is one of the main limitation why open-field research is preferred over lab research, wherein the researcher can influence the study.
Time-consuming:
Setting up and conducting a true experiment is highly time-consuming. This is because of the processes like recruiting a large enough sample, gathering respondent data, random distribution into groups, monitoring the process over a span of time, tracking changes, and making adjustments.
The amount of processes, although essential to the entire model, is not a feasible option to go for when the results are required in the near future.
Now that we’ve learned about the advantages and disadvantages let’s look at its types.
Get started with your Experimental Research
Send your survey to the right people to receive quality responses.
What are the 3 types of true experimental design?
The research design is categorized into three types based on the way you should conduct the research. Each type has its own procedure and guidelines, which you should be aware of to achieve reliable data.
The three types are:
1) Post-test-only control group design.
2) Pre-test post-test control group design.
3) Solomon four group control design.
Let’s see how these three types differ.
1) Post-test-only control group design:
In this type of true experimental research, the control as well as the experimental group that has been formed using random allocation, are not tested before applying the experimental methodology. This is so as to avoid affecting the quality of the study.
The participants are always on the lookout to identify the purpose and criteria for assessment. Pre-test conveys to them the basis on which they are being judged which can allow them to modify their end responses, compromising the quality of the entire research process.
However, this can hinder your ability to establish a comparison between the pre-experiment and post-experiment conditions which weighs in on the changes that have taken place over the course of the research.
2) Pre-test post-test control group design:
It is a modification of the post-test control group design with an additional test carried out before the implementation of the experimental methodology.
This two-way testing method can help in noticing significant changes brought in the research groups as a result of the experimental intervention. There is no guarantee that the results present the true picture as post-testing can be affected due to the exposure of the respondents to the pre-test.
3) Solomon four group control design:
This type of true experimental design involves the random distribution of sample members into 4 groups. These groups consist of 2 control groups that are not subjected to the experiments and changes and 2 experimental groups that the experimental methodology applies to.
Out of these 4 groups, one control and one experimental group is used for pre-testing while all four groups are subjected to post-tests.
This way researcher gets to establish pre-test post-test contrast while there remains another set of respondents that have not been exposed to pre-tests and so, provide genuine post-test responses, thus, accounting for testing effects.
Explore all the survey question types possible on Voxco.
What is the difference between pre-experimental & true experimental research design.
Pre-experimental research helps determine the researchers’ intervention on a group of people. It is a step where you design the proper experiment to address a research question.
True experiment defines that you are conducting the research. It helps establish a cause-and-effect relationship between the variables.
We’ll discuss the differences between the two based on four categories, which are:
- Observatory Vs. Statistical.
- Absence Vs. Presence of control groups.
- Non-randomization Vs. Randomization.
- Feasibility test Vs. Conclusive test.
Let’s find the differences to better understand the two experiments.
Observatory vs Statistical:
Pre-experimental research is an observation-based model i.e. it is highly subjective and qualitative in nature.
The true experimental design offers an accurate analysis of the data collected using statistical data analysis tools.
Absence vs Presence of control groups:
Pre-experimental research designs do not usually employ a control group which makes it difficult to establish contrast.
While all three types of true experiments employ control groups.
Non-randomization vs Randomization:
Pre-experimental research doesn’t use randomization in certain cases whereas
True experimental research always adheres to a randomization approach to group distribution.
Feasibility test vs Conclusive test:
Pre-tests are used as a feasibility mechanism to see if the methodology being applied is actually suitable for the research purpose and whether it will have an impact or not.
While true experiments are conclusive in nature.
Guide to Descriptive Research
Learn the key steps of conducting descriptive research to uncover breakthrough insights into your target market.
7 Steps to conduct a true experimental research
It’s important to understand the steps/guidelines of research in order to maintain research integrity and gather valid and reliable data.
We have explained 7 steps to conducting this research in detail. The TL;DR version of it is:
1) Identify the research objective.
2) Identify independent and dependent variables.
3) Define and group the population.
4) Conduct Pre-tests.
5) Conduct the research.
6) Conduct post-tests.
7) Analyse the collected data.
Now let’s explore these seven steps in true experimental design.
1) Identify the research objective:
Identify the variables which you need to analyze for a cause-and-effect relationship. Deliberate which particular relationship study will help you make effective decisions and frame this research objective in one of the following manners:
- Determination of the impact of X on Y
- Studying how the usage/application of X causes Y
2) Identify independent and dependent variables:
Establish clarity as to what would be your controlling/independent variable and what variable would change and would be observed by the researcher. In the above samples, for research purposes, X is an independent variable & Y is a dependent variable.
3) Define and group the population:
Define the targeted audience for the true experimental design. It is out of this target audience that a sample needs to be selected for accurate research to be carried out. It is imperative that the target population gets defined in as much detail as possible.
To narrow the field of view, a random selection of individuals from the population is carried out. These are the selected respondents that help the researcher in answering their research questions. Post their selection, this sample of individuals gets randomly subdivided into control and experimental groups.
4) Conduct Pre-tests:
Before commencing with the actual study, pre-tests are to be carried out wherever necessary. These pre-tests take an assessment of the condition of the respondent so that an effective comparison between the pre and post-tests reveals the change brought about by the research.
5) Conduct the research:
Implement your experimental procedure with the experimental group created in the previous step in the true experimental design. Provide the necessary instructions and solve any doubts or queries that the participants might have. Monitor their practices and track their progress. Ensure that the intervention is being properly complied with, otherwise, the results can be tainted.
6) Conduct post-tests:
Gauge the impact that the intervention has had on the experimental group and compare it with the pre-tests. This is particularly important since the pre-test serves as a starting point from where all the changes that have been measured in the post-test, are the effect of the experimental intervention.
So for example: If the pre-test in the above example shows that a particular customer service employee was able to solve 10 customer problems in two hours and the post-test conducted after a month of 2-hour workouts every day shows a boost of 5 additional customer problems being solved within those 2 hours, the additional 5 customer service calls that the employee makes is the result of the additional productivity gained by the employee as a result of putting in the requisite time
7) Analyse the collected data:
Use appropriate statistical tools to derive inferences from the data observed and collected. Correlational data analysis tools and tests of significance are highly effective relationship-based studies and so are highly applicable for true experimental research.
This step also includes differentiating between the pre and the post-tests for scoping in on the impact that the independent variable has had on the dependent variable. A contrast between the control group and the experimental groups sheds light on the change brought about within the span of the experiment and how much change is brought intentionally and is not caused by chance.
Voxco is trusted by 500+ global brands and top 50 MR firms to gather insights and take actions.
See how Voxco can help enhance your research efficiency.
Wrapping up;
This sums up everything about true experimental design. While it’s often considered complex and expensive, it is also one of the most accurate research.
The true experiment uses statistical analysis which ensures that your data is reliable and has a high confidence level. Curious to learn how you can use survey software to conduct your experimental research, book a meeting with us .

- What is true experimental research design?
True experimental research design helps investigate the cause-and-effect relationships between the variables under study. The research method requires manipulating an independent variable, random assignment of participants to different groups, and measuring the dependent variable.
- How does true experiment research differ from other research designs?
The true experiment uses random selection/assignment of participants in the group to minimize preexisting differences between groups. It allows researchers to make causal inferences about the influence of independent variables. This is the factor that makes it different from other research designs like correlational research.
- What are the key components of true experimental research designs?
The following are the important factors of a true experimental design:
- Manipulation of the independent variable.
- Control groups.
- Experiment groups.
- Dependent variable.
- Random assignment.
- What are some advantages of true experiment design?
It enables you to establish causal relationships between variables and offers control over the confounding variables. Moreover, you can generalize the research findings to the target population.
- What ethical considerations are important in a true experimental research design?
When conducting this research method, you must obtain informed consent from the participants. It’s important to ensure the confidentiality and privacy of the participants to minimize any risk or harm.
Explore Voxco Survey Software
+ Omnichannel Survey Software
+ Online Survey Software
+ CATI Survey Software
+ IVR Survey Software
+ Market Research Tool
+ Customer Experience Tool
+ Product Experience Software
+ Enterprise Survey Software

Homewood Research Institute Case Study
Case Study How HRI uses Voxco to conduct complex research studies & speed up insight generation Homewood Research Institute is an independent charitable organization dedicated
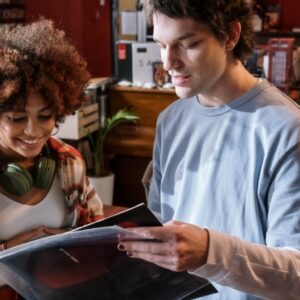
360 customer journey
360 customer journey SHARE THE ARTICLE ON Share on facebook Share on twitter Share on linkedin Table of Contents What is the 360 customer journey?
