- Privacy Policy

Home » Dissertation Methodology – Structure, Example and Writing Guide

Dissertation Methodology – Structure, Example and Writing Guide
Table of Contents

Dissertation Methodology
The methodology section of a dissertation explains the approach, design, and methods you used to conduct your research. This section is critical for demonstrating the rigor and credibility of your study and allows readers to evaluate the reliability and validity of your findings. The methodology should be clear, detailed, and justified, ensuring that anyone reading it understands how and why the research was conducted.
Purpose of a Dissertation Methodology
The methodology section serves several essential purposes:
- Justifies the Research Approach : Explains why specific research methods were chosen and how they align with the research question.
- Describes Data Collection and Analysis : Details the tools, techniques, and procedures used to gather and analyze data.
- Ensures Replicability : Provides enough detail for other researchers to replicate or build on the study.
- Addresses Limitations : Identifies any limitations or constraints of the chosen methodology and their potential impact on results.
Structure of a Dissertation Methodology
A well-organized methodology section is usually structured into five main components: Research Design , Participants/Sampling , Data Collection Methods , Data Analysis , and Ethical Considerations . Some dissertations may include additional sections as needed for specific methods or fields of study.
1. Research Design
Definition : The research design is the overall strategy and framework guiding the study. It outlines whether the research is qualitative, quantitative, or mixed-methods and justifies this choice in relation to the research question.
Example : If studying consumer behavior, a quantitative survey might be appropriate to gather statistical data, while qualitative interviews could provide in-depth insights into motivations.
Typical Components :
- Approach : Qualitative, quantitative, or mixed-methods.
- Research Type : Experimental, observational, survey-based, case study, etc.
- Justification : Explanation of why this design aligns with the research objectives.
2. Participants and Sampling
Definition : This section describes the study population, how participants were chosen, and the sample size. It should include the criteria for inclusion and exclusion, as well as details about recruitment procedures.
Example : For a study on workplace satisfaction, you might select employees from various departments in a company and use a sample size calculated based on statistical power analysis.
- Target Population : The demographic characteristics and scope of the population studied.
- Sampling Method : Probability or non-probability sampling (e.g., random sampling, convenience sampling).
- Sample Size : The number of participants included and justification for this number.
3. Data Collection Methods
Definition : This section describes how data was collected, detailing the tools and techniques used. It should include specifics on instruments (e.g., surveys, interviews, experiments), how they were administered, and why these methods are appropriate for the study.
Example : In a study examining consumer satisfaction, data collection could involve an online survey with closed-ended questions to quantify satisfaction levels.
- Tools/Procedures : Description of instruments, procedures, and protocols.
- Type of Data Collected : Qualitative (e.g., interviews, focus groups) or quantitative (e.g., surveys, experiments).
- Rationale : Explanation of why these data collection methods were chosen.
4. Data Analysis
Definition : Data analysis involves explaining the methods and techniques used to interpret the collected data. This includes any statistical or thematic analysis methods applied, as well as software used.
Example : For quantitative data, you might use software like SPSS or R for statistical analysis, while qualitative data could be analyzed using NVivo to identify key themes.
- Techniques : Statistical tests for quantitative data (e.g., t-tests, regression analysis) or coding for qualitative data.
- Software : Mention any software used, such as SPSS, R, or NVivo.
- Justification : Explanation of why these analysis methods are appropriate for the research question and data.
5. Ethical Considerations
Definition : Ethical considerations involve addressing any ethical issues related to the study, such as informed consent, confidentiality, and participant welfare.
Example : In a study involving human subjects, ethical considerations might include obtaining informed consent and ensuring data anonymity.
- Informed Consent : Description of how participants were informed about the study and consent obtained.
- Confidentiality : Explanation of measures taken to protect participants’ privacy.
- Risk Mitigation : Any procedures in place to protect participants from harm.
Example of a Dissertation Methodology
Here is a sample methodology for a hypothetical dissertation examining the impact of online learning on student engagement.
Title : The Impact of Online Learning Platforms on Student Engagement in Higher Education
- This study employs a mixed-methods approach, combining quantitative surveys and qualitative interviews to obtain a comprehensive understanding of student engagement in online learning. The quantitative component provides generalizable data, while the qualitative component offers in-depth insights.