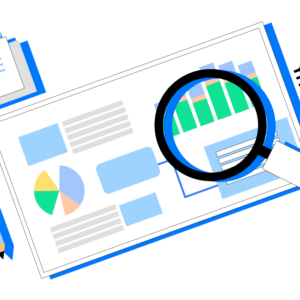
Online Survey Analysis Tool: Tips and Best Practices
Online Survey Analysis Tool: Tips and Best Practices SHARE THE ARTICLE ON Table of Contents Data are an integral part of making informed decisions in
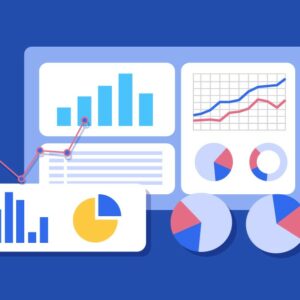
NPS Scale : Transforming Customer Feedback into Actionable Insights
NPS Scale : Transforming Customer Feedback into Actionable Insights SHARE THE ARTICLE ON Table of Contents It is important for business to know what brings

Misconceptions about Market Research Automation
Misconceptions about Market Research Automation Free Market Research Tool kit Access 5 market research survey templates + 2 MR guides Download The Toolkit Now SHARE THE
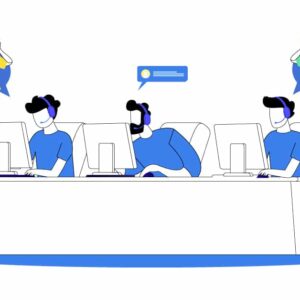
Predictive Analytics See what question types are possible with a sample survey! Try a Sample Survey Table of Contents 01 What is Predictive Analytics? Predictive
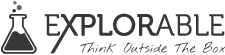
- Foundations
- Write Paper
Search form
- Experiments
- Anthropology
- Self-Esteem
- Social Anxiety

True Experimental Design
True experimental design is regarded as the most accurate form of experimental research, in that it tries to prove or disprove a hypothesis mathematically, with statistical analysis.
This article is a part of the guide:
- Research Designs
- Quantitative and Qualitative Research
- Literature Review
- Quantitative Research Design
Browse Full Outline
- 1 Research Designs
- 2.1 Pilot Study
- 2.2 Quantitative Research Design
- 2.3 Qualitative Research Design
- 2.4 Quantitative and Qualitative Research
- 3.1 Case Study
- 3.2 Naturalistic Observation
- 3.3 Survey Research Design
- 3.4 Observational Study
- 4.1 Case-Control Study
- 4.2 Cohort Study
- 4.3 Longitudinal Study
- 4.4 Cross Sectional Study
- 4.5 Correlational Study
- 5.1 Field Experiments
- 5.2 Quasi-Experimental Design
- 5.3 Identical Twins Study
- 6.1 Experimental Design
- 6.2 True Experimental Design
- 6.3 Double Blind Experiment
- 6.4 Factorial Design
- 7.1 Literature Review
- 7.2 Systematic Reviews
- 7.3 Meta Analysis
For some of the physical sciences, such as physics, chemistry and geology, they are standard and commonly used. For social sciences, psychology and biology, they can be a little more difficult to set up.
For an experiment to be classed as a true experimental design, it must fit all of the following criteria.
- The sample groups must be assigned randomly .
- There must be a viable control group .
- Only one variable can be manipulated and tested. It is possible to test more than one, but such experiments and their statistical analysis tend to be cumbersome and difficult.
- The tested subjects must be randomly assigned to either control or experimental groups.
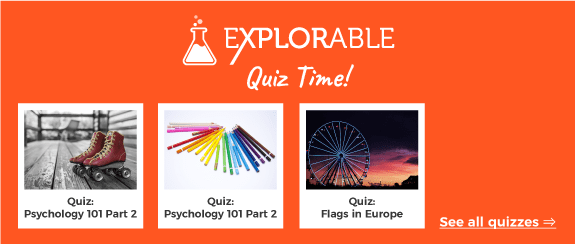
The results of a true experimental design can be statistically analyzed and so there can be little argument about the results .
It is also much easier for other researchers to replicate the experiment and validate the results.
For physical sciences working with mainly numerical data, it is much easier to manipulate one variable, so true experimental design usually gives a yes or no answer.
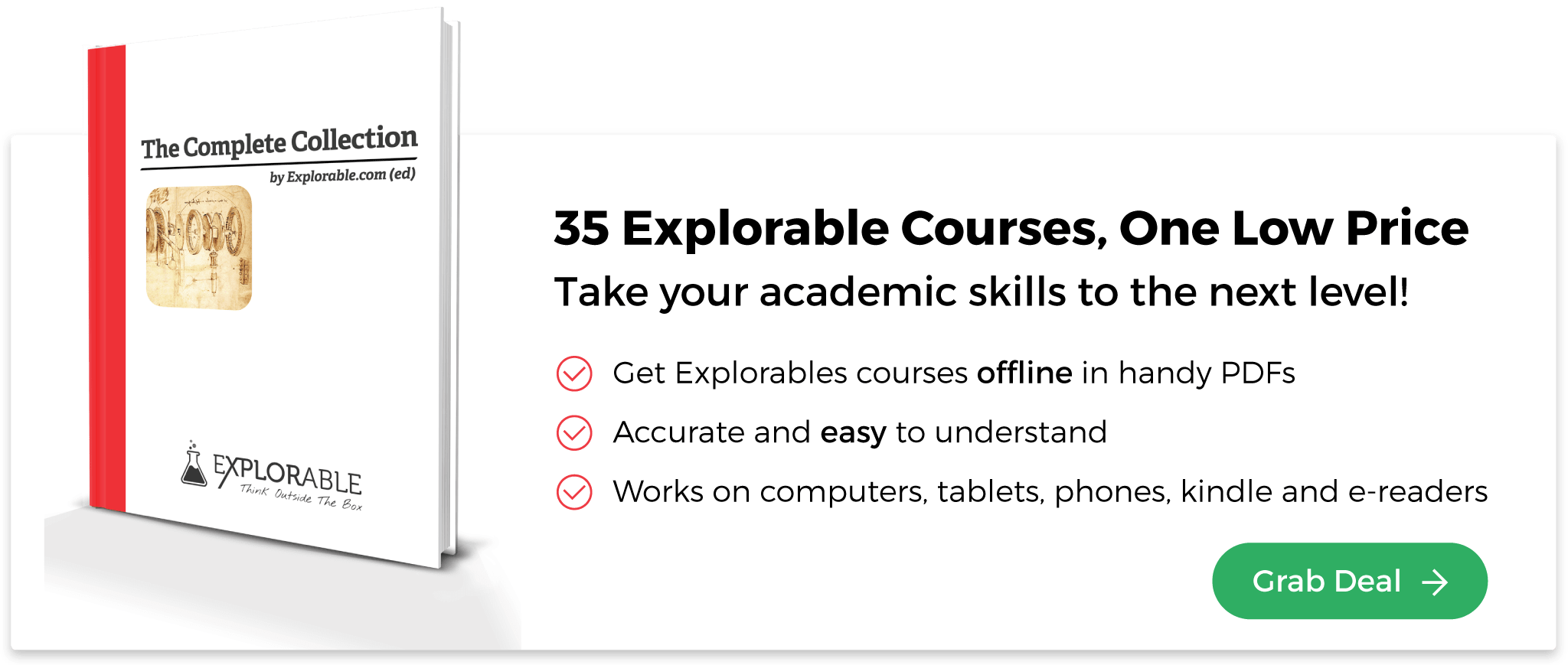
Disadvantages
Whilst perfect in principle, there are a number of problems with this type of design. Firstly, they can be almost too perfect, with the conditions being under complete control and not being representative of real world conditions.
For psychologists and behavioral biologists, for example, there can never be any guarantee that a human or living organism will exhibit ‘normal’ behavior under experimental conditions.
True experiments can be too accurate and it is very difficult to obtain a complete rejection or acceptance of a hypothesis because the standards of proof required are so difficult to reach.
True experiments are also difficult and expensive to set up. They can also be very impractical.
While for some fields, like physics, there are not as many variables so the design is easy, for social sciences and biological sciences, where variations are not so clearly defined it is much more difficult to exclude other factors that may be affecting the manipulated variable.
True experimental design is an integral part of science, usually acting as a final test of a hypothesis . Whilst they can be cumbersome and expensive to set up, literature reviews , qualitative research and descriptive research can serve as a good precursor to generate a testable hypothesis, saving time and money.
Whilst they can be a little artificial and restrictive, they are the only type of research that is accepted by all disciplines as statistically provable.
- Psychology 101
- Flags and Countries
- Capitals and Countries
Martyn Shuttleworth (Mar 24, 2008). True Experimental Design. Retrieved Jan 02, 2025 from Explorable.com: https://explorable.com/true-experimental-design
You Are Allowed To Copy The Text
The text in this article is licensed under the Creative Commons-License Attribution 4.0 International (CC BY 4.0) .
This means you're free to copy, share and adapt any parts (or all) of the text in the article, as long as you give appropriate credit and provide a link/reference to this page.
That is it. You don't need our permission to copy the article; just include a link/reference back to this page. You can use it freely (with some kind of link), and we're also okay with people reprinting in publications like books, blogs, newsletters, course-material, papers, wikipedia and presentations (with clear attribution).
Related articles
Quasi-Experimental Design
Design of Experiments
Experimental Research
Control Group
Hypothesis Testing
Want to stay up to date? Follow us!
Get all these articles in 1 guide.
Want the full version to study at home, take to school or just scribble on?
Whether you are an academic novice, or you simply want to brush up your skills, this book will take your academic writing skills to the next level.
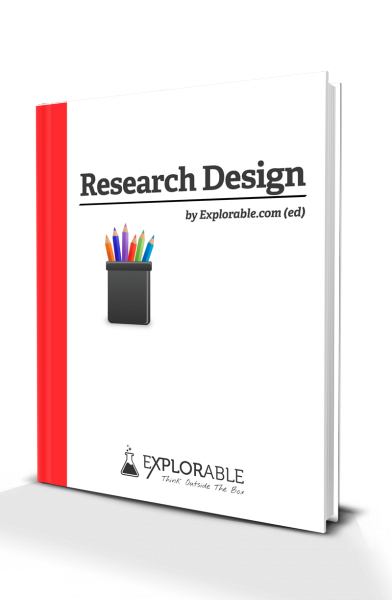
Download electronic versions: - Epub for mobiles and tablets - PDF version here
Save this course for later
Don't have time for it all now? No problem, save it as a course and come back to it later.

Footer bottom
- Privacy Policy
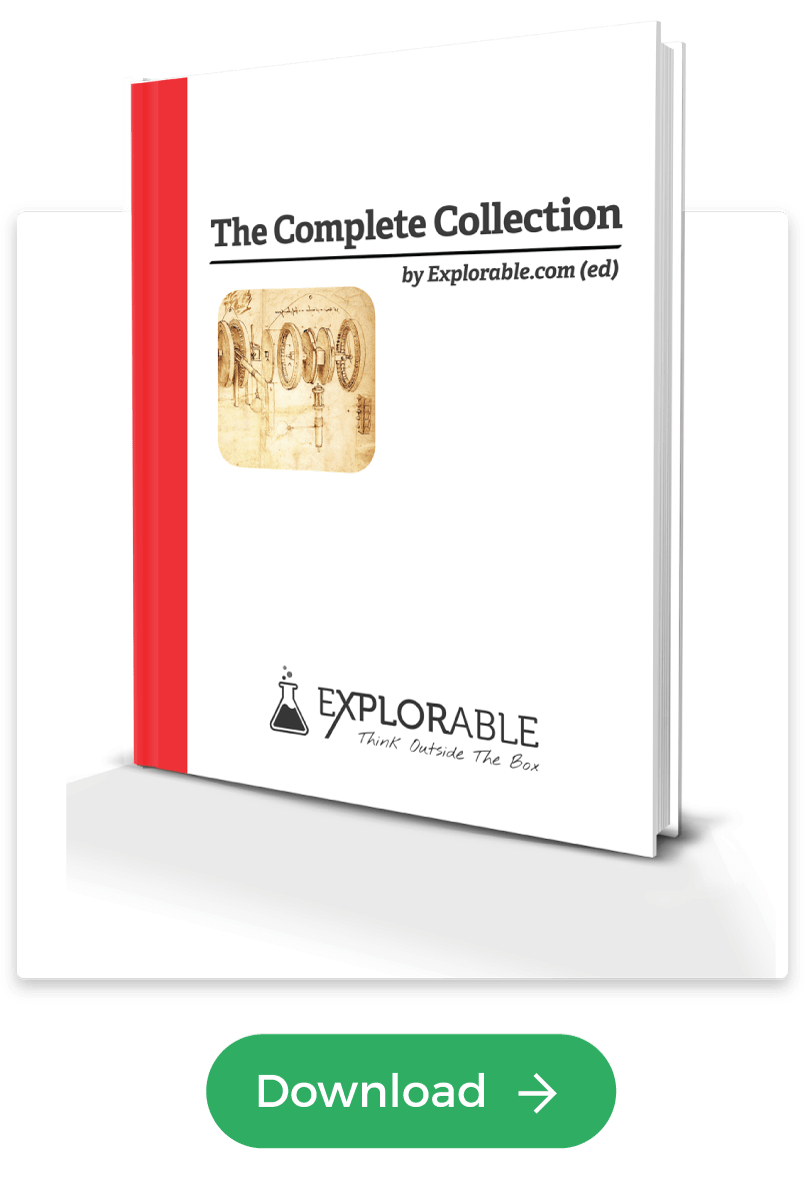
- Subscribe to our RSS Feed
- Like us on Facebook
- Follow us on Twitter
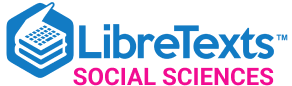
- school Campus Bookshelves
- menu_book Bookshelves
- perm_media Learning Objects
- login Login
- how_to_reg Request Instructor Account
- hub Instructor Commons
Margin Size
- Download Page (PDF)
- Download Full Book (PDF)
- Periodic Table
- Physics Constants
- Scientific Calculator
- Reference & Cite
- Tools expand_more
- Readability
selected template will load here
This action is not available.
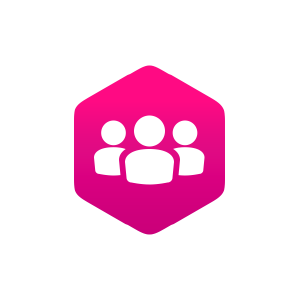
13.2: True experimental design
- Last updated
- Save as PDF
- Page ID 135156
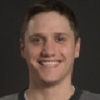
- Matthew DeCarlo, Cory Cummings, & Kate Agnelli
- Open Social Work Education
\( \newcommand{\vecs}[1]{\overset { \scriptstyle \rightharpoonup} {\mathbf{#1}} } \)
\( \newcommand{\vecd}[1]{\overset{-\!-\!\rightharpoonup}{\vphantom{a}\smash {#1}}} \)
\( \newcommand{\id}{\mathrm{id}}\) \( \newcommand{\Span}{\mathrm{span}}\)
( \newcommand{\kernel}{\mathrm{null}\,}\) \( \newcommand{\range}{\mathrm{range}\,}\)
\( \newcommand{\RealPart}{\mathrm{Re}}\) \( \newcommand{\ImaginaryPart}{\mathrm{Im}}\)
\( \newcommand{\Argument}{\mathrm{Arg}}\) \( \newcommand{\norm}[1]{\| #1 \|}\)
\( \newcommand{\inner}[2]{\langle #1, #2 \rangle}\)
\( \newcommand{\Span}{\mathrm{span}}\)
\( \newcommand{\id}{\mathrm{id}}\)
\( \newcommand{\kernel}{\mathrm{null}\,}\)
\( \newcommand{\range}{\mathrm{range}\,}\)
\( \newcommand{\RealPart}{\mathrm{Re}}\)
\( \newcommand{\ImaginaryPart}{\mathrm{Im}}\)
\( \newcommand{\Argument}{\mathrm{Arg}}\)
\( \newcommand{\norm}[1]{\| #1 \|}\)
\( \newcommand{\Span}{\mathrm{span}}\) \( \newcommand{\AA}{\unicode[.8,0]{x212B}}\)
\( \newcommand{\vectorA}[1]{\vec{#1}} % arrow\)
\( \newcommand{\vectorAt}[1]{\vec{\text{#1}}} % arrow\)
\( \newcommand{\vectorB}[1]{\overset { \scriptstyle \rightharpoonup} {\mathbf{#1}} } \)
\( \newcommand{\vectorC}[1]{\textbf{#1}} \)
\( \newcommand{\vectorD}[1]{\overrightarrow{#1}} \)
\( \newcommand{\vectorDt}[1]{\overrightarrow{\text{#1}}} \)
\( \newcommand{\vectE}[1]{\overset{-\!-\!\rightharpoonup}{\vphantom{a}\smash{\mathbf {#1}}}} \)
Learning Objectives
Learners will be able to…
- Describe a true experimental design in social work research
- Understand the different types of true experimental designs
- Determine what kinds of research questions true experimental designs are suited for
- Discuss advantages and disadvantages of true experimental designs
True experimental design , often considered to be the “gold standard” in research designs, is thought of as one of the most rigorous of all research designs. In this design, one or more independent variables are manipulated by the researcher (as treatments), subjects are randomly assigned to different treatment levels (random assignment), and the results of the treatments on outcomes (dependent variables) are observed. The unique strength of experimental research is its internal validity and its ability to establish ( causality ) through treatment manipulation, while controlling for the effects of extraneous variable. Sometimes the treatment level is no treatment, while other times it is simply a different treatment than that which we are trying to evaluate. For example, we might have a control group that is made up of people who will not receive any treatment for a particular condition. Or, a control group could consist of people who consent to treatment with DBT when we are testing the effectiveness of CBT.
As we discussed in the previous section, a true experiment has a control group with participants randomly assigned , and an experimental group . This is the most basic element of a true experiment. The next decision a researcher must make is when they need to gather data during their experiment. Do they take a baseline measurement and then a measurement after treatment, or just a measurement after treatment, or do they handle measurement another way? Below, we’ll discuss the three main types of true experimental designs. There are sub-types of each of these designs, but here, we just want to get you started with some of the basics.
Using a true experiment in social work research is often pretty difficult, since as I mentioned earlier, true experiments can be quite resource intensive. True experiments work best with relatively large sample sizes, and random assignment, a key criterion for a true experimental design, is hard (and unethical) to execute in practice when you have people in dire need of an intervention. Nonetheless, some of the strongest evidence bases are built on true experiments.
For the purposes of this section, let’s bring back the example of CBT for the treatment of social anxiety. We have a group of 500 individuals who have agreed to participate in our study, and we have randomly assigned them to the control and experimental groups. The folks in the experimental group will receive CBT, while the folks in the control group will receive more unstructured, basic talk therapy. These designs, as we talked about above, are best suited for explanatory research questions.
Before we get started, take a look at the table below. When explaining experimental research designs, we often use diagrams with abbreviations to visually represent the experiment. Table 13.1 starts us off by laying out what each of the abbreviations mean.
Table 13.1 Experimental research design notations
Pretest and post-test control group design
In pretest and post-test control group design , participants are given a pretest of some kind to measure their baseline state before their participation in an intervention. In our social anxiety experiment, we would have participants in both the experimental and control groups complete some measure of social anxiety—most likely an established scale and/or a structured interview—before they start their treatment. As part of the experiment, we would have a defined time period during which the treatment would take place (let’s say 12 weeks, just for illustration). At the end of 12 weeks, we would give both groups the same measure as a post-test .
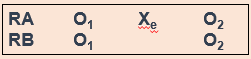
Figure 13.1 Pretest and post-test control group design
In the diagram, RA (random assignment group A) is the experimental group and RB is the control group. O 1 denotes the pre-test, X e denotes the experimental intervention, and O 2 denotes the post-test. Let’s look at this diagram another way, using the example of CBT for social anxiety that we’ve been talking about.

Figure 13.2 Pretest and post-test control group design testing CBT an intervention
In a situation where the control group received treatment as usual instead of no intervention, the diagram would look this way, with X i denoting treatment as usual (Figure 13.3).