- Participants include 300 undergraduate students enrolled in online courses at three universities. A random sampling method was used to ensure a representative sample across different disciplines.
- Data collection involved an online survey with closed-ended questions on engagement, followed by semi-structured interviews with a subset of 20 students to explore their experiences more deeply.
- Quantitative data were analyzed using descriptive statistics and regression analysis in SPSS to assess relationships between variables. Qualitative data were coded and thematically analyzed using NVivo to identify key themes.
- Ethical approval was obtained from each institution’s review board. Participants were informed of the study’s purpose, and informed consent was obtained. All responses were anonymized to ensure confidentiality.
Writing Guide for Dissertation Methodology
Step 1: choose the research design and justify it.
Begin by identifying the research design that best suits your research question. Justify your choice based on how it aligns with the study’s objectives and the type of data needed.
Example : For a dissertation on the effects of diet on physical health, a longitudinal study might be chosen to observe changes over time, with justification based on the need to monitor long-term health outcomes.
Step 2: Describe the Sampling Process
Explain the target population, sampling method, and sample size. Justify your choices by explaining how they ensure the data will be representative and reliable.
Example : A study on employee motivation might choose a random sampling method to prevent bias, ensuring that findings can be generalized to a broader workforce.
Step 3: Outline Data Collection Methods
Provide a detailed description of how data was collected, including any instruments, techniques, and procedures. Explain why these methods are appropriate for capturing the data needed to answer the research question.
Example : If measuring customer satisfaction, an online survey may be selected due to its efficiency and reach, and its questions might be chosen to quantify specific satisfaction dimensions.
Step 4: Explain Data Analysis Procedures
Describe how you analyzed the data and justify the chosen techniques. Include information on any software used and specific statistical or thematic analysis methods applied.
Example : For a quantitative study, you might perform regression analysis to explore relationships between variables. In qualitative studies, you might use thematic coding to identify patterns.
Step 5: Address Ethical Considerations
Identify any ethical issues related to your study and describe how you addressed them. Ethical considerations are crucial for studies involving human subjects, as they help protect participant rights.
Example : For a study involving interviews, explain how participants were assured of confidentiality and provided with the option to withdraw at any time.
Tips for Writing an Effective Methodology
- Be Clear and Detailed : Provide enough detail so that another researcher could replicate the study based on your description.
- Use Justification for Every Choice : Explain why each methodological choice is suitable for your research objectives.
- Stay Objective and Neutral : Avoid using personal opinions or biases; focus on describing your research design and methods factually.
- Organize Logically : Follow a logical flow, usually beginning with research design, followed by sampling, data collection, data analysis, and ethical considerations.
- Keep It Concise but Comprehensive : Avoid unnecessary detail, but ensure you cover all relevant information needed to understand your methodology.
Common Pitfalls to Avoid
- Vagueness : Avoid vague language and provide specific details for each section.
- Lack of Justification : Justify all choices, from sampling methods to analytical techniques, to demonstrate thoughtfulness and rigor.
- Overcomplicating Language : Use clear, straightforward language rather than overly technical jargon to improve readability.
- Ignoring Limitations : Acknowledge any limitations in your methodology and discuss how they may impact the results.
The methodology section is a vital part of a dissertation, outlining the design, participants, data collection, data analysis, and ethical considerations. By following a structured approach, providing justifications for each choice, and ensuring clarity, you can write a robust methodology that supports the credibility and reliability of your research. A well-written methodology allows readers to evaluate the validity of your study and serves as a foundation for replicating or expanding upon your work.
- Creswell, J. W., & Creswell, J. D. (2018). Research Design: Qualitative, Quantitative, and Mixed Methods Approaches (5th ed.). SAGE Publications.
- Flick, U. (2018). An Introduction to Qualitative Research (6th ed.). SAGE Publications.
- Silverman, D. (2016). Qualitative Research (4th ed.). SAGE Publications.
- Yin, R. K. (2017). Case Study Research and Applications: Design and Methods (6th ed.). SAGE Publications.
- Saunders, M., Lewis, P., & Thornhill, A. (2016). Research Methods for Business Students (7th ed.). Pearson.
About the author
Muhammad Hassan
Researcher, Academic Writer, Web developer
You may also like