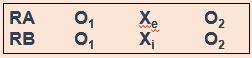
Figure 13.3 Pretest and post-test control group design with treatment as usual instead of no treatment
Hopefully, these diagrams provide you a visualization of how this type of experiment establishes time order , a key component of a causal relationship. Did the change occur after the intervention? Assuming there is a change in the scores between the pretest and post-test, we would be able to say that yes, the change did occur after the intervention. Causality can’t exist if the change happened before the intervention—this would mean that something else led to the change, not our intervention.
Post-test only control group design
Post-test only control group design involves only giving participants a post-test, just like it sounds (Figure 13.4).
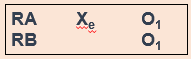
Figure 13.4 Post-test only control group design
But why would you use this design instead of using a pretest/post-test design? One reason could be the testing effect that can happen when research participants take a pretest. In research, the testing effect refers to “measurement error related to how a test is given; the conditions of the testing, including environmental conditions; and acclimation to the test itself” (Engel & Schutt, 2017, p. 444)\(^1\) (When we say “measurement error,” all we mean is the accuracy of the way we measure the dependent variable.) Figure 13.4 is a visualization of this type of experiment. The testing effect isn’t always bad in practice—our initial assessments might help clients identify or put into words feelings or experiences they are having when they haven’t been able to do that before. In research, however, we might want to control its effects to isolate a cleaner causal relationship between intervention and outcome.
Going back to our CBT for social anxiety example, we might be concerned that participants would learn about social anxiety symptoms by virtue of taking a pretest. They might then identify that they have those symptoms on the post-test, even though they are not new symptoms for them. That could make our intervention look less effective than it actually is.
However, without a baseline measurement establishing causality can be more difficult. If we don’t know someone’s state of mind before our intervention, how do we know our intervention did anything at all? Establishing time order is thus a little more difficult. You must balance this consideration with the benefits of this type of design.
Solomon four group design
One way we can possibly measure how much the testing effect might change the results of the experiment is with the Solomon four group design. Basically, as part of this experiment, you have two control groups and two experimental groups. The first pair of groups receives both a pretest and a post-test. The other pair of groups receives only a post-test (Figure 13.5). This design helps address the problem of establishing time order in post-test only control group designs.
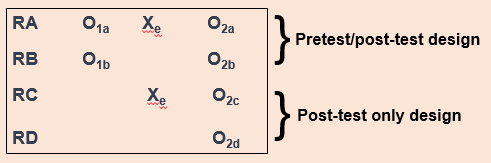
Figure 13.5 Solomon four-group design
For our CBT project, we would randomly assign people to four different groups instead of just two. Groups A and B would take our pretest measures and our post-test measures, and groups C and D would take only our post-test measures. We could then compare the results among these groups and see if they’re significantly different between the folks in A and B, and C and D. If they are, we may have identified some kind of testing effect, which enables us to put our results into full context. We don’t want to draw a strong causal conclusion about our intervention when we have major concerns about testing effects without trying to determine the extent of those effects.
Solomon four group designs are less common in social work research, primarily because of the logistics and resource needs involved. Nonetheless, this is an important experimental design to consider when we want to address major concerns about testing effects.
Key Takeaways
- True experimental design is best suited for explanatory research questions.
- True experiments require random assignment of participants to control and experimental groups.
- Pretest/post-test research design involves two points of measurement—one pre-intervention and one post-intervention.
- Post-test only research design involves only one point of measurement—post-intervention. It is a useful design to minimize the effect of testing effects on our results.
- Solomon four group research design involves both of the above types of designs, using 2 pairs of control and experimental groups. One group receives both a pretest and a post-test, while the other receives only a post-test. This can help uncover the influence of testing effects.
- Think about a true experiment you might conduct for your research project. Which design would be best for your research, and why?
- What challenges or limitations might make it unrealistic (or at least very complicated!) for you to carry your true experimental design in the real-world as a student researcher?
- What hypothesis(es) would you test using this true experiment?
- Call for Articles
- Login

Experimental Research Design — 6 mistakes you should never make!
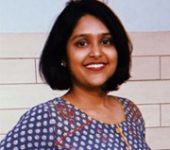
Since school days’ students perform scientific experiments that provide results that define and prove the laws and theorems in science. These experiments are laid on a strong foundation of experimental research designs.
An experimental research design helps researchers execute their research objectives with more clarity and transparency.
In this article, we will not only discuss the key aspects of experimental research designs but also the issues to avoid and problems to resolve while designing your research study.
Table of Contents
What Is Experimental Research Design?
Experimental research design is a framework of protocols and procedures created to conduct experimental research with a scientific approach using two sets of variables. Herein, the first set of variables acts as a constant, used to measure the differences of the second set. The best example of experimental research methods is quantitative research .
Experimental research helps a researcher gather the necessary data for making better research decisions and determining the facts of a research study.
When Can a Researcher Conduct Experimental Research?
A researcher can conduct experimental research in the following situations —
- When time is an important factor in establishing a relationship between the cause and effect.
- When there is an invariable or never-changing behavior between the cause and effect.
- Finally, when the researcher wishes to understand the importance of the cause and effect.
Importance of Experimental Research Design
To publish significant results, choosing a quality research design forms the foundation to build the research study. Moreover, effective research design helps establish quality decision-making procedures, structures the research to lead to easier data analysis, and addresses the main research question. Therefore, it is essential to cater undivided attention and time to create an experimental research design before beginning the practical experiment.
By creating a research design, a researcher is also giving oneself time to organize the research, set up relevant boundaries for the study, and increase the reliability of the results. Through all these efforts, one could also avoid inconclusive results. If any part of the research design is flawed, it will reflect on the quality of the results derived.
Types of Experimental Research Designs
Based on the methods used to collect data in experimental studies, the experimental research designs are of three primary types:
1. Pre-experimental Research Design
A research study could conduct pre-experimental research design when a group or many groups are under observation after implementing factors of cause and effect of the research. The pre-experimental design will help researchers understand whether further investigation is necessary for the groups under observation.
Pre-experimental research is of three types —
- One-shot Case Study Research Design
- One-group Pretest-posttest Research Design
- Static-group Comparison
2. True Experimental Research Design
A true experimental research design relies on statistical analysis to prove or disprove a researcher’s hypothesis. It is one of the most accurate forms of research because it provides specific scientific evidence. Furthermore, out of all the types of experimental designs, only a true experimental design can establish a cause-effect relationship within a group. However, in a true experiment, a researcher must satisfy these three factors —
- There is a control group that is not subjected to changes and an experimental group that will experience the changed variables
- A variable that can be manipulated by the researcher
- Random distribution of the variables
This type of experimental research is commonly observed in the physical sciences.
3. Quasi-experimental Research Design
The word “Quasi” means similarity. A quasi-experimental design is similar to a true experimental design. However, the difference between the two is the assignment of the control group. In this research design, an independent variable is manipulated, but the participants of a group are not randomly assigned. This type of research design is used in field settings where random assignment is either irrelevant or not required.
The classification of the research subjects, conditions, or groups determines the type of research design to be used.
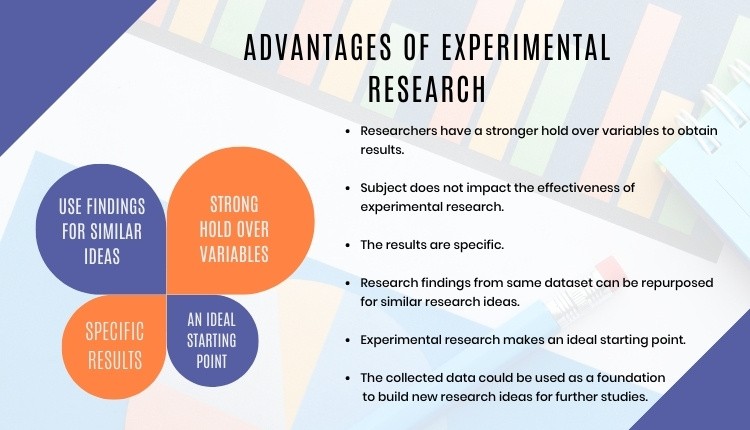
Advantages of Experimental Research
Experimental research allows you to test your idea in a controlled environment before taking the research to clinical trials. Moreover, it provides the best method to test your theory because of the following advantages:
- Researchers have firm control over variables to obtain results.
- The subject does not impact the effectiveness of experimental research. Anyone can implement it for research purposes.
- The results are specific.
- Post results analysis, research findings from the same dataset can be repurposed for similar research ideas.
- Researchers can identify the cause and effect of the hypothesis and further analyze this relationship to determine in-depth ideas.
- Experimental research makes an ideal starting point. The collected data could be used as a foundation to build new research ideas for further studies.
6 Mistakes to Avoid While Designing Your Research
There is no order to this list, and any one of these issues can seriously compromise the quality of your research. You could refer to the list as a checklist of what to avoid while designing your research.
1. Invalid Theoretical Framework
Usually, researchers miss out on checking if their hypothesis is logical to be tested. If your research design does not have basic assumptions or postulates, then it is fundamentally flawed and you need to rework on your research framework.
2. Inadequate Literature Study
Without a comprehensive research literature review , it is difficult to identify and fill the knowledge and information gaps. Furthermore, you need to clearly state how your research will contribute to the research field, either by adding value to the pertinent literature or challenging previous findings and assumptions.
3. Insufficient or Incorrect Statistical Analysis
Statistical results are one of the most trusted scientific evidence. The ultimate goal of a research experiment is to gain valid and sustainable evidence. Therefore, incorrect statistical analysis could affect the quality of any quantitative research.
4. Undefined Research Problem
This is one of the most basic aspects of research design. The research problem statement must be clear and to do that, you must set the framework for the development of research questions that address the core problems.
5. Research Limitations
Every study has some type of limitations . You should anticipate and incorporate those limitations into your conclusion, as well as the basic research design. Include a statement in your manuscript about any perceived limitations, and how you considered them while designing your experiment and drawing the conclusion.
6. Ethical Implications
The most important yet less talked about topic is the ethical issue. Your research design must include ways to minimize any risk for your participants and also address the research problem or question at hand. If you cannot manage the ethical norms along with your research study, your research objectives and validity could be questioned.
Experimental Research Design Example
In an experimental design, a researcher gathers plant samples and then randomly assigns half the samples to photosynthesize in sunlight and the other half to be kept in a dark box without sunlight, while controlling all the other variables (nutrients, water, soil, etc.)
By comparing their outcomes in biochemical tests, the researcher can confirm that the changes in the plants were due to the sunlight and not the other variables.
Experimental research is often the final form of a study conducted in the research process which is considered to provide conclusive and specific results. But it is not meant for every research. It involves a lot of resources, time, and money and is not easy to conduct, unless a foundation of research is built. Yet it is widely used in research institutes and commercial industries, for its most conclusive results in the scientific approach.
Have you worked on research designs? How was your experience creating an experimental design? What difficulties did you face? Do write to us or comment below and share your insights on experimental research designs!
Frequently Asked Questions
Randomization is important in an experimental research because it ensures unbiased results of the experiment. It also measures the cause-effect relationship on a particular group of interest.
Experimental research design lay the foundation of a research and structures the research to establish quality decision making process.
There are 3 types of experimental research designs. These are pre-experimental research design, true experimental research design, and quasi experimental research design.
The difference between an experimental and a quasi-experimental design are: 1. The assignment of the control group in quasi experimental research is non-random, unlike true experimental design, which is randomly assigned. 2. Experimental research group always has a control group; on the other hand, it may not be always present in quasi experimental research.
Experimental research establishes a cause-effect relationship by testing a theory or hypothesis using experimental groups or control variables. In contrast, descriptive research describes a study or a topic by defining the variables under it and answering the questions related to the same.

good and valuable
Very very good
Good presentation.
That was clearly understandable
Rate this article Cancel Reply
Your email address will not be published.
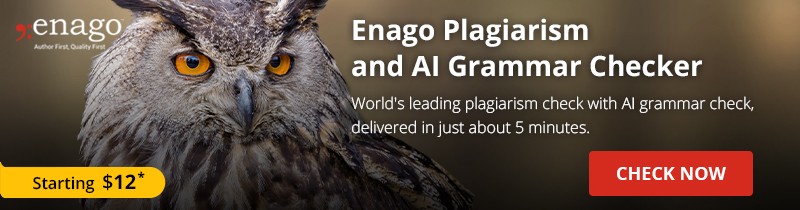
Enago Academy's Most Popular Articles
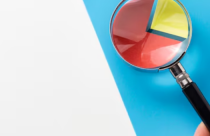
- Promoting Research
Graphical Abstracts Vs. Infographics: Best practices for using visual illustrations for increased research impact
Dr. Sarah Chen stared at her computer screen, her eyes staring at her recently published…
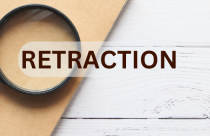
- Publishing Research
10 Tips to Prevent Research Papers From Being Retracted
Research paper retractions represent a critical event in the scientific community. When a published article…
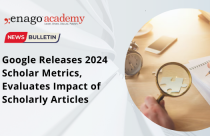
- Industry News
Google Releases 2024 Scholar Metrics, Evaluates Impact of Scholarly Articles
Google has released its 2024 Scholar Metrics, assessing scholarly articles from 2019 to 2023. This…
![true experimental in quantitative research What is Academic Integrity and How to Uphold it [FREE CHECKLIST]](https://www.enago.com/academy/wp-content/uploads/2024/05/FeatureImages-59-210x136.png)
Ensuring Academic Integrity and Transparency in Academic Research: A comprehensive checklist for researchers
Academic integrity is the foundation upon which the credibility and value of scientific findings are…
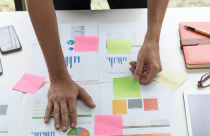
- Reporting Research
How to Optimize Your Research Process: A step-by-step guide
For researchers across disciplines, the path to uncovering novel findings and insights is often filled…
Choosing the Right Analytical Approach: Thematic analysis vs. content analysis for…
Comparing Cross Sectional and Longitudinal Studies: 5 steps for choosing the right…

Sign-up to read more
Subscribe for free to get unrestricted access to all our resources on research writing and academic publishing including:
- 2000+ blog articles
- 50+ Webinars
- 10+ Expert podcasts
- 50+ Infographics
- 10+ Checklists
- Research Guides
We hate spam too. We promise to protect your privacy and never spam you.
- Plagiarism Checker
- AI Content Detector
- Academic Editing
- Publication Support Services
- Thesis Editing
- Enago Reports
- Journal Finder
- Thought Leadership
- Diversity and Inclusion
- Al in Academia
- Career Corner
- Other Resources
- Infographics
- Enago Learn
- On-Demand Webinar
- Open Access Week
- Peer Review Week
- Publication Integrity Week
- Conference Videos
- Call for speakers
- Author Training
I am looking for Editing/ Proofreading services for my manuscript Tentative date of next journal submission:
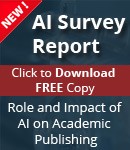
What features do you prefer in a plagiarism detector? (Select all that apply)
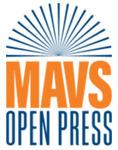
Want to create or adapt books like this? Learn more about how Pressbooks supports open publishing practices.
14.2 True experiments
Learning objectives.
Learners will be able to…
- Describe a true experimental design in social work research
- Understand the different types of true experimental designs
- Determine what kinds of research questions true experimental designs are suited for
- Discuss advantages and disadvantages of true experimental designs
A true experiment , often considered to be the “gold standard” in research designs, is thought of as one of the most rigorous of all research designs. In this design, one or more independent variables (as treatments) are manipulated by the researcher, subjects are randomly assigned (i.e., random assignment) to different treatment levels, and the results of the treatments on outcomes (dependent variables) are observed. The unique strength of experimental research is its ability to increase internal validity and help establish causality through treatment manipulation, while controlling for the effects of extraneous variables. As such they are best suited for explanatory research questions.
In true experimental design, research subjects are assigned to either an experimental group, which receives the treatment or intervention being investigated, or a control group, which does not. Control groups may receive no treatment at all, the standard treatment (which is called “treatment as usual” or TAU), or a treatment that entails some type of contact or interaction without the characteristics of the intervention being investigated. For example, the control group may participate in a support group while the experimental group is receiving a new group-based therapeutic intervention consisting of education and cognitive behavioral group therapy.
After determining the nature of the experimental and control groups, the next decision a researcher must make is when they need to collect data during their experiment. Do they take a baseline measurement and then a measurement after treatment, or just a measurement after treatment, or do they handle data collection another way? Below, we’ll discuss three main types of true experimental designs. There are sub-types of each of these designs, but here, we just want to get you started with some of the basics.
Using a true experiment in social work research is often difficult and can be quite resource intensive. True experiments work best with relatively large sample sizes, and random assignment, a key criterion for a true experimental design, is hard (and unethical) to execute in practice when you have people in dire need of an intervention. Nonetheless, some of the strongest evidence bases are built on true experiments.
For the purposes of this section, let’s bring back the example of CBT for the treatment of social anxiety. We have a group of 500 individuals who have agreed to participate in our study, and we have randomly assigned them to the control and experimental groups. The participants in the experimental group will receive CBT, while the participants in the control group will receive a series of videos about social anxiety.
Classical experiments (pretest posttest control group design)
The elements of a classical experiment are (1) random assignment of participants into an experimental and control group, (2) a pretest to assess the outcome(s) of interest for each group, (3) delivery of an intervention/treatment to the experimental group, and (4) a posttest to both groups to assess potential change in the outcome(s).
When explaining experimental research designs, we often use diagrams with abbreviations to visually represent the components of the experiment. Table 14.2 starts us off by laying out what the abbreviations mean.
Figure 14.1 depicts a classical experiment using our example of assessing the intervention of CBT for social anxiety. In the figure, RA denotes random assignment to the experimental group A and RB is random assignment to the control group B. O 1 (observation 1) denotes the pretest, X e denotes the experimental intervention, and O 2 (observation 2) denotes the posttest.

The more general, or universal, notation for classical experimental design is shown in Figure 14.2.
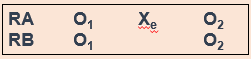
In a situation where the control group received treatment as usual instead of no intervention, the diagram would look this way (Figure 14.3), with X i denoting treatment as usual:
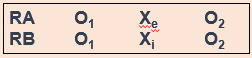
Hopefully, these diagrams provide you a visualization of how this type of experiment establishes temporality , a key component of a causal relationship. By administering the pretest, researchers can assess if the change in the outcome occured after the intervention. Assuming there is a change in the scores between the pretest and posttest, we would be able to say that yes, the change did occur after the intervention.
Posttest only control group design
Posttest only control group design involves only giving participants a posttest, just like it sounds. But why would you use this design instead of using a pretest posttest design? One reason could be to avoid potential testing effects that can happen when research participants take a pretest.