Table of Contents – Types, Formats, Examples

Thesis Format – Templates and Samples


Figures in Research Paper – Examples and Guide

Theoretical Framework – Types, Examples and...

Dissertation vs Thesis – Key Differences

Informed Consent in Research – Types, Templates...
ON YOUR 1ST ORDER
Mastering Dissertation Data Analysis: A Comprehensive Guide
By Laura Brown on 29th December 2023
To craft an effective dissertation data analysis chapter, you need to follow some simple steps:
- Start by planning the structure and objectives of the chapter.
- Clearly set the stage by providing a concise overview of your research design and methodology.
- Proceed to thorough data preparation, ensuring accuracy and organisation.
- Justify your methods and present the results using visual aids for clarity.
- Discuss the findings within the context of your research questions.
- Finally, review and edit your chapter to ensure coherence.
This approach will ensure a well-crafted and impactful analysis section.
Before delving into details on how you can come up with an engaging data analysis show in your dissertation, we first need to understand what it is and why it is required.
What Is Data Analysis In A Dissertation?
The data analysis chapter is a crucial section of a research dissertation that involves the examination, interpretation, and synthesis of collected data. In this chapter, researchers employ statistical techniques, qualitative methods, or a combination of both to make sense of the data gathered during the research process.
Why Is The Data Analysis Chapter So Important?
The primary objectives of the data analysis chapter are to identify patterns, trends, relationships, and insights within the data set. Researchers use various tools and software to conduct a thorough analysis, ensuring that the results are both accurate and relevant to the research questions or hypotheses. Ultimately, the findings derived from this chapter contribute to the overall conclusions of the dissertation, providing a basis for drawing meaningful and well-supported insights.
Steps Required To Craft Data Analysis Chapter To Perfection
Now that we have an idea of what a dissertation analysis chapter is and why it is necessary to put it in the dissertation, let’s move towards how we can create one that has a significant impact. Our guide will move around the bulleted points that have been discussed initially in the beginning. So, it’s time to begin.
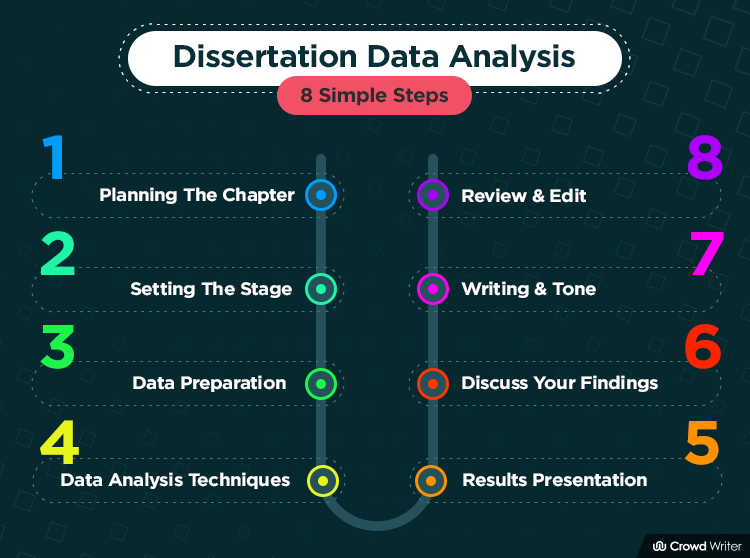
Step 1: Planning Your Data Analysis Chapter
Planning your data analysis chapter is a critical precursor to its successful execution.
- Begin by outlining the chapter structure to provide a roadmap for your analysis.
- Start with an introduction that succinctly introduces the purpose and significance of the data analysis in the context of your research.
- Following this, delineate the chapter into sections such as Data Preparation, where you detail the steps taken to organise and clean your data.
- Plan on to clearly define the Data Analysis Techniques employed, justifying their relevance to your research objectives.
- As you progress, plan for the Results Presentation, incorporating visual aids for clarity. Lastly, earmark a section for the Discussion of Findings, where you will interpret results within the broader context of your research questions.
This structured approach ensures a comprehensive and cohesive data analysis chapter, setting the stage for a compelling narrative that contributes significantly to your dissertation. You can always seek our dissertation data analysis help to plan your chapter.
Step 2: Setting The Stage – Introduction to Data Analysis
Your primary objective is to establish a solid foundation for the analytical journey. You need to skillfully link your data analysis to your research questions, elucidating the direct relevance and purpose of the upcoming analysis.
Simultaneously, define key concepts to provide clarity and ensure a shared understanding of the terms integral to your study. Following this, offer a concise overview of your data set characteristics, outlining its source, nature, and any noteworthy features.
This meticulous groundwork alongside our help with dissertation data analysis lays the base for a coherent and purposeful chapter, guiding readers seamlessly into the subsequent stages of your dissertation.
Step 3: Data Preparation
Now this is another pivotal phase in the data analysis process, ensuring the integrity and reliability of your findings. You should start with an insightful overview of the data cleaning and preprocessing procedures, highlighting the steps taken to refine and organise your dataset. Then, discuss any challenges encountered during the process and the strategies employed to address them.
Moving forward, delve into the specifics of data transformation procedures, elucidating any alterations made to the raw data for analysis. Clearly describe the methods employed for normalisation, scaling, or any other transformations deemed necessary. It will not only enhance the quality of your analysis but also foster transparency in your research methodology, reinforcing the robustness of your data-driven insights.
Step 4: Data Analysis Techniques
The data analysis section of a dissertation is akin to choosing the right tools for an artistic masterpiece. Carefully weigh the quantitative and qualitative approaches, ensuring a tailored fit for the nature of your data.
Quantitative Analysis
- Descriptive Statistics: Paint a vivid picture of your data through measures like mean, median, and mode. It’s like capturing the essence of your data’s personality.
- Inferential Statistics:Take a leap into the unknown, making educated guesses and inferences about your larger population based on a sample. It’s statistical magic in action.
Qualitative Analysis