In research, the testing effect threatens internal validity when the pretest changes the way the participants respond on the posttest or subsequent assessments (Flannelly, Flannelly, & Jankowski, 2018). [1] A common example occurs when testing interventions for cognitive impairment in older adults. By taking a cognitive assessment during the pretest, participants get exposed to the items on the assessment and get to “practice” taking it (see for example, Cooley et al., 2015). [2] They may perform better the second time they take it because they have learned how to take the test, not because there have been changes in cognition. This specific type of testing effect is called the practice effect . [3]
The testing effect isn’t always bad in practice—our initial assessments might help clients identify or put into words feelings or experiences they are having when they haven’t been able to do that before. In research, however, we might want to control its effects to isolate a cleaner causal relationship between intervention and outcome. Going back to our CBT for social anxiety example, we might be concerned that participants would learn about social anxiety symptoms by virtue of taking a pretest. They might then identify that they have those symptoms on the posttest, even though they are not new symptoms for them. That could make our intervention look less effective than it actually is. To mitigate the influence of testing effects, posttest only control group designs do not administer a pretest to participants. Figure 14.4 depicts this.
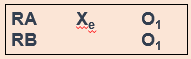
A drawback to the posttest only control group design is that without a baseline measurement, establishing causality can be more difficult. If we don’t know someone’s state of mind before our intervention, how do we know our intervention did anything at all? Establishing time order is thus a little more difficult. The posttest only control group design relies on the random assignment to groups to create groups that are equivalent at baseline because, without a pretest, researchers cannot assess whether the groups are equivalent before the intervention. Researchers must balance this consideration with the benefits of this type of design.
Solomon four group design
One way we can possibly measure how much the testing effect threatens internal validity is with the Solomon four group design. Basically, as part of this experiment, there are two experimental groups and two control groups. The first pair of experimental/control groups receives both a pretest and a posttest. The other pair receives only a posttest (Figure 14.5). In addition to addressing testing effects, this design also addresses the problems of establishing time order and equivalent groups in posttest only control group designs.
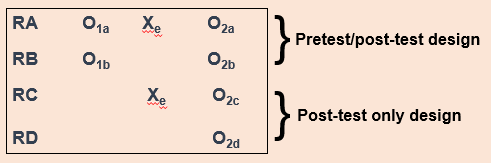
For our CBT project, we would randomly assign people to four different groups instead of just two. Groups A and B would take our pretest measures and our posttest measures, and groups C and D would take only our posttest measures. We could then compare the results among these groups and see if they’re significantly different between the folks in A and B, and C and D. If they are, we may have identified some kind of testing effect, which enables us to put our results into full context. We don’t want to draw a strong causal conclusion about our intervention when we have major concerns about testing effects without trying to determine the extent of those effects.
Solomon four group designs are less common in social work research, primarily because of the logistics and resource needs involved. Nonetheless, this is an important experimental design to consider when we want to address major concerns about testing effects.
Key Takeaways
- True experimental design is best suited for explanatory research questions.
- True experiments require random assignment of participants to control and experimental groups.
- Pretest posttest research design involves two points of measurement—one pre-intervention and one post-intervention.
- Posttest only research design involves only one point of measurement—after the intervention or treatment. It is a useful design to minimize the effect of testing effects on our results.
- Solomon four group research design involves both of the above types of designs, using 2 pairs of control and experimental groups. One group receives both a pretest and a posttest, while the other receives only a posttest. This can help uncover the influence of testing effects.
TRACK 1 (IF YOU ARE CREATING A RESEARCH PROPOSAL FOR THIS CLASS):
- Think about a true experiment you might conduct for your research project. Which design would be best for your research, and why?
- What challenges or limitations might make it unrealistic (or at least very complicated!) for you to carry your true experimental design in the real-world as a researcher?
- What hypothesis(es) would you test using this true experiment?
TRACK 2 (IF YOU AREN’T CREATING A RESEARCH PROPOSAL FOR THIS CLASS):
Imagine you are interested in studying child welfare practice. You are interested in learning more about community-based programs aimed to prevent child maltreatment and to prevent out-of-home placement for children.
- Think about a true experiment you might conduct for this research project. Which design would be best for this research, and why?
- What challenges or limitations might make it unrealistic (or at least very complicated) for you to carry your true experimental design in the real-world as a researcher?
- Flannelly, K. J., Flannelly, L. T., & Jankowski, K. R. B. (2018). Threats to the internal validity of experimental and quasi-experimental research in healthcare. Journal of Health Care Chaplaincy, 24 (3), 107-130. https://doi.org/10.1080/08854726.20 17.1421019 ↵
- Cooley, S. A., Heaps, J. M., Bolzenius, J. D., Salminen, L. E., Baker, L. M., Scott, S. E., & Paul, R. H. (2015). Longitudinal change in performance on the Montreal Cognitive Assessment in older adults. The Clinical Neuropsychologist, 29(6), 824-835. https://doi.org/10.1080/13854046.2015.1087596 ↵
- Duff, K., Beglinger, L. J., Schultz, S. K., Moser, D. J., McCaffrey, R. J., Haase, R. F., Westervelt, H. J., Langbehn, D. R., Paulsen, J. S., & Huntington's Study Group (2007). Practice effects in the prediction of long-term cognitive outcome in three patient samples: a novel prognostic index. Archives of clinical neuropsychology : the official journal of the National Academy of Neuropsychologists, 22(1), 15–24. https://doi.org/10.1016/j.acn.2006.08.013 ↵
An experimental design in which one or more independent variables are manipulated by the researcher (as treatments), subjects are randomly assigned to different treatment levels (random assignment), and the results of the treatments on outcomes (dependent variables) are observed
Ability to say that one variable "causes" something to happen to another variable. Very important to assess when thinking about studies that examine causation such as experimental or quasi-experimental designs.
the idea that one event, behavior, or belief will result in the occurrence of another, subsequent event, behavior, or belief
A demonstration that a change occurred after an intervention. An important criterion for establishing causality.
an experimental design in which participants are randomly assigned to control and treatment groups, one group receives an intervention, and both groups receive only a post-test assessment
The measurement error related to how a test is given; the conditions of the testing, including environmental conditions; and acclimation to the test itself
improvements in cognitive assessments due to exposure to the instrument
Doctoral Research Methods in Social Work Copyright © by Mavs Open Press. All Rights Reserved.
Share This Book
No internet connection.
All search filters on the page have been cleared., your search has been saved..
- Sign in to my profile My Profile
Reader's guide
Entries a-z, subject index.
- True Experimental Design
- By: Michael A. Dawes
- In: Encyclopedia of Research Design
- Chapter DOI: https:// doi. org/10.4135/9781412961288.n472
- Subject: Anthropology , Business and Management , Criminology and Criminal Justice , Communication and Media Studies , Counseling and Psychotherapy , Economics , Education , Geography , Health , History , Marketing , Nursing , Political Science and International Relations , Psychology , Social Policy and Public Policy , Social Work , Sociology , Technology , Medicine
- Show page numbers Hide page numbers
The exact definition of true experimental designs has been debated. The term true experiment is sometimes used to refer to any randomized experiment. In other instances, the term true experiment is used to describe all studies with at least one independent variable that is experimentally manipulated and with at least one dependent or outcome variable. Furthermore, the word true has been interpreted to mean there are a limited number of correct experimental methods. Regardless of the exact definition, the distinguishing feature of true experimental designs is that the units of study—be they individuals, animals, time periods, areas, clinics, or institutions (i.e., whoever or whatever is assigned to an experimental condition is the unit)—are randomly assigned to different treatment conditions.
Because of the various meanings and the use of the term true experiment , others have preferred to use the term randomized experiment as a clearer, more concise description of the experimental design. A randomized experiment is any experiment in which units are randomly assigned to receive one or more treatments or one or more alternative control conditions. Randomized assignment refers to any procedure for assigning units to either treatment or control conditions based on chance, with the potential for every unit to be assigned to one of the treatment conditions at a greater than zero probability. In the following sections, how random assignment facilitates causal inference is discussed, and common designs that use random assignment are presented.
Random Assignment and Causality
For randomized experiments, the use of random assignment is important because it facilitates causal inference. For example, randomization ensures that the experimental units' treatment condition is not confounded by an alternative cause or systematically introduced difference between conditions. Randomization, therefore, reduces potential threats to experimental validity by dispersing these threats randomly across experimental groups to minimize group differences before treatment begins. Randomization allows the researcher to know how groups were assigned and has the advantage that the selection process can be modeled accurately. Random assignment also allows for the computation of valid estimates of error variance that are orthogonal to treatment conditions. Each advantage captures different aspects of the benefits of randomization. Random assignment is the only design feature that accomplishes all the above in the same experiment, and it does so reliably while minimizing threats to internal validity.
Designs Using Random Assignment
This section presents various randomized experimental designs. The following designs are among the most commonly used in field and treatment research and are basic designs from which more complex designs can be built. With each design, key features include control and manipulation over the timing, intensity, and duration of experimental variables. The basic design of a randomized experiment requires a minimum of two conditions, with random assignment of experimental units to treatment conditions, followed by posttest assessment of units. Herein, the letter “R” indicates that the group on a given line is formed by random assignment. Although random assignment R is placed at the beginning of each line, in practice, [Page 1544] randomization often occurs before or after pretests. “X” is the treatment and “O” is the observation. The basic design can be depicted as
The diagram presented above depicts a two-group experiment in which one group receives the experimental treatment X followed by observation O, and the second group acts as a comparison to be observed without experimental manipulation. This second group is one example of a control condition. An important issue with this design is the selection of the control condition. In all randomized experiments, the researcher must consider what factors need to be controlled in the design of the study. Depending on what hypothesis needs to be tested, the number and type of treatment and control groups might vary. Examples of control groups include no-treatment controls, dose-response controls, wait-list controls, expectancy controls, or attention-only controls.
The Basic Randomized Design Comparing Two Treatments
One variation of the randomized design uses two treatments, one of which is a gold standard treatment of known efficacy. This design can be used to test what is the effect of the new treatment compared with the effect of the standard treatment. This design often works well when the standard treatment has already been shown to be effective when compared with no-treatment controls (see basic design, presented previously):
The Basic Randomized Design Comparing Two Treatments and a Control
A second variation of the randomized design is used when it is unknown whether experimental units after treatments A and B are likely to be different (e.g., because of the treatment being equally effective or equally ineffective) at posttest. In this case, a control group is added to the basic randomized design, as follows:
The Pretest–Posttest Control Group Design
Often, it is advantageous to add a pretest to the basic randomized design, as follows:
Or, random assignment can occur after the pretest, as follows:
The two designs presented earlier are frequently used for randomized field experiments because they offer two major advantages. First, they can help to minimize threats to internal validity resulting from attrition. Second, they can be used with specific statistics to increase power to reject the null hypothesis.
In some instances, however, it makes sense not to use a pretest. Examples include when pretesting is expected to produce an unwanted change in the experimental units before the treatment occurs, when pretesting cannot be obtained, and when the pretest condition is known to be constant.
The Alternative-Treatment Design with Pretest
Pretests are also recommended when comparing different treatments. If the researcher has reason to believe there might be no difference in outcomes between two alternative treatments, this design allows for the determination of whether both groups improved or whether neither did. Another advantage of this design is its utility when the researcher faces the challenge that it is unethical to withhold treatment to a group of patients, but it is unknown which treatment is more effective. Alternatively, this design can be used when there is a gold-standard treatment against which all other treatments can be compared.
Multiple Treatments and Controls with Pretest
Randomized experiments with pretests can also include a control group and multiple treatment groups. This design can be expanded to include more than two treatments and more than one control condition. For example, the Treatment of Depression Collaborative Research Program studied 250 depressed adults who were randomized to receive one of three active treatments: cognitive behavior therapy, interpersonal therapy, antidepressant therapy (imipramine) with clinical management, or placebo medication with clinical management (i.e., control condition).
This design can also be used to manipulate experimentally independent variables that are likely to have differing measurable effects on experimental variables at different doses or with increasing levels (i.e., parametric or dose-response studies). For example, in a study conducted in Cali, Columbia, adults were administered educational, nutritional, and medical treatment in four “doses” of total time receiving the following treatments: 990 hours, 2,070 hours, 3,130 hours, and 4,170 hours. The results showed significant effects at higher doses, with minimal effects at the lowest dosage.
Factorial Designs
Factorial designs use two or more independent variables (i.e., factors), which have at least two levels, and are combined such that all logical combinations of factors can be tested. The simplest version of this design is called a two-by-two factorial design, which is depicted as follows:
Factorial designs have several advantages. They can allow for smaller sample sizes than might be needed when using other designs, because each participant can receive the treatment conditions simultaneously. Second, factorial designs can be used to test whether a combination of treatments is more effective than one treatment or no treatment. Third, experiments that use a factorial design can be used to test for interactions (i.e., when the effects of treatment vary over levels of another variable).
Although factorial designs have clear advantages and are commonly used in laboratory research, they also have disadvantages because of the difficulty of implementing this design in many situations. For example, factorial designs require rigorous control over the combinations of treatments administered to each experimental unit, but maintaining control over multiple factors and multiple levels can pose special challenges.
Longitudinal Designs
Longitudinal designs use multiple observations that occur before, during, and after treatment, with the number and timing of observations determined by the specific hypotheses. Longitudinal designs have several advantages. They can determine change over time with the use of growth-curve models of individual differences in response to treatment. Longitudinal designs can also increase statistical power when five or more waves of observations are used, even when sample sizes are small.
Unfortunately, longitudinal designs have many disadvantages. Importantly, attrition might increase with longer follow-up periods and require rigorous follow-up procedures. For longitudinal randomized treatment studies, it is often unethical to withhold treatment for long periods of time using no-treatment or wait-list control groups.
Crossover Designs
Crossover designs are generally used with participants who are randomized to receive two or more treatments, after which they receive a posttest. After the posttest, the participants are assigned to the other treatment they did not receive previously and then they receive a second posttest, in the following design:
The crossover design is used to counterbalance and assess order effects. The crossover design is also used to obtain more information on causality than would be possible with only the first posttests. In either case, crossover designs are most useful when the treatments do not produce carryover effects. (Carryover effects occur when one treatment has residual effects such that the effects of the first treatment continue after beginning a second treatment.)
Solomon Four-Group Design
The Solomon four-group design is often used to control for effects of pretesting. This design uses two experimental groups and two control groups. After randomization, for each respective group, one receives a pretest and the other does not. This design allows for examination of the effects of the pretest to determine whether the pretest itself is an active treatment or intervention.
The true experimental design is a synonym for randomized experiments. Randomized experiments are useful because they facilitate causal inference and reduce threats to internal validity. A variety of experimental designs can be used, depending on what research hypotheses are to be tested. However, randomized experiments are often complex, expensive, and difficult to do. Therefore, they should be conducted only after preliminary study results support very specific testable hypotheses.
- Cause and Effect
- Internal Validity
- Quasi-Experimental Design
- Randomization Tests
- Research Design Principles
- Research Hypothesis
- Threats to Validity
Further Readings
- Triple-Blind Study
- True Positive
- Central Tendency, Measures of
- Cohen's d Statistic
- Cohen's f Statistic
- Correspondence Analysis
- Descriptive Statistics
- Effect Size, Measures of
- Eta-Squared
- Factor Loadings
- Krippendorff's Alpha
- Partial Eta-Squared
- Standard Deviation
- Trimmed Mean
- Variability, Measure of
- z Distribution
- Bernoulli Distribution
- Copula Functions
- Cumulative Frequency Distribution
- Distribution
- Frequency Distribution
- Law of Large Numbers
- Normal Distribution
- Normalizing Data
- Poisson Distribution
- Quetelet's Index
- Sampling Distributions
- Weibull Distribution
- Box-and-Whisker Plot
- Column Graph
- Frequency Table
- Graphical Display of Data
- Growth Curve
- L'Abbé Plot
- Radial Plot
- Residual Plot
- Scatterplot
- U-Shaped Curve
- Alternative Hypotheses
- Critical Value
- Decision Rule
- Nondirectional Hypotheses
- Nonsignificance
- Null Hypothesis
- One-Tailed Test
- Power Analysis
- Significance Level, Concept of
- Significance Level, Interpretation and Construction
- Significance, Statistical
- Two-Tailed Test
- Type I Error
- Type II Error
- Type III Error
- “Coefficient Alpha and the Internal Structure of Tests”
- “Convergent and Discriminant Validation by the Multitrait–Multimethod Matrix”
- “Meta-Analysis of Psychotherapy Outcome Studies”
- “On the Theory of Scales of Measurement”
- “Probable Error of a Mean, The”
- “Psychometric Experiments”
- “Sequential Tests of Statistical Hypotheses”
- “Technique for the Measurement of Attitudes, A”
- “Validity”
- Aptitudes and Instructional Methods
- Doctrine of Chances, The
- Logic of Scientific Discovery, The
- Nonparametric Statistics for the Behavioral Sciences
- Probabilistic Models for Some Intelligence and Attainment Tests
- Statistical Power Analysis for the Behavioral Sciences
- Teoria Statistica Delle Classi e Calcolo Delle Probabilità
- Q -Statistic
- Association, Measures of
- Coefficient of Concordance
- Coefficient of Variation
- Coefficients of Correlation, Alienation, and Determination
- Confidence Intervals
- Margin of Error
- Nonparametric Statistics
- Parametric Statistics
- Partial Correlation
- Pearson Product-Moment Correlation Coefficient
- Polychoric Correlation Coefficient
- Regression Coefficient
- Semipartial Correlation Coefficient
- Spearman Rank Order Correlation
- Standard Error of Estimate
- Standard Error of the Mean
- Student's t Test
- Unbiased Estimator
- b Parameter
- Computerized Adaptive Testing
- Differential Item Functioning
- Guessing Parameter
- General Linear Model
- Matrix Algebra
- Polynomials
- Sensitivity Analysis
- Yates's Notation
- Ceiling Effect
- Change Scores
- False Positive
- Gain Scores, Analysis of
- Instrumentation
- Item Analysis
- Item-Test Correlation
- Observations
- Percentile Rank
- Psychometrics
- Random Error
- Response Bias
- Sensitivity
- Social Desirability
- Specificity
- Standardized Score
- American Educational Research Association
- American Statistical Association
- National Council on Measurement in Education
- American Psychological Association Style
- Discussion Section
- Dissertation
- Literature Review
- Methods Section
- Purpose Statement
- Results Section
- Content Analysis
- Discourse Analysis
- Ethnography
- Focus Group
- Interviewing
- Narrative Research
- Naturalistic Inquiry