- Thematic Analysis: Imagine your data as a novel, and thematic analysis as the tool to uncover its hidden chapters. Dissect the narrative, revealing recurring themes and patterns.
- Content Analysis: Scrutinise your data’s content like detectives, identifying key elements and meanings. It’s a deep dive into the substance of your qualitative data.
Providing Rationale for Chosen Methods
You should also articulate the why behind the chosen methods. It’s not just about numbers or themes; it’s about the story you want your data to tell. Through transparent rationale, you should ensure that your chosen techniques align seamlessly with your research goals, adding depth and credibility to the analysis.
Step 5: Presentation Of Your Results
You can simply break this process into two parts.
a. Creating Clear and Concise Visualisations
Effectively communicate your findings through meticulously crafted visualisations. Use tables that offer a structured presentation, summarising key data points for quick comprehension. Graphs, on the other hand, visually depict trends and patterns, enhancing overall clarity. Thoughtfully design these visual aids to align with the nature of your data, ensuring they serve as impactful tools for conveying information.
b. Interpreting and Explaining Results
Go beyond mere presentation by providing insightful interpretation by taking data analysis services for dissertation. Show the significance of your findings within the broader research context. Moreover, articulates the implications of observed patterns or relationships. By weaving a narrative around your results, you guide readers through the relevance and impact of your data analysis, enriching the overall understanding of your dissertation’s key contributions.
Step 6: Discussion of Findings
While discussing your findings and dissertation discussion chapter , it’s like putting together puzzle pieces to understand what your data is saying. You can always take dissertation data analysis help to explain what it all means, connecting back to why you started in the first place.
Be honest about any limitations or possible biases in your study; it’s like showing your cards to make your research more trustworthy. Comparing your results to what other smart people have found before you adds to the conversation, showing where your work fits in.
Looking ahead, you suggest ideas for what future researchers could explore, keeping the conversation going. So, it’s not just about what you found, but also about what comes next and how it all fits into the big picture of what we know.
Step 7: Writing Style and Tone
In order to perfectly come up with this chapter, follow the below points in your writing and adjust the tone accordingly,
- Use clear and concise language to ensure your audience easily understands complex concepts.
- Avoid unnecessary jargon in data analysis for thesis, and if specialised terms are necessary, provide brief explanations.
- Keep your writing style formal and objective, maintaining an academic tone throughout.
- Avoid overly casual language or slang, as the data analysis chapter is a serious academic document.
- Clearly define terms and concepts, providing specific details about your data preparation and analysis procedures.
- Use precise language to convey your ideas, minimising ambiguity.
- Follow a consistent formatting style for headings, subheadings, and citations to enhance readability.
- Ensure that tables, graphs, and visual aids are labelled and formatted uniformly for a polished presentation.
- Connect your analysis to the broader context of your research by explaining the relevance of your chosen methods and the importance of your findings.
- Offer a balance between detail and context, helping readers understand the significance of your data analysis within the larger study.
- Present enough detail to support your findings but avoid overwhelming readers with excessive information.
- Use a balance of text and visual aids to convey information efficiently.
- Maintain reader engagement by incorporating transitions between sections and effectively linking concepts.
- Use a mix of sentence structures to add variety and keep the writing engaging.
- Eliminate grammatical errors, typos, and inconsistencies through thorough proofreading.
- Consider seeking feedback from peers or mentors to ensure the clarity and coherence of your writing.
You can seek a data analysis dissertation example or sample from CrowdWriter to better understand how we write it while following the above-mentioned points.
Step 8: Reviewing and Editing
Reviewing and editing your data analysis chapter is crucial for ensuring its effectiveness and impact. By revising your work, you refine the clarity and coherence of your analysis, enhancing its overall quality.
Seeking feedback from peers, advisors or dissertation data analysis services provides valuable perspectives, helping identify blind spots and areas for improvement. Addressing common writing pitfalls, such as grammatical errors or unclear expressions, ensures your chapter is polished and professional.
Taking the time to review and edit not only strengthens the academic integrity of your work but also contributes to a final product that is clear, compelling, and ready for scholarly scrutiny.
Concluding On This Data Analysis Help
Be it master thesis data analysis, an undergraduate one or for PhD scholars, the steps remain almost the same as we have discussed in this guide. The primary focus is to be connected with your research questions and objectives while writing your data analysis chapter.
Do not lose your focus and choose the right analysis methods and design. Make sure to present your data through various visuals to better explain your data and engage the reader as well. At last, give it a detailed read and seek assistance from experts and your supervisor for further improvement.
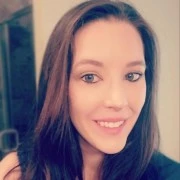
Laura Brown, a senior content writer who writes actionable blogs at Crowd Writer.