- Naturalistic Observation
- Qualitative Research
- Think-Aloud Methods
- Coefficient Alpha
- Correction for Attenuation
- Internal Consistency Reliability
- Interrater Reliability
- Parallel Forms Reliability
- Reliability
- Spearman–Brown Prophecy Formula
- Split-Half Reliability
- Standard Error of Measurement
- Test–Retest Reliability
- Aptitude-Treatment Interaction
- Concomitant Variable
- Confounding
- Control Group
- Interaction
- Internet-Based Research Method
- Intervention
- Natural Experiments
- Network Analysis
- Replication
- Treatment(s)
- Triangulation
- Unit of Analysis
- Yoked Control Procedure
- A Priori Monte Carlo Simulation
- Action Research
- Adaptive Designs in Clinical Trials
- Applied Research
- Behavior Analysis Design
- Block Design
- Case-Only Design
- Causal-Comparative Design
- Cohort Design
- Completely Randomized Design
- Cross-Sectional Design
- Crossover Design
- Double-Blind Procedure
- Ex Post Facto Study
- Experimental Design
- Factorial Design
- Field Study
- Group-Sequential Designs in Clinical Trials
- Laboratory Experiments
- Latin Square Design
- Longitudinal Design
- Meta-Analysis
- Mixed Methods Design
- Mixed Model Design
- Monte Carlo Simulation
- Nested Factor Design
- Nonexperimental Design
- Observational Research
- Panel Design
- Partially Randomized Preference Trial Design
- Pilot Study
- Pragmatic Study
- Pre-Experimental Designs
- Pretest–Posttest Design
- Prospective Study
- Quantitative Research
- Randomized Block Design
- Repeated Measures Design
- Response Surface Design
- Retrospective Study
- Sequential Design
- Single-Blind Study
- Single-Subject Design
- Split-Plot Factorial Design
- Thought Experiments
- Time Studies
- Time-Lag Study
- Time-Series Study
- Wennberg Design
- Within-Subjects Design
- Zelen's Randomized Consent Design
- Animal Research
- Declaration of Helsinki
- Ethics in the Research Process
- Informed Consent
- Nuremberg Code
- Participants
- Recruitment
- Clinical Significance
- Clinical Trial
- Cross-Validation
- Data Cleaning
- Delphi Technique
- Evidence-Based Decision Making
- Exploratory Data Analysis
- Inference: Deductive and Inductive
- Last Observation Carried Forward
- Planning Research
- Primary Data Source
- Q Methodology
- Research Question
- Scientific Method
- Secondary Data Source
- Standardization
- Statistical Control
- Critical Thinking
- Ecological Validity
- Experimenter Expectancy Effect
- External Validity
- File Drawer Problem
- Hawthorne Effect
- Heisenberg Effect
- John Henry Effect
- Multiple Treatment Interference
- Multivalued Treatment Effects
- Nonclassical Experimenter Effects
- Order Effects
- Placebo Effect
- Pretest Sensitization
- Random Assignment
- Reactive Arrangements
- Regression to the Mean
- Sequence Effects
- Validity of Research Conclusions
- Volunteer Bias
- White Noise
- Cluster Sampling
- Convenience Sampling
- Demographics
- Exclusion Criteria
- Experience Sampling Method
- Nonprobability Sampling
- Probability Sampling
- Proportional Sampling
- Quota Sampling
- Random Sampling
- Random Selection
- Sample Size
- Sample Size Planning
- Sampling and Retention of Underrepresented Groups
- Sampling Error
- Stratified Sampling
- Systematic Sampling
- Categorical Variable
- Guttman Scaling
- Interval Scale
- Levels of Measurement
- Likert Scaling
- Nominal Scale
- Ordinal Scale
- Ratio Scale
- Thurstone Scaling
- Software, Free
- Homogeneity of Variance
- Homoscedasticity
- Multivariate Normal Distribution
- Normality Assumption
- Autocorrelation
- Biased Estimator
- Cohen's Kappa
- Collinearity
- Correlation
- Criterion Problem
- Critical Difference
- Data Mining
- Data Snooping
- Degrees of Freedom
- Directional Hypothesis
- Disturbance Terms
- Error Rates
- Expected Value
- Fixed-Effects Model
- Inclusion Criteria
- Influence Statistics
- Influential Data Points
- Intraclass Correlation
- Latent Variable
- Likelihood Ratio Statistic
- Loglinear Models
- Main Effects
- Markov Chains
- Method Variance
- Mixed- and Random-Effects Models
- Multilevel Modeling
- Omega Squared
- Orthogonal Comparisons
- Overfitting
- Pooled Variance
- Quality Effects Model
- Random-Effects Models
- Regression Artifacts
- Regression Discontinuity
- Restriction of Range
- Root Mean Square Error
- Rosenthal Effect
- Serial Correlation
- Simple Main Effects
- Simpson's Paradox
- Sums of Squares
- Accuracy in Parameter Estimation
- Analysis of Covariance (ANCOVA)
- Analysis of Variance (ANOVA)
- Barycentric Discriminant Analysis
- Bivariate Regression
- Bonferroni Procedure
- Bootstrapping
- Canonical Correlation Analysis
- Categorical Data Analysis
- Confirmatory Factor Analysis
- Contrast Analysis
- Descriptive Discriminant Analysis
- Discriminant Analysis
- Dummy Coding
- Effect Coding
- Exploratory Factor Analysis
- Greenhouse–Geisser Correction
- Hierarchical Linear Modeling
- Holm's Sequential Bonferroni Procedure
- Latent Growth Modeling
- Least Squares, Methods of
- Logistic Regression
- Mean Comparisons
- Missing Data, Imputation of
- Multiple Regression
- Multivariate Analysis of Variance (MANOVA)
- Pairwise Comparisons
- Path Analysis
- Post Hoc Analysis
- Post Hoc Comparisons
- Principal Components Analysis
- Propensity Score Analysis
- Sequential Analysis
- Stepwise Regression
- Structural Equation Modeling
- Survival Analysis
- Trend Analysis
- Yates's Correction
- t Test, Independent Samples
- t Test, One Sample
- t Test, Paired Samples
- Bartlett's Test
- Behrens–Fisher t′ Statistic
- Chi-Square Test
- Duncan's Multiple Range Test
- Dunnett's Test
- Fisher's Least Significant Difference Test
- Friedman Test
- Honestly Significant Difference (HSD) Test
- Kolmogorov-Smirnov Test
- Kruskal–Wallis Test
- Mann–Whitney U Test
- Mauchly Test
- McNemar's Test
- Multiple Comparison Tests
- Newman–Keuls Test and Tukey Test
- Omnibus Tests
- Scheffé Test
- Tukey's Honestly Significant Difference (HSD)
- Welch's t Test
- Wilcoxon Rank Sum Test
- Bayes's Theorem
- Central Limit Theorem
- Classical Test Theory
- Correspondence Principle
- Critical Theory
- Falsifiability
- Game Theory
- Gauss–Markov Theorem
- Generalizability Theory
- Grounded Theory
- Item Response Theory
- Occam's Razor
- Probability, Laws of
- Theory of Attitude Measurement
- Weber–Fechner Law
- Control Variables
- Criterion Variable
- Dependent Variable
- Dichotomous Variable
- Endogenous Variables
- Exogenous Variables
- Independent Variable
- Nuisance Variable
- Predictor Variable
- Random Variable
- Concurrent Validity
- Construct Validity
- Content Validity
- Criterion Validity
- Face Validity
- Multitrait–Multimethod Matrix
- Predictive Validity
- Systematic Error
- Validity of Measurement
Sign in to access this content
Get a 30 day free trial, more like this, sage recommends.
We found other relevant content for you on other Sage platforms.
Have you created a personal profile? Login or create a profile so that you can save clips, playlists and searches
- Sign in/register
Navigating away from this page will delete your results
Please save your results to "My Self-Assessments" in your profile before navigating away from this page.
Sign in to my profile
Please sign into your institution before accessing your profile
Sign up for a free trial and experience all Sage Learning Resources have to offer.
You must have a valid academic email address to sign up.
Get off-campus access
- View or download all content my institution has access to.
Sign up for a free trial and experience all Sage Learning Resources has to offer.
- view my profile
- view my lists

IMAGES
COMMENTS
The true experimental design offers an accurate analysis of the data collected using statistical data analysis tools. Absence vs Presence of control groups: Pre-experimental research designs do not usually employ a control group which makes it difficult to establish contrast. While all three types of true experiments employ control groups.
True experimental design is regarded as the most accurate form of experimental research - it can prove or disapprove a hypothesis. ... 2.2 Quantitative Research Design; 2.3 Qualitative Research Design; 2.4 Quantitative and Qualitative Research; 3 Descriptive Research. 3.1 Case Study;
Determine what kinds of research questions true experimental designs are suited for; Discuss advantages and disadvantages of true experimental designs; True experimental design, often considered to be the "gold standard" in research designs, is thought of as one of the most rigorous of all research designs. In this design, one or more ...
A true experimental research design relies on statistical analysis to prove or disprove a researcher's hypothesis. It is one of the most accurate forms of research because it provides specific scientific evidence. ... Therefore, incorrect statistical analysis could affect the quality of any quantitative research. 4. Undefined Research Problem.
True experiments must have a control group, which is a group of research participants that resemble the experimental group but do not receive the experimental treatment. The control group provides ...
True experimental research involves random assignment to groups so participants each have an equal chance of receiving any of the treatments (including no treatment) under study. Quasi-experimental research does not ... Quantitative research designs are often used to look at causal relationships, but they
While there are many types of quantitative research designs, they generally fall under one of two umbrellas: experimental research and non-ex. ... The most convincing results for that would come from a true experimental design with random sampling and random assignment to groups. Ultimately, this is a decision that should be made in close ...
A true experiment, often considered to be the "gold standard" in research designs, is thought of as one of the most rigorous of all research designs.In this design, one or more independent variables (as treatments) are manipulated by the researcher, subjects are randomly assigned (i.e., random assignment) to different treatment levels, and the results of the treatments on outcomes ...
fact, "No other type of quantitative research (descriptive, correlational, or causal-comparative) is as powerful in demonstrating the existence of cause-and-effect relationships among variables as experimental research" (Gall et al., 2005, p. 249). There are three designs that meet the characteristics of true experimental
The exact definition of true experimental designs has been debated. The term true experiment is sometimes used to refer to any randomized experiment. In other instances, the term true experiment is used to describe all studies with at least one independent variable that is experimentally manipulated and with at least one dependent or outcome variable.